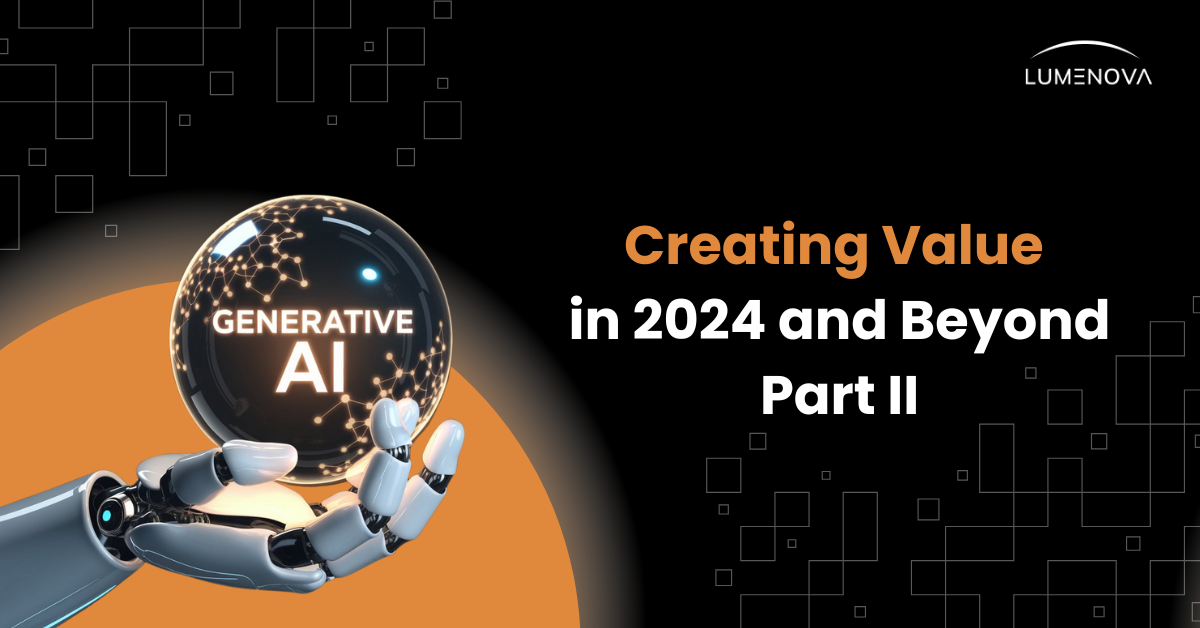
Contents
Why Collective Thought on the Future of GenAI is Vital
Whether you’re on the AI hype or skeptic train, somewhere in between, or missed the train entirely, affording time and effort to think carefully, critically, and pragmatically about the future of generative AI (GenAI) is more than just a thought experiment—it’s a technological imperative. While the term AI does encompass much more than “just” GenAI tools and applications, and many AI breakthroughs are likely to occur outside the GenAI space, GenAI adoption rates have now outpaced those for all other kinds of AI products and services.
This isn’t to say that GenAI is inherently more valuable or even useful—in truth, the reasons for this adoption-centric advantage warrant an entirely separate discussion—than other kinds of AI. However, this trend does highlight a reality that we must accept: as the most currently popularized form of AI, GenAI is poised to exercise a stronger influence on the near-term future of AI than any other available AI technologies. GenAI already has a tight grip on us and this grip will only tighten as it continues to advance and proliferate.
And yet, despite this tightening grip, the collective majority (i.e., the general public) still fails to seriously consider how this technology might evolve, alter the world we live in, and redefine the very notions and experiences in which we base our humanity and survival, assuming that “things will work themselves out” in one way or another. If this were true, we’d already have concrete answers to the questions below, or at least, evidence-driven approximations that are acceptable to the majority. Can we claim, with certainty, that GenAI is the future of AI? The fact that this simple question is controversial demonstrates how little collective thought—”collective” is the keyword here (there are plenty of individuals who are thinking about this)—has been funneled into anticipating the near-term, let alone long-term, trajectory of this technology. The critical questions illustrated below, due to their lack of concrete, or even approximate answers, further bolster this point.
