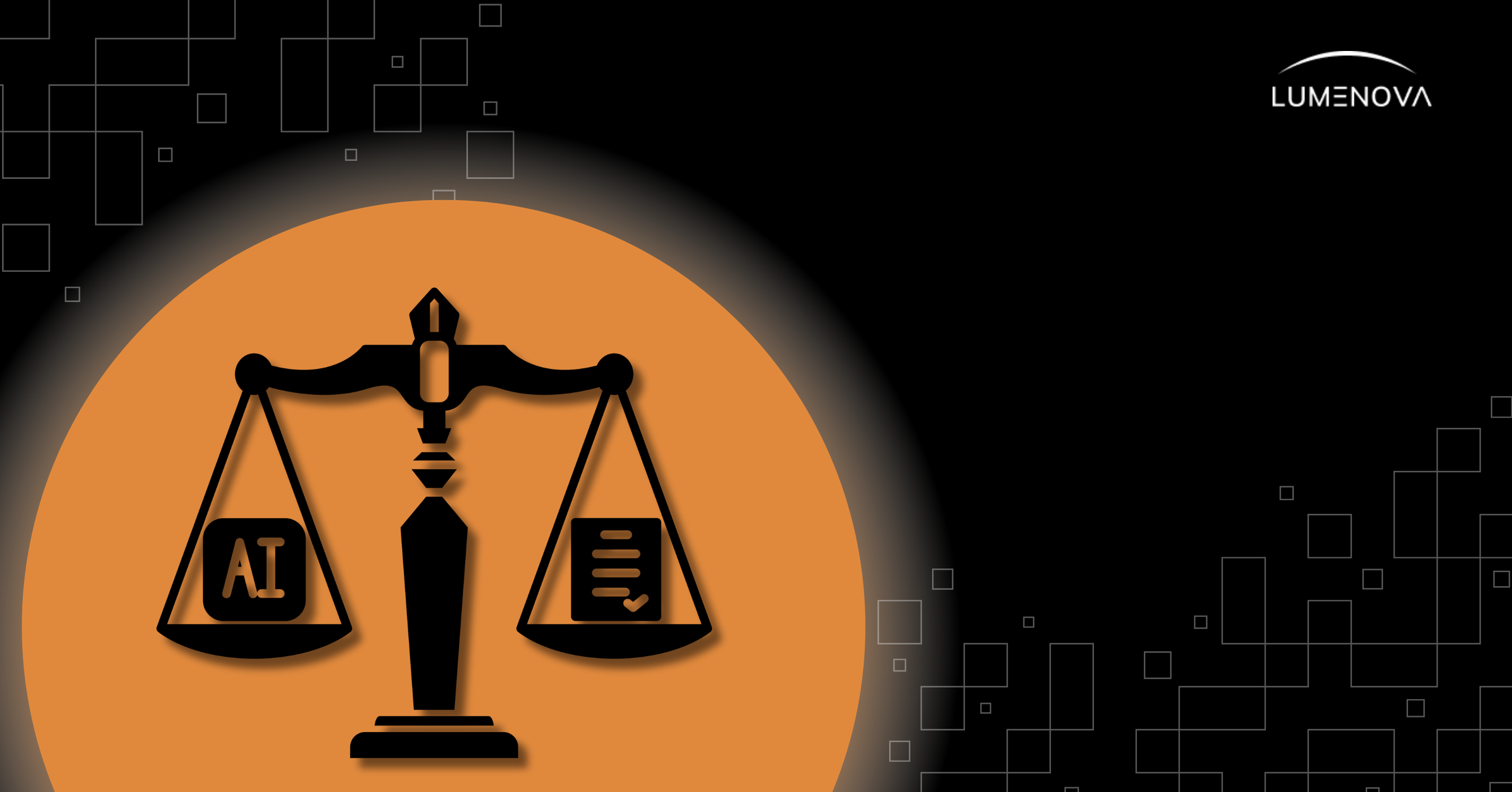
Contents
AI is no longer an experiment for the future. It’s embedded in the core infrastructure of modern finance. From credit underwriting to algorithmic trading, customer service to compliance, artificial intelligence in finance is powering the systems that drive decisions, manage risk, and shape customer experience.
