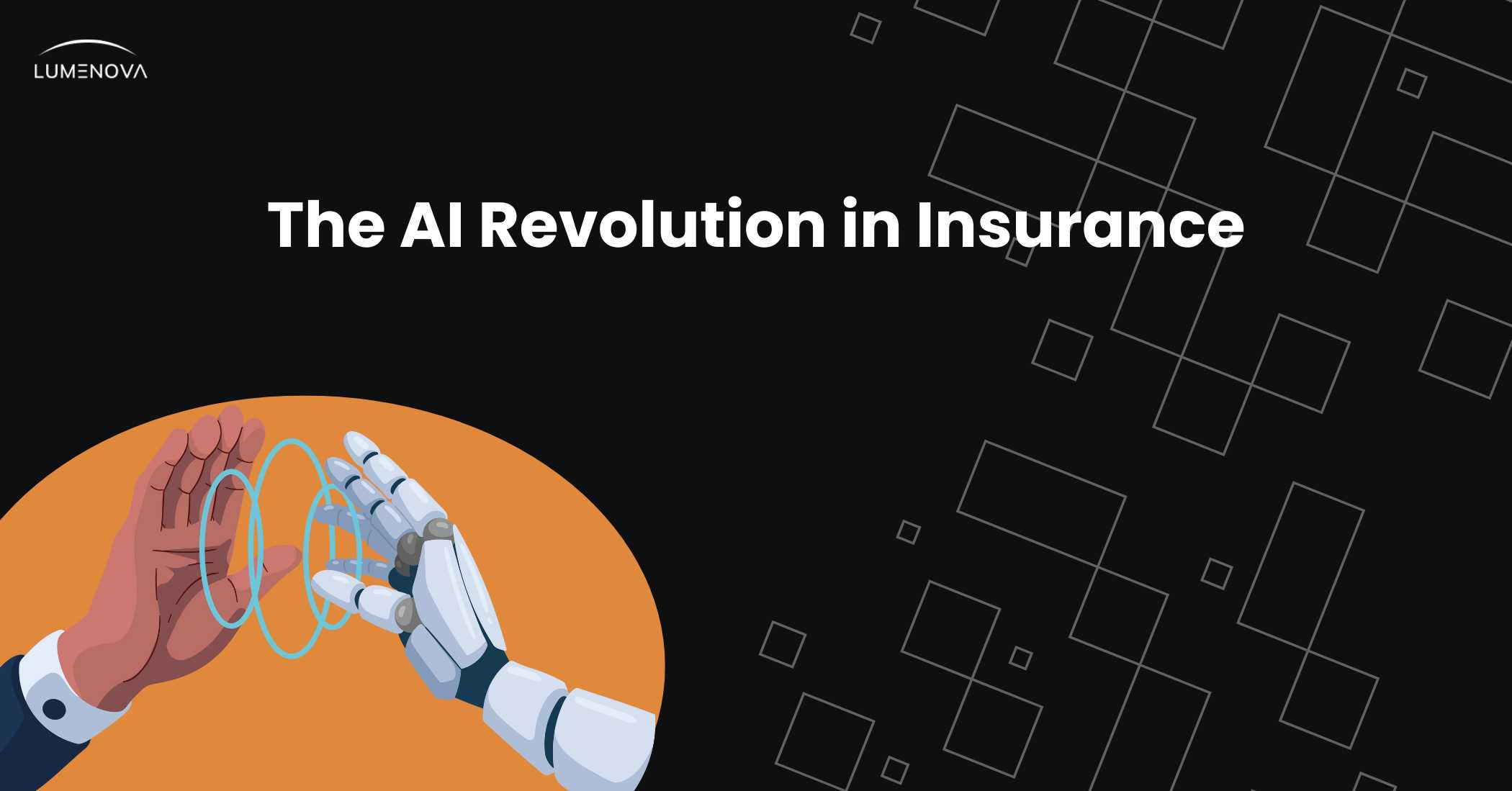
As AI started to emerge in the insurance sector, it represented a transformative leap forward, harnessing the power of data analysis and automated processes to enhance the customer experience, streamline operations, and redefine the traditional insurance landscape.
AI’s introduction into this space has brought about efficiency of an unprecedented scale. By employing algorithms and machine learning, insurance firms are now able to process claims at an accelerated pace, reducing the time it takes for policyholders to receive payouts. For instance, AI can quickly assess damage using images, determine appropriate compensation, and even predict fraudulent claims with a level of precision that human adjusters would struggle to match.
