April 29, 2025
Does Your AI Monitoring System Follow These Best Practices?
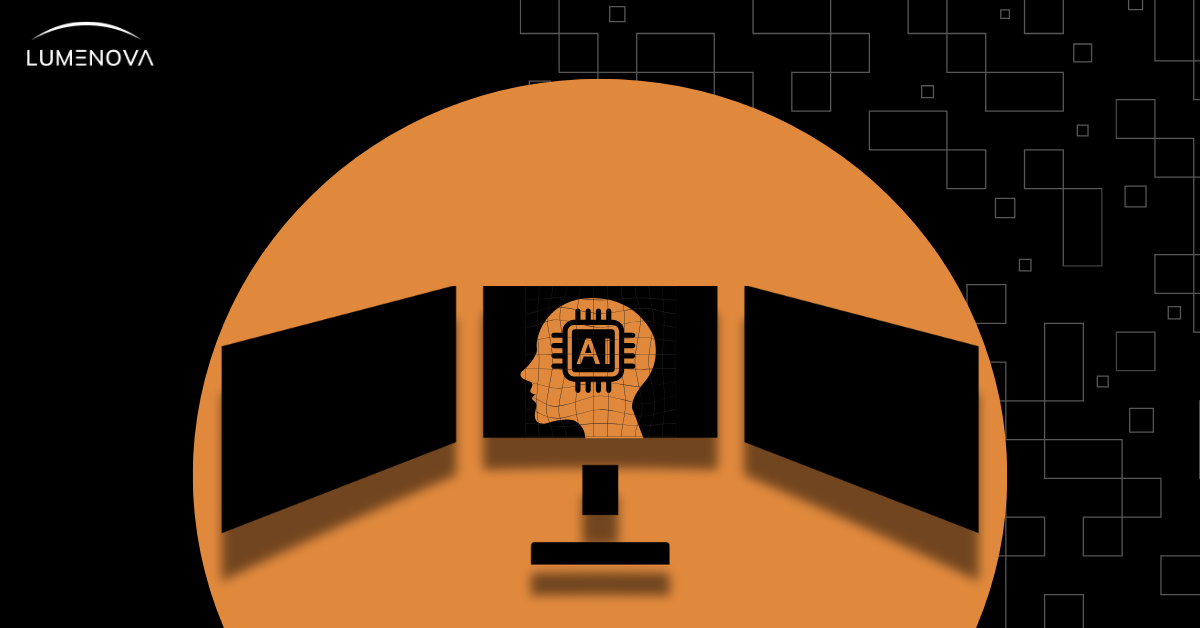
Contents
A while ago, everything seemed fine. The model was working. The dashboard looked good. The numbers were where they were supposed to be. But then something shifted. Maybe it was subtle at first (a few strange predictions, a bump in error rates, or customer support raising an eyebrow). By the time someone noticed, it was already a mess. The AI had drifted, and no one caught it in time.
This kind of moment isn’t rare. It’s becoming more common. And it usually happens in the gap between deploying an AI system and keeping it healthy.
The remedy extends beyond supplementary dashboards or mere notifications. A robust AI monitoring system is imperative, characterized by efficacy (its ability to detect issues accurately and in real time), scalability in alignment with model evolution, adaptability to data variations, and the facilitation of operational resilience.
Here’s what that looks like in practice.
Why AI Monitoring Is a Must-Have
When AI first gets deployed, there’s excitement. You’ve tested the model, cleaned the data, and tuned the performance. But deployment is just the beginning.
After launch, models face the real world. Data changes. Users behave differently. New AI risks show up. And if no one’s watching closely, things can go sideways.
AI monitoring helps you:
- Catch issues early (before they turn into real damage)
- Keep your model performing the way it should
- Stay compliant with laws and internal policies
- Build trust with users, auditors, and regulators
Example: Apple’s AI-powered news summary tool, “Apple Intelligence,” delivered notifications with false information, including claims about high-profile events that were never reported by the referenced sources.
For example, it falsely stated that a darts player had won a championship before the final match occurred. Apple had to disable the feature temporarily and issue updates to clarify when notifications are AI-generated. This problem underscores the need for robust monitoring to prevent misinformation and maintain user trust.
AI Monitoring Best Practices
If you’re implementing an AI monitoring system, these are the best practices you should be following. These best practices are drawn from real-world challenges we’ve seen across teams and industries (mistakes that often surface only after the damage is done).
1. Continuous Monitoring (Don’t Just Check Once)
Things change fast after deployment. Monitoring periodically isn’t enough. AI systems need continuous, real-time checks. That means:
- Watching inputs, outputs, and system behavior in real-time
- Setting performance thresholds and alerts for potential issues like model drift
- Logging what the model is doing, not just what results it gives
For additional insights on this subject, please consult our guide: How to Make Sure Your AI Monitoring Solution Isn’t Holding You Back. This resource will enable the identification of inefficiencies and the optimization of your strategic approach.
