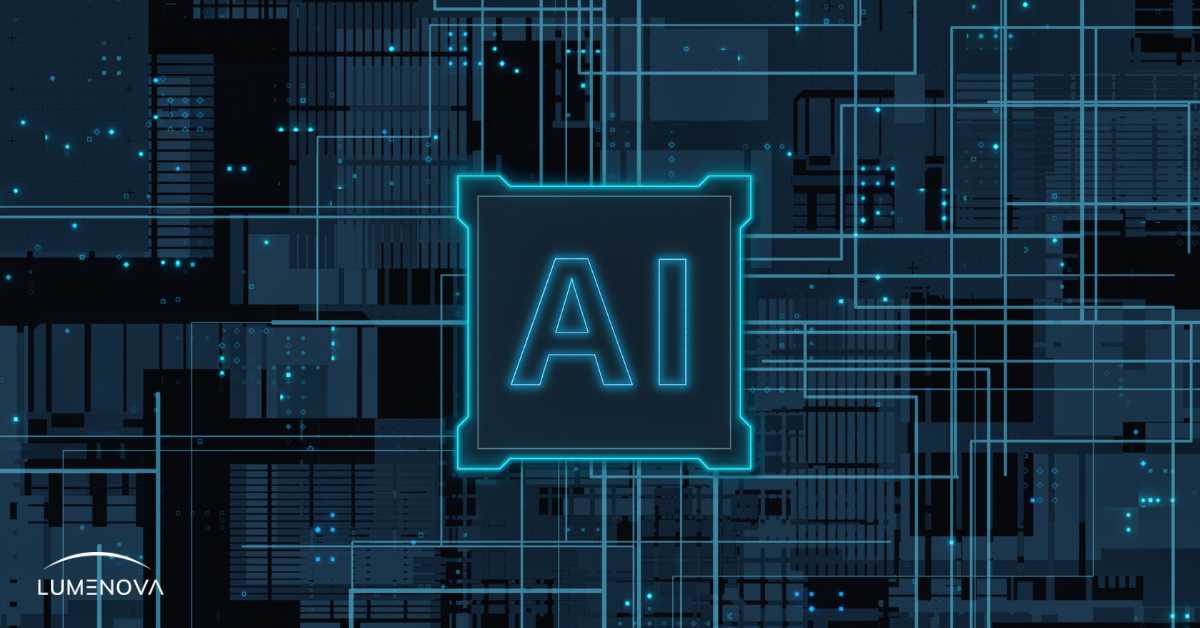
Organizations and governments worldwide have begun to embrace prediction-based decision algorithms. The areas of interest span from credit applications and lending to online advertising and criminal pre-trial proceedings.
AI can help organizations and businesses to:
- Build and benefit from intelligent processes
- Gain a competitive edge through real-time optimization
- Increase revenue by analyzing consumer behavior
- Minimize risks when it comes to offering loans or parole
Of course, the list above is far from exhaustive.
Still, one challenge remains: achieving AI fairness.
In one of our previous articles we discussed the source of AI bias, and how data collection and feedback can sometimes negatively influence an ML model’s prediction. Depending on the circumstances, such outputs can lead to harmful impacts on human lives.
Consequently, bias and unfairness in machine learning models also raise ethical concerns regarding the use of AI in real-life situations.
Let’s take a look at one of the most notorious cases where the biased historical data on which the ML model was trained ultimately led to unfair or biased predictions.
COMPAS
The COMPAS (Correctional Offender Management Profiling for Alternative Sanctions) prediction system developed by the firm Equivant (formerly known as Northpointe) is used in courtrooms across the US to forecast which criminals are most likely to commit another crime.
COMPAS issues risk scores for recidivism based on factors such as previous arrests, employment, and age. Judges use these scores to decide if offenders will face prison or jail time.
In 2016, ProPublica reported that the AI was biased against black people. While the algorithm correctly predicted recidivism for black and white defendants at approximately the same rate, black arrestees were incorrectly labeled as being ‘high-risk’ twice as often as their white counterparts. The ML model also scored white people who were more likely to commit a crime as lower.
Northpointe rejected this accusation, arguing against the conclusion of racial AI bias in COMPAS’s predictions. According to them, the algorithm remains fair since it reflects the same likelihood of recidivism across all groups.
This type of reasoning goes against other definitions of machine learning fairness, especially since race is a protected social group category.
Other noteworthy cases of AI bias
Unfortunately, the bias behind COMPAS does not reflect a one-off happening.
There are other several noteworthy examples of giant industry names employing models that turned out to be biased at a closer inspection. For example:
- Amazon’s sexist recruitment tool
- Google’s racist object detection software
- Apple’s sexist credit card
- Meta’s antisemitic chatbot
- LinkedIn’s sexist search algorithm
Is it surprising then, that people’s faith in AI is falling? After all, many machine learning models are black boxes.
Because of this uncertainty, as well as other concerns relating to AI fairness and discrimination, academics, practitioners, and consumers alike have been calling for more transparency regarding the inner workings of ML models.
Mitigating AI bias
While we expect machine learning models to be fair and non-discriminatory, the truth is, things are not that simple. From dataset development through to algorithm development, our different interpretations and understanding of AI fairness prove it difficult to identify and apply a unique solution for de-biasing ML models.
Furthermore, challenges arise from a technical perspective as well, since AI fairness constraints lead to lower accuracy in terms of predictions.
Steps to Mitigate AI Bias
While there are a number of available ‘de-biasing’ techniques, it still remains difficult to measure AI fairness mathematically. There are several gateways through which bias can enter an AI system, taking the form of:
- Incorrect data collection
- Differential treatment
- Biased feedback
This being said, AI fairness can be addressed at several stages of the modeling workflow. These techniques involve:
- Pre-processing: Applying mitigation methods to the dataset before training the algorithm.
- In-processing: Incorporating mitigation techniques into the model training process.
- Post-processing: Using bias correction methods on predictions in order to achieve the desired level of AI fairness.
Qualitative and quantitative tools can help machine learning professionals to better understand the concept of fairness and calibrate AI systems accordingly. They are created to assist engineers and data scientists in their efforts to examine, report, and mitigate bias in ML models.
Such tools include:
- IBM’s AI Fairness 360 Toolkit
- Google’s What-If Tool
- Microsoft’s fairlean.py
- Microsoft’s Co-designed AI fairness checklist
Key takeaways
Recognizing and reducing AI unfairness and bias are indeed difficult tasks, but necessary in order to prevent outcomes that might have a harmful impact on people’s lives and build trust in the use of AI.
As ML models become increasingly complex and obtaining transparency increasingly challenging, the need to incorporate elements of explainability is now perhaps more pressing than ever.
At Lumenova AI, we employ a unique way of measuring AI fairness at a glance, by analyzing metrics such as data impartiality, demographic parity, equality of opportunity, equality of odds, and predictive parity.
Moreover, our framework allows you to go even further by analyzing intersectional fairness, and determining how different groups of protected attributes may be discriminated against.
To learn more about our tool and how it can make your ML model fair, feel free to request a demo.