Beyond claims, AI is personalizing insurance offerings. By evaluating a wealth of user data, algorithms can tailor policies to individual needs and risk profiles. Customers could benefit from more accurate premium calculations and policy recommendations that align more closely with their lifestyles, a far cry from the one-size-fits-all policies of the bygone era.
The age of chatbots in insurance is already here
As one of the leading insurers in the United States, Illinois-based State Farm leverages artificial intelligence (AI) to enhance its service delivery and operational efficiency. The use of AI by State Farm manifests in several applications, including virtual assistants for customer service, claim handling, fraud detection, and personalization of insurance policies.
AI-driven chatbots assist customers with inquiries and claims processing, offering a streamlined and responsive service experience, while their machine learning (ML) algorithms are employed to analyze claims data, which helps in the quick and accurate settlement of claims, as well as identifying potential fraud. By embracing AI technologies, State Farm is better positioned to meet customer demands and adapt to the evolving landscape of the insurance industry.
They’ve managed to:
-
Streamline customer communications by using their AI-driven CRM and financial services platform to enable their agents to source details about customers much faster and enable personalized communications across channels, thus not only improving customer satisfaction levels and retention, but also productivity.
-
Expedite processing and analysis for contracts by using natural language processing (NLP), computer vision (CV) and machine learning to reduce contract analysis processing time, which would boost employee productivity and reduce costs.
-
Combat insurance fraud by detecting anomalies and using predictive modeling to anticipate and counteract new threats in the insurance industry.
Insurance companies like State Farm are increasingly using AI for its ability to analyze vast amounts of data quickly, accurately, and cost-effectively. AI enhances risk assessment, claims processing, customer service, and fraud detection. As the technology advances, AI systems will be more adept at predicting individual customer needs, offering personalized policies, and preventing losses. This leads to greater efficiency, improved customer satisfaction, and competitive edge in the market. The scalability and evolving capabilities of AI make it a key driver of innovation and strategic advantage in the insurance industry.
So… what could go wrong with AI in insurance?
Despite the transformative benefits AI brings to the insurance sector, its infusion also raises several potential concerns. According to KPMG, ethical issues around AI decision-making and the absence of robust regulation are seen as highly challenging by 52% of CEOs. As AI continues to go deeper into this industry, it’s necessary to consider what could go wrong and the implications of these issues.
When it comes to the responsible adoption of AI, embracing the right platforms can help insurers adapt to any market changes and welcome innovation to drive insurance toward a more inclusive, customer-centric, data-driven future.
Data Security and Privacy
AI systems in insurance operate on copious amounts of personal data, including sensitive information such as medical records, financial history, and personal identification details. In the case of a breach:
- Hackers could access or corrupt these vast data stores, leading to significant privacy violations.
- Stolen data could be used for nefarious purposes, such as identity theft or financial fraud, inflicting immense damage on individuals.
- Insurance companies could face costly legal battles, severe fines, and lasting reputational harm.
Bias and Discrimination
AI algorithms learn from historical data, which may reflect existing prejudices:
- Biased AI can lead to unfair policy pricing, where certain groups are inadvertently discriminated against.
- Pervasive biases could erode public trust and lead to systemic inequality.
- Regulatory backlash could emerge, forcing insurers to overhaul their AI systems or face sanctions.
Over-Reliance on Automation
Excessive reliance on AI can diminish the human expertise within the insurance industry:
- Job displacement could negatively impact the labor market, as AI streamlines processes traditionally handled by employees.
- Over-automation could reduce the quality of customer service, with impersonal interactions and a lack of empathy for customer situations.
- AI may make errors that humans could have caught, such as misinterpreting unique or complex claims.
Transparency and Accountability
AI decisions are often inscrutable, presenting challenges in transparency:
- Customers may not understand how or why certain decisions are made, which can be problematic when disputing claims or handling grievances.
- Without clear accountability, it becomes difficult to distinguish whether errors stemmed from AI or human oversight.
- Regulators may require explanations for AI decisions, and companies that fail to provide this clarity could be penalized.
Systemic Risks and Failures
AI systems, though designed to be robust, can fail:
- A malfunctioning AI could lead to widespread errors in claims processing, affecting thousands of policies at once.