-
How will GenAI tools change and evolve over the next five years, and what kind of GenAI technologies can we envision in the near term?
-
What purpose will these technologies serve, what solutions will they offer, and what risks and vulnerabilities will they introduce?
-
What core factors will drive the development and emergence of these technologies, and will these factors remain consistent over time?
-
How will trust and confidence in GenAI shift as it becomes more embedded into daily life?
-
How will the dynamics and value of digital information ecosystems adapt to increasing influxes of AI-generated content?
-
What industries will experience the most significant GenAI-driven disruptions and transformations, and how will workers adapt to these fundamental changes?
-
How will personal and professional skills repertoires metamorphose, mature, and/or regress as GenAI tools become more accessible, popularized, and sophisticated?
-
How will human creativity, novelty, and authenticity evolve alongside GenAI innovations?
-
What role will governments play in GenAI innovation and how will they execute that role while preserving and reflecting the interests of those they govern?
Of course, answering any future-oriented questions with certainty is a dangerous endeavor because predictions are approximate at best, and they must be framed as such. That being said, in this piece and the one that follows, we aim to address most of these questions concretely, recognizing that even if we’re completely wrong, something positive will come of it—the grounds on which a given prediction is conclusively deemed false are concrete and therefore serve as a reliable mechanism through which to engage in a meaningful, realistic, and useful dialogue on the future of GenAI.
Before moving on, we’d also like to address a possible counterargument to our earlier point on collective thought—the masses make bad decisions, so it’s best to leave predictions on the future of GenAI to the experts. First, this argument is predicated upon a grossly unsubstantiated assumption: experts consistently make the right predictions. If unconvinced by this point, consider how many so-called “experts” completely failed to predict major world events like the 2008 financial crisis, the 2016 US presidential election results, the UK’s Brexit Referendum, and most notably, the Covid-19 pandemic. These prediction failures are especially potent examples because in each case, a suppressed expert minority did predict their emergence, despite being drowned out by the vocal majority.
Second, the notion of expertise is innately narrow—expertise is confined to a specific field or practice—meaning that experts are more likely to make decisions that reflect their non-generalizable interests. To be clear, this is not an indictment of experts, particularly since this tendency doesn’t typically stem from malice or egocentrism, but rather, a more subtle and foundational problem—expertise, by default, fosters thought processes that operate in the absence of a holistic world model. In the previously mentioned prediction failure cases, experts who made the right predictions were able to do so because they viewed a situation from multiple perspectives and disciplines, operationalizing their expertise within a comprehensive world model informed by their curiosity and collaboration with other open-minded experts. In a nutshell, we need experts as much as ever, but we also need them to think bigger and more collaboratively, considering how the complex underlying dynamics of the real world affect the value and utility of their expertise over time.
Finally, becoming an expert at anything requires excess time and resources, a luxury that most people simply can’t afford due to real-world constraints. While there may be a few who muscle their way to expertise through sheer ambition, work ethic, and willpower, most experts, even if they possess these qualities, will embark on the path toward expertise by exploiting the already existing privileges and opportunities at their disposal—in the words of Malcolm Gladwell, “to build a better world we need to replace the patchwork of lucky breaks and arbitrary advantages today that determine success.”
Simply put, if you want to become an expert at something, chances are you aren’t thinking about how you’ll put food on the table tomorrow, afford healthcare costs, or put your kids through school. If you’re skeptical of this point, do a quick Google search on the top 5 global experts in any industry and take a look at their educational and financial backgrounds. Expert opinions don’t always reflect the interests of the general public.
In the next sections, we’ll cover the main factors that are driving GenAI innovation after which we’ll make a series of technology predictions for 2024 and beyond. In doing so, we’ll do our best to stay true to the points we’ve just discussed, ensuring that this discussion is accessible to a wide audience while fostering a multi-disciplinary dialogue that favors collective thinking—in other words, why does thinking about the future of GenAI matter for you?
For those who crave a little more background information and context, we advise reading the first piece in this series, in which we cover various documented cases of real-world AI successes and failures across multiple industries.