If you’re leading a bank, investment firm, or fintech company, chances are AI is already part of your operations, even if it’s operating quietly in the background. It’s helping detect fraud in real time, assess creditworthiness, optimize portfolios, and deliver hyper-personalized client interactions. And it’s doing all of this continuously, with scale and speed that human teams simply can’t match.
But the same technologies that offer this competitive edge also introduce a new layer of complexity. Are your models making fair, explainable decisions? Are they secure from manipulation? And are they compliant with the fast-evolving regulations now targeting AI in financial services?
As pressure grows to accelerate innovation, the responsibility to govern AI wisely grows with it. Regulators are watching. Customers are asking tougher questions. And reputational risk has never moved faster.
This article looks at both sides of the equation: what AI is making possible in banking and investment and what it demands in return. The goal isn’t just to highlight the promise or the pitfalls, but to offer a clearer path to building AI that’s both powerful and trustworthy.
The Benefits: Smarter, Faster, More Personalized
In banking and investing, time and accuracy aren’t just nice to have; they’re critical. A delay in spotting fraud, a missed trend in the market, or a poor lending decision can cost millions and damage trust. That’s why so many financial institutions have turned to AI. It’s not just about cutting costs or replacing manual work: it’s about doing things better, faster, and at a scale that wasn’t possible before.
AI can process massive amounts of data in seconds, detect patterns humans might overlook, and help banks and investment firms make more informed decisions. It also allows companies to offer more tailored services, whether that’s adjusting a portfolio in real time or flagging a suspicious transaction before it becomes a problem.
At its best, AI doesn’t just improve operations; it adds real value by strengthening trust, improving performance, and making services more responsive to customer needs.
Here are some of the most impactful benefits AI offers to the finance industry:
1. Increased Efficiency and Speed
AI streamlines processes like loan approvals, trades, and portfolio management. For example, machine learning algorithms can analyze thousands of transactions in seconds, allowing firms to respond faster to market changes or client needs. This speed reduces manual errors and frees up teams to focus on strategy, ultimately boosting productivity and client satisfaction.
2. Enhanced Fraud Detection and Risk Management
One of the most powerful applications of AI in banking is fraud detection. Machine learning models can monitor huge volumes of transactions and instantly spot unusual behavior. If something looks off, such as a charge in another country or a pattern that doesn’t match a user’s habits, the system can flag it, block it, or notify a human to review.
This helps prevent losses, protects customers, and reinforces trust. As of April 2025, many firms report that AI has significantly lowered fraud-related losses and strengthened their risk models. According to BioCatch’s 2024 AI Financial Crime Survey, while 51% of financial institutions experienced losses between $5 million and $25 million from AI-driven fraud threats in 2023, the adoption of AI-based detection systems is helping many organizations close this gap. Firms that invested early in AI technologies for fraud prevention reported stronger resilience against evolving threats, with a growing emphasis on continuous monitoring, identity verification, and risk-based authentication as key components of their defense strategies.
3. Personalized Customer Experiences
AI enables banks and wealth managers to provide more personalized financial advice and investment strategies. By analyzing client behavior, preferences, and financial goals, AI can recommend relevant products or adjust portfolios automatically. It also powers tools like virtual advisors, which offer low-cost, tailored guidance. This level of personalization helps firms build stronger, more loyal relationships in a competitive market.
4. Cost Reduction
By automating repetitive tasks, AI helps cut operational costs. Chatbots handle routine inquiries, predictive analytics improves resource planning, and back-office automation speeds up paperwork-heavy processes. These savings can be reinvested into innovation or passed on to clients, creating a more efficient and competitive business model.
