August 8, 2024
Existential and Systemic AI Risks: A Brief Introduction
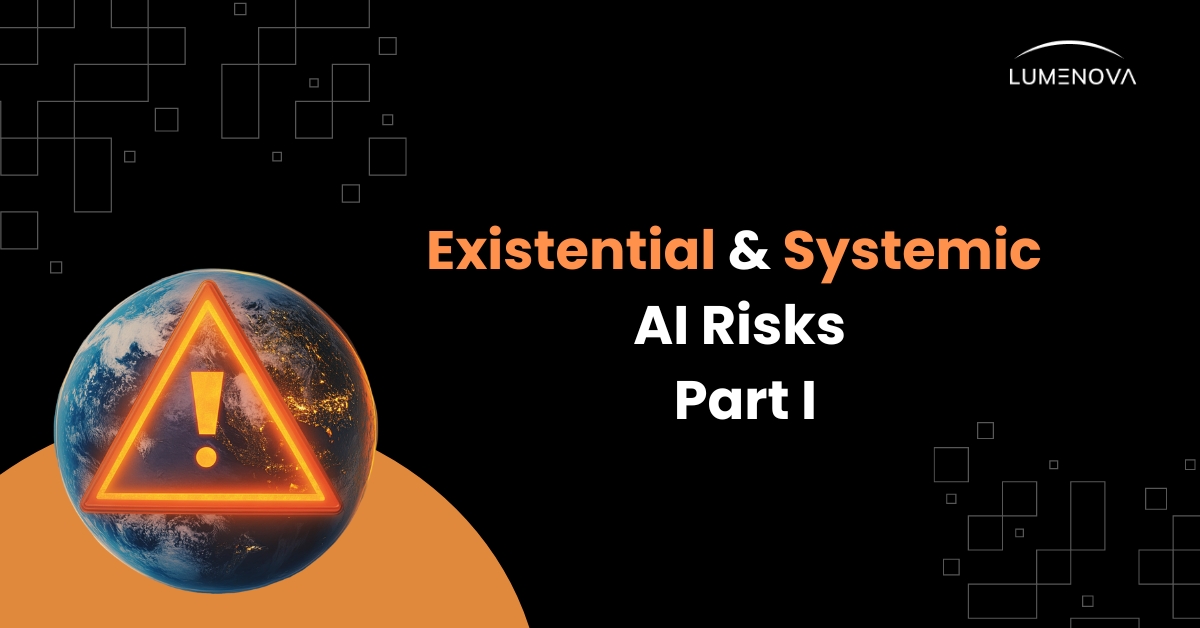
Contents
Existential and systemic AI risks, despite not being highly prioritized by most existing risk management frameworks and regulations, are crucial to mitigate due to the magnitude, severity, intensity, and timeframe of their consequences, which could drive potentially catastrophic events for society, democracy, and humanity at large.
These kinds of risks, however, tend to be much harder to quantify and understand concretely—as opposed to conventional AI risks like algorithmic discrimination or IP rights infringement—seeing as they’re either fueled by speculative assumptions like the emergence of artificial general intelligence (AGI) or superintelligence (ASI), or alternatively, operate over prolonged timeframes where a conglomeration of complex factors manifesting at all levels of society dictates whether they materialize.
Realistically, most private sector organizations will adopt a predominantly pragmatic and narrow approach to AI risk management, whereby risks specific to the organization are targeted and addressed—most businesses simply won’t have the time, resources, knowledge, or interest to fully grasp how their AI actions may factor into systemic and existential risk scenarios unless they’re required to do so under existing regulations or industry standards. This isn’t a slight on existing organizations, but rather an acknowledgment of reality.
Fortunately, public sector organizations, which include government entities, nonprofits, think tanks, AI safety, and academic institutes are paying serious attention to systemic and existential AI risk scenarios, but if businesses, civil society, and policymakers don’t reach at least a basic understanding of these risks, much of this work might be done in vain. In other words, the effort is there, but the awareness isn’t.
On a different yet equally crucial note, the exponential rate at which AI advances and proliferates further necessitates profound consideration for systemic and existential AI risks, especially as AI systems are progressively integrated into physical and digital infrastructures, high-stakes decision-making environments, weapons systems and manufacturing, law enforcement and intelligence agencies, biochemical and pharmaceutical research, and a rapidly increasing variety of edge devices (also known as IoT devices), from simple wearables to smart homes, accessible to consumers of all profiles.
To make things more complicated, the risks inspired by these kinds of use contexts are distinct from the risks tied to increasingly sophisticated AI agents, multi-agent systems, deceptive AI, AI race dynamics, and malicious AI actors, which, if they were to overlap with any of these use contexts, would introduce unprecedented complexity and uncertainty.
While building awareness around systemic and existential AI risk scenarios is paramount to establishing the conditions for a universally safe and beneficial AI-driven future, we have no intention of fear-mongering our readers. Admittedly, removing the element of fear from the discussions we’ll entertain in this series is perhaps an impossible task—particularly in light of the AI sci-fi narratives that many are already familiar with—but this doesn’t detract from our overarching goal: to provide our readers with a realistic understanding of systemic and existential AI risks.
Existential and Systemic AI Risks: Introduction
Before we examine (in parts 2 and 3 of this series) the array of systemic and existential AI risks that currently exist, we’ll first define these two terms precisely, after which we’ll briefly discuss two contrasting arguments concerning the trajectory of a catastrophic AI-driven outcome. Moving forward, we urge readers to keep these two arguments in mind, thinking critically about them throughout the duration of this series.