Factors Driving GenAI Innovation
Investment interest, potential business value, and AI research advancements aren’t the only factors driving GenAI innovation—we won’t address these factors here, not because they’re unimportant (which they certainly aren’t) but because they’re obvious and wouldn’t add any original value to our discussion. Nonetheless, with a technology that’s this widespread and impactful, understanding the complicated interplay between the forces that drive its further innovation is crucial for several reasons, which are described below:
-
Understanding which factors drive GenAI innovation allows you to ground your predictions and intuitions about the future of GenAI in something tangible. This won’t eliminate uncertainty by any means, but it will help mitigate it.
-
GenAI will continue to permeate both the professional and personal realms, meaning that its impacts won’t be confined to businesses and organizations but also day-to-day life, society, and culture. At some point, everyone will feel the impacts of GenAI, regardless of whether they’re aware of them or not.
-
Maximizing the value and utility of GenAI requires individuals and organizations to build and maintain a robust GenAI-specific skillset. Knowing which skills to identify and prioritize as the technology moves forward will be critical to enacting its value in targeted contexts, whether personal or professional.
-
The foundations on which society, industry, and government are built may appear stagnant though they are far from it. GenAI will fundamentally alter many of the systems in which we exist today, and if we are to strive toward positive change, comprehending the dynamics of GenAI-influenced transformations will play a pivotal role in this process.
-
GenAI technologies, especially as they become more sophisticated, introduce a wide array of systemic and existential risks, depending on what they’re designed and used for. Proactive risk mitigation measures must consider the evolution of this technology from a holistic and future-oriented perspective, otherwise, they’re doomed to fail.
There are many more reasons we could list to make our point, but in the interest of keeping things succinct, let’s dive into the core factors driving GenAI innovation. Below, we’ve listed these factors in order from most to least influential, roughly envisioning what we believe to be the most salient forces behind GenAI innovation (omitting business value, investment interest, and AI research advancements). We recognize that as the future unfolds, certain factors we discuss will shift up or down the list, and it’s precisely through this possible controversy that we hope to encourage more meaningful discussion and engagement—to know what’s right, we need to know what’s wrong.
-
AI Arms Race Dynamics: The global stage is anarchical—power is seized, not acquired. GenAI’s enormous and largely untapped potential to dramatically improve military operations and national security provisions positions it as one of the most crucial modern-day assets for nations to possess if they are to cement their adversarial advantages. The nations that end up developing and integrating the most sophisticated forms of this technology will likely dominate the global stage, and this incentive will supersede all domestic incentives, even if nations aren’t willing to admit it.
-
Computational Power and Cloud Infrastructure: GenAI requires colossal amounts of computational resources, both in terms of compute and infrastructure, to develop, train, and run advanced, large-scale models. As these resources are depleted and costs correspondingly increase, GenAI developers will need to derive novel methods for scaling and improving GenAI tools and applications efficiently.
-
Data Availability and Quality: Despite the apparent wealth of available data throughout the global digital information ecosystem, data quality and availability will continue to present significant challenges for GenAI innovation. Limited high-quality data has been a central concern from the beginning, and as the digital information ecosystem becomes more saturated with synthetic AI-generated data, the illusion of data availability will be enforced, especially as the line between human-made and AI-generated content is blurred.
-
Increasing Collective AI Literacy: Right now, very few people are AI literate, notwithstanding early adopters. However, as collective AI literacy rates build, particularly among non-technical professionals and young, technology-native populations, consumers will begin developing their own ideas and preferences for what they seek to gain from and use GenAI for. The consumer-centric knowledge-based evolution of these ideas and preferences will significantly shape the future design, development, and purpose of GenAI tools.
-
Low Barrier Innovation Tools and No-Code Platforms: The rising popularity of low-code/no-code platforms—Akkio, DataRobot, and RunwayML—especially when coupled with the custom GPT and AI Agent building features offered by other state-of-the-art platforms—OpenAI, Mistral AI, and Google Vertex AI—have practically eliminated many of the conventional technical barriers dissuading non-technical users from building GenAI tools and applications. This relative democratization of GenAI technology is accelerating the pace of AI innovation while also cultivating and encouraging a much more diverse tools landscape.