2. Define the Right Performance Metrics
Accuracy alone isn’t enough to understand how well your model is performing in production. It’s a surface-level metric that can hide deeper problems, especially in complex or high-risk use cases.
What you measure should reflect how the model is actually used, what’s at stake if it goes wrong, and what outcomes you care about.
That might mean tracking how confident the model is in its predictions, how fast it responds, how it impacts user behavior or business KPIs (or something more task-specific), depending on the model type. The key is flexibility. Your monitoring should adapt to the problem you’re solving, not just the type of model you’re using.
Review these often. As your business changes, your metrics might need to change too.
3. Watch for Anomalies (Before They Escalate)
Sometimes the model doesn’t fail obviously. It just starts making odd choices. You need systems that can detect:
- Data distribution shifts
- Emergent behaviors or objectives
- Adversarial vulnerabilities
- Outlier outputs
- Changes in user behavior or operational conditions
- Spikes in low-confidence predictions
Anomaly detection tools help you catch performance degradation early, when it’s still manageable.
4. Keep an Eye on Model Drift
Drift is what happens when the model starts to lose touch with reality. The world changes, but the model doesn’t. For example:
- Data drift: The inputs are changing
- Concept drift: The relationships between inputs and outputs have changed
Your monitoring tools should help detect model drift, so you can retrain when needed.
For a full perspective on model drift (from early detection to long-term mitigation):
↳ Read Part I: Model Drift: Types, Causes and Early Detection
↳ Continue to Part II: Model Drift: Detecting, Preventing and Managing Model Drift
5. Don’t Ignore Data Quality
If your model ingests bad data, it will produce bad results. To proactively counteract data quality issues Make sure to:
- Check for missing, corrupted, or inconsistent input data
- Validate data formats and sources
- Track which features are most important to model output
Poor data can make a great model look broken.
6. Use Explainability Tools
Not every stakeholder wants to hear about log-loss. But they all want to know why the model made a certain decision. Build in XAI tools that:
- Show feature importance in plain language
- Help non-technical users understand outcomes
- Support audits, reviews, and incident analysis
7. Feedback Loops (Your Secret Weapon)
Your best insights often come from the people using the model every day. Build systems that:
- Let users flag anomalous or incorrect results
- Capture corrections or labels from downstream teams
- Feed that data back into retraining or improvement cycles
The people closest to the model can see what metrics are sometimes missed.
8. Security and Privacy Monitoring
An AI system is an asset, which makes it a target. If it’s tied to customer data or financial decisions, even more so. You need to:
- Monitor for unauthorized access or tampering
- Track changes to models or data pipelines
- Ensure encryption and access controls are in place
Compliance with GDPR, HIPAA, or other standards should be baked into your monitoring design.
9. Regular Audits and Reviews
You need formal checkpoints, not just alerts. Build a schedule for:
- Internal model audits
- External reviews or third-party validations
- Documentation of changes, incidents, and performance over time
Audits are not just about defense. They’re how you learn what’s working and what’s not.
10. Plan for Incidents Before They Happen
Most systems fail eventually. What matters is how fast you recover. Create a plan that includes:
- Defined incident types (performance drop, security breach, etc.)
- Response teams and roles
- Communication protocols (internal and external)
- Post-incident review processes
Having a plan means you don’t have to panic later.
11. Document Everything
Monitoring isn’t just about doing. It’s about showing your work. Keep clear, up-to-date documentation of:
- What you’re monitoring and why
- Your performance baselines and thresholds
- Changes to models, data, and systems
- Any incidents or escalations
Documentation helps teams stay aligned and proves to stakeholders that your AI is under control.
How Lumenova AI Supports These Best Practices
Our RAI platform is built to make AI monitoring work at scale. Here’s how we help:
- Continuous model tracking: Stay on top of how your model’s performance metrics evolve over time.
- Anomaly detection: Spot anomalous patterns before they cause trouble
- Model drift detection: Automatic alerts when your model starts to drift
- Explainability built-in: Clear, understandable insights for any audience
- Integrated feedback: Connects user feedback right into your review loop
- Audit-ready reports: Generate logs and summaries for any time period
- Access control and compliance checks: Role-based permissions and privacy controls from day one
- Incident management tools: Set workflows, assign tasks, and follow through
Conclusion
AI systems are powerful. But they don’t run on autopilot forever. If you’re still using ad hoc scripts or hoping someone notices when things break, it’s time for a change. These best practices give you a checklist. A direction. A way to move from reactive to proactive.
When it comes to AI, the sooner you catch a problem, the easier it is to solve.
Want to see how this works in real life? Book a demo with Lumenova AI. We’ll walk you through how intelligent monitoring keeps your models sharp, your teams ready, and your business protected.