The Risks of AI in Finance
While the benefits are clear, AI also introduces new risks that financial firms must take seriously. These aren’t hypothetical or distant concerns, they’re already showing up in day-to-day operations, regulatory audits, and customer complaints.
The reality is, AI systems don’t run themselves. They require oversight, planning, and the right AI governance structures to avoid unintended consequences. Without that, what starts as a tool for efficiency can quickly become a source of legal, ethical, or financial exposure.
Some of the biggest challenges include:
1. Regulatory Compliance Challenges
Various global, US state and federal regulations like the EU AI Act, U.S. SEC proposals, and UK guidelines demand transparency, AI fairness, and human oversight in AI systems. If your AI tools make decisions about loans, investments, or risk, you’ll need to ensure they meet these standards, which can mean additional audits, documentation, and training. Non-compliance can lead to fines, reputational damage, and loss of customer trust, so staying ahead of regulatory changes is essential.
2. Security and Privacy Risks
AI systems handle sensitive financial data, making them prime targets for AI cyberattacks. Hackers can exploit vulnerabilities in AI models to steal information, manipulate outcomes, or disrupt operations. For example, where malicious inputs trick AI into making wrong decisions, it poses a growing threat to fraud detection and trading systems. Additionally, data privacy laws, such as those in the EU and U.S, require strict controls on how AI uses customer information, adding complexity to your security efforts.
3. Potential for Bias and Unfair Outcomes
Not all AI risks are technical. Some are moral. Should an AI decide who gets approved for a mortgage? Should a trading algorithm be allowed to act on subtle signals no human can fully understand? Financial institutions need to grapple with where automation stops and accountability begins, especially in areas that affect people’s livelihoods.
AI models learn from historical data, which can contain biases that lead to unfair decisions. In banking, this might mean denying loans to certain groups unfairly; in investments, it could skew portfolio recommendations. By April 2025, regulators and clients alike are demanding that AI systems be free from discrimination and that AI determinations made by AI are justifiable. If your company doesn’t address bias, you risk legal challenges, reputational harm, and eroding trust with stakeholders.
4. Operational and Systemic Risks
Over-reliance on AI can create vulnerabilities if systems fail or produce unexpected results. For instance, if an AI model used for market predictions malfunctions during a crisis, it could amplify losses or destabilize markets. The Basel Committee on Banking Supervision and other bodies are particularly concerned about systemic risks, where widespread AI failures could threaten financial stability. Managing these risks requires robust governance, regular testing, and contingency plans to ensure resilience.
Finding the Balance
These challenges don’t mean AI shouldn’t be used. They just mean it needs to be used responsibly. The right approach isn’t to slow innovation, it’s to guide it. That starts with putting strong systems in place to audit, monitor, and adjust AI tools as needed. It also means involving the right people, not just data scientists, but compliance officers, legal teams, and ethical advisors early in the development process.
Here are a few steps to consider:
-
Invest in governance and oversight
Put frameworks in place to monitor AI performance, flag issues, and ensure compliance. Maintain human oversight for key decisions and train teams to work with AI systems responsibly. -
Strengthen cybersecurity
Conduct regular security audits. Protect your data pipelines. And be transparent with clients about how their information is used and protected. -
Test for bias and fix it
Run AI bias checks. Involve diverse voices in model development. And make fairness a key part of your AI design process, not an afterthought. -
Stay ahead of regulation
Laws are changing fast. Assign people or teams to track updates and translate legal requirements into practical policies. Partnering with legal or compliance experts can help make this manageable.
Bottom line?
AI is helping finance evolve, but it’s also changing the risks. With smart strategies, strong governance, and an eye on ethics, banks and investment firms can unlock the full value of AI without losing control.
Want more insights on building responsible AI in finance? Explore expert resources on the Lumenova AI blog, where we dive deeper into AI governance, regulatory trends, and practical tools to help you stay ahead. Book a demo to see how Lumenova can support your AI initiatives, and follow us on X and LinkedIn for the latest updates and thought leadership.