-
Need for Self-Actualization: While a more subtle and less influential factor at this time, the innate and fundamental human need for self-actualization will play an increasingly potent part in the future of GenAI innovation. As more people adopt GenAI into their personal and professional lives, they will begin tapping into the technology’s potential to foster personal growth and discovery, realizing benefits that would’ve taken them years to realize on their own. How GenAI is used both individually and at scale to fulfill human needs for self-actualization will likely redefine the technology’s scope and application in multiple ways.
-
Mimetic Desire and Social Influence: As creatures with profoundly pro-social tendencies—which have been instrumental to our survival—humans care deeply about what others in their social groups are doing. The mimetic desire to replicate the successful strategies that peers, coworkers, and organizations have implemented, specifically in the context of early GenAI adoption, will substantially impact near-term trends in AI deployment, adoption, and integration initiatives across numerous domains and industries. In many cases, however, these pressures could result in rushed and poorly designed GenAI initiatives.
-
Emerging Regulations and Slow-Burn Regulatory Loopholes: The EU AI Act remains the only comprehensive AI regulation designed and implemented to date, and while we’re not certain how it will impact GenAI innovation, we know that it will. The EU AI Act, along with other emerging AI regulations throughout various nations, will catastrophically fail in some areas while succeeding in others, exposing regulatory loopholes while showcasing relevant strengths. The lessons learned from these early-stage regulatory successes and failures will trickle into other AI regulations worldwide, exercising multi-faceted compliance pressures on the global GenAI innovation landscape.
-
User-Generated Data Feedback Loops: Advanced GenAI models like ChatGPT and Claude can learn from and adapt to user interaction through user-generated data feedback loops. These loops help models personalize their interactions, align with user preferences and skillsets, and improve capabilities repertoires—feedback loops can also help developers uncover model vulnerabilities, risks, and limitations. As people continue to adopt AI on progressively larger scales, the growing richness and diversity of user-generated feedback loops will position them as high-value data assets leveraged for further model improvement and remediation. The implicit role that users play in improving GenAI capabilities will steadily strengthen.
-
GenAI’s Invisibility: GenAI’s ability to seamlessly integrate with a variety of edge devices (e.g, Smartphones, AVs, Wearables, etc.) and different kinds of software-based services like Google Docs or Spotify indicates that humans benefit from and leverage GenAI much more than they are aware of. This covert appearance suggests that non-obvious opportunities for GenAI integration and adoption are much more plentiful than we think, especially as distributed computing becomes more popularized, enabling new forms of GenAI innovation that prioritize low-latency, real-time responses without relying on centralized cloud services. In this respect, novel opportunities for GenAI integration and enhancement will continue to emerge in fields like gaming, VR, and AR, significantly broadening GenAI’s applicability scope.
-
Digital Nomadism and Remote Work Culture: Remote work arrangements have been on the rise, particularly since the COVID-19 pandemic, with some projections estimating that by 2025, over 32 million Americans will be working from home. For this digital nomad lifestyle to benefit both workers and their companies, especially as remote work arrangements become more frequent, access to advanced digital tools that foster collaboration, productivity, and creativity will become a necessity. Fortunately, GenAI is perfectly situated to capitalize on this continual shift, enabling the creation and deployment of tools that support asynchronous work, digital collaboration, and creative ideation without location-based constraints.
-
Fear of Automation: The now viral quote, “AI won’t replace you, but someone using AI will” is misleading. While GenAI will create new jobs and change others, it’s virtually impossible to deny that it will automate many existing professions, especially across fields like customer service, administrative functions, data analytics, manufacturing, and retail—the fear of automation is both real and legitimate. To diminish automation fears and build trust among consumers, GenAI companies may respond to these pressures by focusing more on developing and deploying hybrid solutions where GenAI compliments and enhances rather than replaces human efforts.
-
Shifting Intellectual Property (IP) Norms: Since ChatGPT’s release in 2022, IP rights concerns linked to data scraping practices and AI-generated content have reached the forefront of the legal discourse, particularly among creative communities. Depending on how existing lawsuits against GenAI companies are handled, shifting IP norms could push GenAI developers to exercise much more caution and consideration when building models intended for creative content generation. On the other hand, these situations also stress the critical need for technical methods and solutions for authenticating AI-generated content, which will intensify as it becomes increasingly difficult to distinguish between human-created and AI-generated content.