- AI-driven investment algorithms could misread market signals, leading to significant financial losses.
- The homogenization of risk assessment could amplify systemic market risks, as many insurers might rely on similar AI models.
What to do to prevent your AI system from going wrong
After touching on ways an AI system could fail in Insurance, it’s best to know that cultivating a robust AI-driven environment can save you from dealing with a wide variety of negative factors along the way, and can be achieved through various strategies.
To ensure the robustness of their AI systems, companies must focus on several key areas, including the design, data, and ongoing monitoring. When developing and deploying AI systems, it is crucial to take a proactive approach to prevent the possibility of malfunctions or unintended consequences.
As a first step, it’s essential to begin with a strong foundation in design. AI systems should be built with transparency in mind, making sure that each and every decision made by the system can be understood and explained. This is often referred to as explainable AI (XAI), which identifies faults or biases in the system’s decision-making process.So, it’s especially important to rely on a multidisciplinary team that includes ethicists, engineers, and domain experts to receive different perspectives that could address potential issues.
Secondly, the quality and variety of data used to train AI systems play a significant role, which stresses the importance of having comprehensive and representative datasets to avoid embedding biases into the AI. This involves not only the careful selection of data sources but also the diligent preprocessing of data, which helps in identifying and mitigating any biases or anomalies.
AI audits and data solutions are critical in ensuring the integrity, fairness, and transparency of AI systems. By ensuring regular audits, organizations can easily identify biases, errors, and security vulnerabilities, therefore ensuring that their AI applications are trusted. Audits are also essential for regulatory compliance, risk management, and maintaining data privacy. As AI systems influence decision-making in insurance, these audits become vital for mitigating potential harm and reinforcing ethical standards.
To ensure data privacy, especially in the insurance sector, where client information’s confidentiality is paramount, organizations need to use techniques that intelligently identify and mitigate potential security breaches before they occur. This dual strategy of data encryption coupled with AI-specific security not only fortifies the insurers' defense against cyber threats, but also reinforces the trust placed in them by their clients, creating a robust framework that protects client privacy.
Equally important is establishing strict ethical guidelines by setting clear boundaries for what the AI system should and should not do, based on societal norms and legal considerations. This means that embedding ethical considerations into the AI development process helps prevent misuse and harm.
When it comes to testing AI systems, they should go through rigorous and robust testing before deployment to catch many potential issues. By ensuring a continuous integration and secure deployment practices to allow for regular updates and patches to the AI system, any emerging issues could be rectified in a short amount of time.
Finally, the most important aspect after deployment is that AI should not be set and forgotten, and this is where the human touch (oversight) is necessary to continuously monitor and detect any abnormal behavior. The implementation of feedback loops — where the system’s performance and decisions can be assessed and refined — could ensure quick corrective actions from a human operator.
In summary, preventing AI systems from going wrong requires careful attention to system design, data integrity, ethical guidelines, thorough testing, and ongoing monitoring. By addressing each of these areas with due diligence, one can mitigate risks and contribute to the development of reliable, trustworthy AI solutions.
Lead your organization to Responsible AI with Lumenova AI
To conclude, the AI revolution in insurance is not without its pitfalls, yet it is undeniably pushing the industry toward a more user-friendly, efficient, and customized future. With appropriate measures to address ethical and security concerns, AI can lead to a significant net positive for both insurers and consumers. The key is in finding the right balance between leveraging cutting-edge technologies and upholding the values that earn customer loyalty.
While AI in insurance heralds efficiency and innovation, its potential for data breaches, bias, loss of human interaction, opacity, and systemic flaws should be thoroughly addressed. Organizations must implement robust safeguards, maintain balance with human judgment, and ensure AI systems are transparent and free from prejudice. This forward-thinking approach will safeguard against AI’s pitfalls while capitalizing on its ability to revolutionize the industry.
Lumenova’s Responsible AI (RAI) platform provides a holistic strategy to address AI related risks with precision. Designed to elevate the fairness, transparency, and responsibility of your AI systems, our platform makes sure you’re up to par when it comes to ethical standards and the promotion of fairness in decision-making processes. We put a very strong emphasis on these issues, which means our RAI platform can empower your organization to cultivate an inclusive and responsible AI environment.
Discover how Lumenova AI’s RAI platform can help your organization navigate the complexities of AI deployment by requesting a demo, or contact our AI experts for more details.