- Systemic risk: Risks that severely compromise the continued functioning of an entire system, not just its parts. Systemic risks, depending on accumulation trends, cascading failure phenomena, and what kinds of systems are affected, can also perpetuate existential risks. Some key categories of systemic risks are described below:
- Democracy and society: Risks that threaten the overall functioning of democracy and society, democratic and societal structures, or democratic rule of law at the scale of one or multiple nations.
- Critical infrastructures: Risks that threaten the continued functioning of critical digital and physical infrastructures like banking systems, electrical grids, emergency response centers, or water treatment plants.
- Organizations: Risks that compromise the overall functioning of an organization, provided that it’s sufficiently large—organizations are themselves complex systems but scale is also a factor here, so anything larger than a small-to-medium-sized enterprise (SME) could be more prone to organizational systemic risks.
- Critical supply chains: Risks that threaten the continued functioning of critical supply chains like pharmaceuticals, food processing and distribution, oil and gas pipelines, and semiconductor manufacturing.
- Environmental sustainability: Risks that can profoundly destabilize natural ecosystems or sustainability infrastructures like nuclear power plants, and solar and wind farms.
- Existential risk: Risks that irreversibly threaten the continued survival and existence of humanity, or similarly, risks that can perpetuate large-scale (i.e., affect an entire population) harms. We’ll refer to these risks by their formal denomination as x-risks. However, under the umbrella of x-risks, fall several other pertinent risk categories, which are defined below:
- S-risks: Risks of extreme and unprecedented human suffering, like global mass starvation. Importantly, s-risks imply a scale of human suffering that far exceeds anything humans have historically experienced—mass starvation at the scale of an individual nation likely wouldn’t be categorized as an s-risk. By contrast, mass starvation at the scale of an entire continent or even the globe would qualify as an s-risk. While a concrete s-risk threshold doesn’t exist, the scope of a potential risk scenario, both in terms of scale and impact on future generations, will most strongly influence whether it qualifies as an s-risk.
- Sx-risks: X-risks that arise due to systemic risk factors, like the collapse of democracy. In extreme cases, the collapse of democracy could perpetuate widespread societal instability that results in violent conflicts whereby an entire population inadvertently annihilates itself.
- I-risks: Predicated on the Japanese notion of Ikigai, these risks are more philosophical in nature, extinguishing humans’ sense of meaning and purpose in the world. For example, AI-induced over-optimization could lead people to lose their sense of personal growth and fulfillment, which could create existential conditions in which life is regarded as purposeless and not worth living.
- Ax-risks: Risks that pose a threat to the continued survival and existence of sentient or conscious AI systems or digital minds. While arguably less important than their counterparts, these risk scenarios grapple with abstract ethical questions concerning the moral status of future AI systems or digital minds. (We won’t discuss these risks in this series since they fall beyond the scope of our discussion, although for readers interested in learning more about them, we suggest this pivotal paper by Nick Bostrom).
As for the x-risk trajectory arguments, we must first consider two central theses:
- Orthogonality thesis: The idea that the goals and intelligence of an AI system are largely independent of one another. For instance, an ASI could display goals that are entirely alien to humans. For reference, see this thought experiment.
- Instrumental convergence thesis: The idea that as an AI system becomes more intelligent, it will display emergent goals that intrinsically favor self-preservation. For example, an AGI might realize that it will need to exploit more and more resources to continue functioning, eventually perpetuating a resource pool collapse.
Both these theses lay the foundation for the decisive x-risk hypothesis and the accumulative x-risk hypothesis. The decisive x-risk hypothesis posits that an x-risk scenario will materialize dramatically and abruptly, in the form of a crippling catastrophic event that either leads to human extinction or irreversible large-scale harm. This hypothesis more closely aligns with the orthogonality thesis, seeing as it implies that due to the status of intelligence and goals as independent variables, we won’t be able to predict with a sufficient degree of certainty, whether an AGI or ASI would be benevolent, malicious, or completely indifferent to human existence.
Conversely, the accumulative x-risk hypothesis suggests that incremental increases in the severity and breadth of non-existential AI risks will steadily erode human systems until an x-risk materializes. This hypothesis is more strongly substantiated by the instrumental convergence thesis, which suggests that we may be able to predict, with some certainty, the degree to which increases in AI intelligence will motivate the emergence of instrumental self-preservation goals that pose catastrophic consequences for continued human existence. Seeing as the most advanced AI systems today are being released iteratively, tending to behave as rational agents would, the instrumental convergence hypothesis appears to be the more likely path toward potential x-risk scenarios.
Conclusion
Now that we’ve covered the foundation for our ensuing discussion, we can begin our detailed exploration of systemic and existential AI risks. Seeing as this material is quite dense and heavy, we’ve split up the next few pieces according to the following topics (in this order): systemic AI risks, followed by existential AI risks, concluding with a final discussion and analysis.
It’s worth noting that while the majority of the risks we’ll point out in the next pieces are supported by existing literature, we do suggest some additional risk scenarios not well-covered in the literature. Despite their lack of an empirical basis, these latter kinds of risks are not less important than their counterparts, and we hope that by identifying and exploring them, we’ll positively contribute to ongoing research in the AI safety, ethics, and risk management space.
For readers interested in further exploring the AI risk landscape alongside other relevant topics across AI policy, governance, generative, and responsible AI (RAI), we invite you to follow Lumenova AI’s blog.
For those who are actively exploring or initiating AI governance and risk management protocols, we suggest checking out Lumenova AI’s RAI platform and booking a product demo today.
Existential and Systemic AI Risks Series
Existential and Systemic AI Risks: A Brief Introduction
Existential and Systemic AI Risks: Systemic Risks
Existential and Systemic AI Risks: Existential Risks
Existential and Systemic AI Risks: Prevention and Evaluation