-
Environmental and Sustainability Pressures: In this context, GenAI has a two-fold objective—reducing its own energy consumption, carbon footprint, and e-waste while also enabling novel and effective environmental sustainability solutions, particularly for supply chain efficiency, sustainable building and agriculture, resource optimization, and environmental impact testing. Seeing as sustainability concerns aren’t likely to dwindle anytime soon, GenAI companies won’t be able to ignore them for much longer, especially if they intend to make a scalable and positive impact in the sustainability sector.
-
AI Ethics and Safety Initiatives: It may surprise readers that we’ve placed what’s arguably the most important factor in GenAI development at the end of this list, but there’s a good reason for it—the safety and ethics precedent set by Big Tech over the last two decades is virtually non-existent. Big Tech has a robust history of privacy and human rights violations, the vast majority of which have been swept under the rug via legal settlements. The current lack of comprehensive federal regulations, low AI literacy rates, and AI race dynamics further weaken incentives for compliance with voluntary ethics and safety standards. While some GenAI companies will take care to preserve ethics and safety in product deployments, others will eventually be forced to confront their importance through hard wake-up calls, likely in the form of catastrophic and large-scale AI failures.
Before transitioning to the next section, we challenge readers to take a moment and pick a few factors in this list that stand out to them. Consider the interplay between the factors you select and try to anticipate how changes in one factor’s influence may affect the saliency of the other factors you chose.
Technology Predictions for 2024 and Beyond
Largely based on the factors we’ve just discussed, this section offers a series of concise technology predictions on the near-term future—roughly 5 years from now—of GenAI, which is illustrated below:
-
Governance AI → As GenAI systems assume more consequential roles in critical decision-making domains, the notion of autonomous AI governance will become more appealing, particularly as bureaucratic requirements continue to hinder governmental and organizational efficiency. We expect to see the first governance AIs deployed in localized and constrained environments like small cities, districts, or even companies.
-
AI Brains → Not to be confused with artificial general intelligence (AGI), the first “AI brains” will emerge as powerful conglomerations of various advanced GenAI models designed for functions that mimic human sensory and cognitive experiences. For example, the following models, all built by OpenAI, can be viewed as analogous to various regions of the brain: GPT-4 (language & reasoning → Frontal Lobe), o1-preview (complex reasoning & problem-solving → Prefrontal Cortex), Whisper (text-to-speech & audio → Auditory Cortex), DALL-E (image generation → Visual Cortex), and Sora (multimodal understanding & interaction → Parietal Lobe).
-
Thought-to-Content Generation → Depending on the integration success, commercial cost, and federal approval rate of current state-of-the-art neural interfaces (e.g., Neuralink), users will be able to interact with advanced GenAI models without the need for natural language, using only their thoughts to guide and produce model outputs. However, though-to-content generation will have a steep learning curve and will take time to become commercially viable, even for minimally invasive neural interface products. Initial deployments will occur in clinical medical settings with patients who are unable to verbally communicate with the external world despite being cognitively intact.
-
Augmented Reality (AR) Universes → AI-generated, fully immersive AR environments that support hyper-personalized worlds and experiences, adapting to users in real-time based on their emotions, past behaviors, body language, and biometric feedback from wearables. These AR universes are likely to make their debut within the gaming and VR sector, however, we wouldn’t be surprised to also witness early deployment attempts from online streaming companies like Netflix, which are already beta-testing interactive, narrative-driven games on their platforms.
-
AI-Generated Knowledge Universes → AI-generated “hive minds” or “collective consciousnesses” in which numerous users can dynamically interact with each other, uploading and sharing knowledge and ideas that AI seamlessly blends into cohesive, emergent creations. These knowledge universes will support multi-lingual and multi-cultural communication, actively reinforcing the increasingly close relationship between humans and AI while fostering potentially global human-AI collaboration efforts. This technology will be especially appealing to academic researchers.
