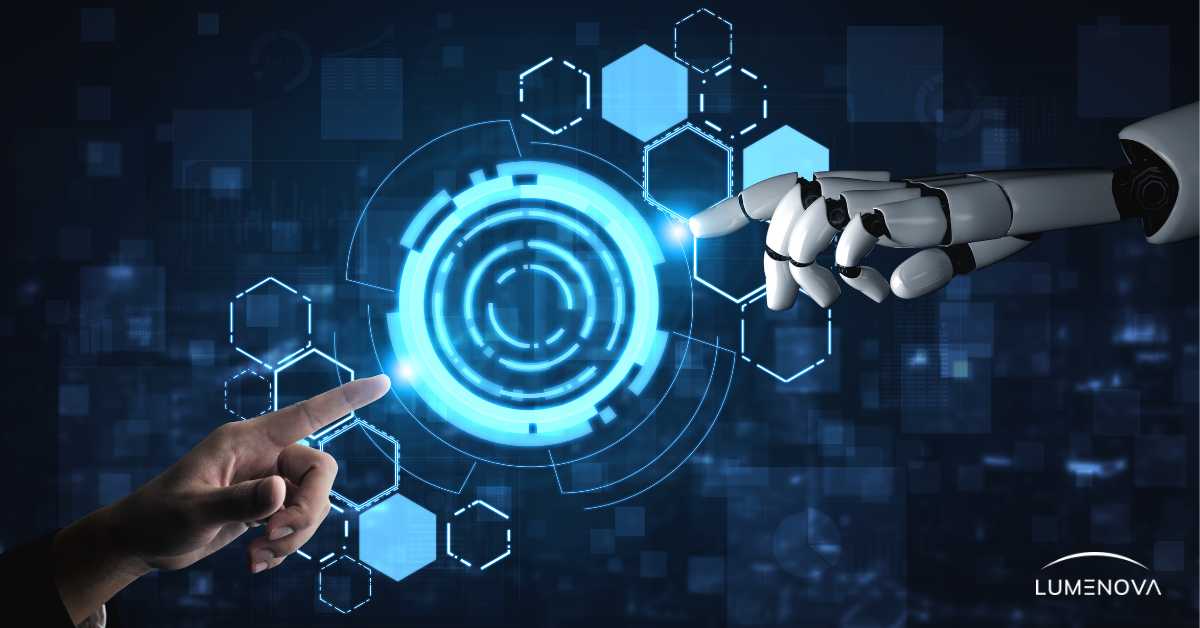
Understanding how a machine learning algorithm reaches the predictions it does is important knowledge, required even, in the case of highly-regulated industries like Healthcare and Finance.
Transparent AI can help mitigate issues relating to bias, discrimination, and performance. From both an ethical and legal standpoint, AI transparency is becoming an important advantage for those who have it and a major roadblock for businesses that don’t.
As previously discussed on our blog, the subfield of AI focusing on enhancing model transparency is called Explainable AI or XAI.
Transparency and explainability aim to bridge the gap between machines and humans, by creating a shared understanding between them, and helping the latter make sense of the ‘why’ behind a particular decision.
Working toward AI transparency
As opposed to an opaque and potentially flawed model that intimidates users, or unknowingly perpetuates bias, a transparent AI works toward building trust, by allowing them to understand the reasoning behind the predictive process.
Transparent AI makes room for two distinct forms of model interpretability, which promote a multifaceted view of how the system behaves in different situations.
💡 Global explainability enables the understanding of how various features contribute to the predictive process across the entire dataset. It is especially useful for developers and business operation representatives because it improves debugging and facilitates adherence to regulatory compliance.
💡 Local explainability indicates which attributes contribute most to an individual prediction. Local explanations would be more important to end-users, allowing them to understand which criteria weigh more in their specific case. For instance, a person would understand why their loan application got rejected and what they would need to change in order to get it approved.
The benefits of transparent AI
Increased adoption
A closed environment leaves room for questions and doubts. As such, it is no wonder that people feel anxious and wary about embracing AI-based solutions.
This standpoint is very evident in the financial sector, where 32% of finance executives cited the lack of interpretability of black-box machine learning algorithms as a major blockage in the path of adoption, as per the 2021 LendIt annual survey.
Often enough, AI transparency is sacrificed on behalf of accuracy. However, in the grand scheme of things, without a layer of explainability, adoption will be slower both in the case of employees and consumers.
Compliance
Another benefit of AI transparency lies in the facilitation of legal and regulatory compliance. Since machine learning algorithms must be developed responsibly and in accord with legal requirements, transparent AI is essential to highly-regulated sectors.
The Algorithmic Accountability Act of 2022 is the most recent example of legal oversight, showcasing the increasing demand for transparency in automation.
The list of potential AI applications is ever-growing and Machine Learning algorithms will continue to shape industries worldwide. However, the more impactful AI becomes, the more important it will be for machine learning algorithms to abide by ethical regulations and be compliant from a legal perspective.
Optimization
The use case of AI transparency goes even further, by enabling efficient process optimization and allowing data scientists and developers to ensure that their machine learning algorithms are performing as planned.
Through an insightful real-life example, machine learning engineer Ayla Kangur highlights the importance of AI transparency for model optimization. She describes how one of the models she worked on at some point in her career was able to sort and categorize scientific documents with high accuracy. It turned out, however, that the model’s performance was not quite on point. Instead of basing its predictions on relevant information, the machine learning algorithm used a leftover HTML tag as a reference.
In this case, the need for AI transparency, which initially arose as a requirement of the pharmaceutical industry, turned useful in more ways than simply ensuring compliance. It helped the team understand that the model was, in fact, flawed.
As such, it’s safe to say that AI transparency enables growth.
Key takeaways
While Machine Learning algorithms are often reputed to be black boxes, prone to bias and unfairness, they don’t have to be. With the right tools, businesses can make their AI models explainable and transparent.
By means of Responsible AI, business leaders, data scientists and developers alike can gain all the insights needed to minimize risks, reduce systematic bias, and comply with current and upcoming regulations.
Lumenova AI can help make your black box AI transparent, so your company can:
- Meet the increasing legal and regulatory requirements for AI use
- Ensure a fair and bias-free decision-making process
- Enhance model performance, reliability and robustness
To request a demo, please get in touch with our team of our experts today.