-
AI-Generated Alternate Realities → Similarly to AR universes, AI-generated alternate realities will serve as fully immersive digital environments with haptic feedback capabilities, enabling users to replicate, re-create, or build novel physical environments. These environments will be holistic, allowing users to live, work, and socialize within them as they would in the real world. If the ethics, safety, and legal concerns linked to this technology are resolved before early deployments, we expect to note the first viable use cases within professional settings—companies that are 100% virtual, both in form and function.
-
Hyper-Personalized AI Companions → GenAI-powered companions designed to learn users' personalities in intricate detail and mimic the personality traits that users find comforting, useful, or interesting. These AIs will allow users to select from certain personality profiles in real-time, set personality preferences and track personality changes, and create digital personality clones of their friends or themselves. Obvious ethical concerns—bordering on dystopian—will determine whether this technology comes to fruition. Nonetheless, we expect early use cases to emerge in assisted living facilities and nursing homes, where human loneliness and isolation often compromise well-being.
-
AI Researchers and Scientists → Purpose-built GenAI-powered agents designed to execute various research tasks and develop/run novel experimental paradigms to streamline the rate of scientific discovery. These agents will work alongside human scientists, although in much smaller teams, and the first significant use cases will likely pop up in high-impact fields like medical research and pharmaceuticals, materials manufacturing, sustainability and environmental impacts, and, believe it or not, AI research.
-
Autonomous Society Simulations → Fully AI-generated societies, composed of various individual AI agents that learn about one another and build relationships, communicate about their daily “lives” and work, develop interests and hobbies, and even pursue emergent goals or objectives. The purpose of these simulated societies will be to model human behavior at scale and test the impact of certain events before they happen in the real world. Economists, policymakers, and historians will be among the first groups to leverage this technology.
-
Time AI → GenAI systems that manipulate users’ perception of time within physical, AR, and VR environments by leveraging covert mindfulness and meditation techniques that reflect and modify users’ emotional tendencies, routines, and daily tasks. In VR environments, Time AI will further allow users to experience alternate historical timelines and events—in the past and future—while also enabling them to construct “what-if” scenarios to manipulate the potential flow of historical and hypothetical events. The gaming and VR community will be the first to capitalize on this technology, however, less obvious use cases could emerge in workplace settings, particularly where workers must complete mundane or time-consuming tasks regularly.
These predictions are intentionally bold and ambitious because we want to push our readers to think unconventionally and critically about the future of GenAI—predicting the obvious is pointless. In truth, even leading state-of-the-art companies like Google and OpenAI, despite what they’re doing behind the scenes, can’t be certain about how this technology will evolve in the near term. What we do know, however, is that GenAI, like most other technologies that came before it, will continue to advance and proliferate exponentially—as predominantly linear thinkers, what we expect will happen is not what will happen.
Conclusion
Throughout this admittedly dense discussion, we’ve covered several key points: 1) the reasons for which collective thought on the future of GenAI is crucial, 2) the core factors affecting the near-term evolutionary trajectory of GenAI, and 3) technology predictions for the next 5 years.
In doing so, we’ve conducted a thorough, grounded, and original (hopefully) examination of the path GenAI could follow. Importantly, this path won’t just involve GenAI companies and developers, and if you’re concerned with the future of this technology—irrespective of whether your concerns are positive or negative—the best way to ensure that your voice is represented is by engaging with GenAI from multiple practical, experimental, cognitive, theoretical, and social perspectives. If this feels like a daunting task, it’s because it is—the sooner you get started, the better.
Readers may have also noted that we didn’t cover several of the questions we posed in our introduction. Fortunately, these questions will form the basis of our third and final post in this series, where we explore the potential social and governmental impacts of GenAI over the next few years. This final piece will be more experimental and open-ended than its predecessors, seeing as social dynamics are more volatile and unpredictable, particularly in the age of AI in which precedents for understanding social evolution have not yet been established.
For those interested in exploring additional content on GenAI, AI regulation and governance, risk management, and other noteworthy developments within the AI landscape, we suggest following Lumenova AI’s blog. If you find yourself craving more in-depth analytical, experimental, and thought-leadership content, check out the “Deep Dives” section on our blog.
Alternatively, if you’ve already undertaken AI governance and risk management efforts, or are simply interested in beginning to identify and build AI governance and risk management strategies, we invite you to test Lumenova’s Responsible AI platform and book a product demo today.