September 30, 2024
Governing Generative AI in Finance: A Balanced Approach
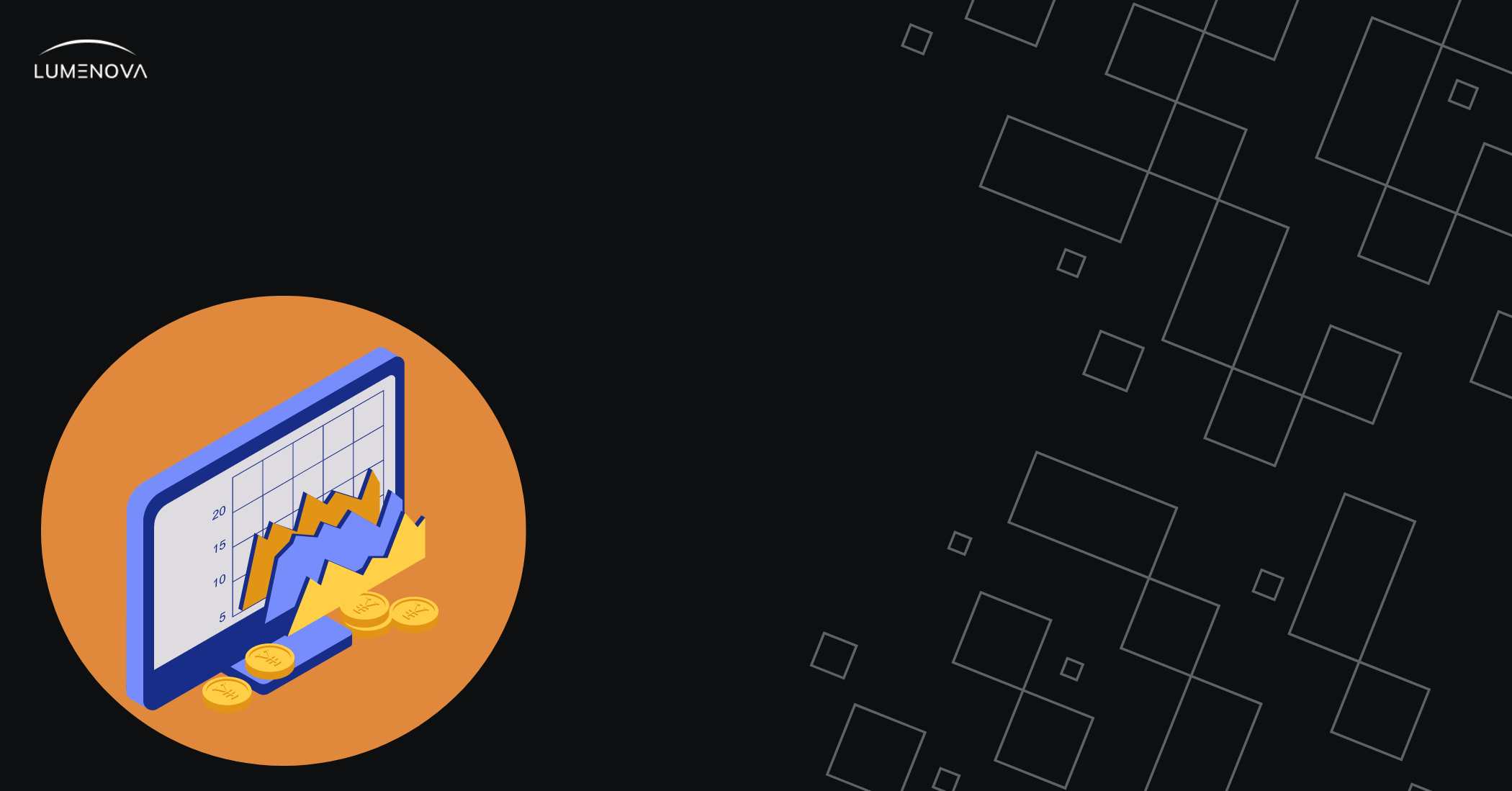
Contents
Generative AI is revolutionizing the finance industry by enhancing efficiency, accuracy, and adaptability across various functions. For instance, organizations are leveraging GenAI for developing forecasts and budgets, with a KPMG survey showing that starting last year, 83% of respondents use AI in financial planning for predictive models, scenario creation, and budget insights.
So, today, AI-generated financial commentary and presentations are reducing the time and effort required for recurring financial reports by automating data imports and generating insights. Large language models are being used to gather market intelligence, producing competitive and customer insights that are tailored to specific regions or personas. Furthermore, GenAI is also being used to produce strategic insights from customer relationship management (CRM) and enterprise resource planning (ERP) data, aiding in critical business decisions and solving pricing or performance issues.
Beyond these applications, GenAI is also improving contract management by streamlining the generation of contracts, particularly those with non-standard terms, and ensuring accurate documentation of revenue-related clauses. Additionally, GenAI is playing a crucial role in detecting anomalies and preventing fraud by analyzing data for irregularities and enhancing financial security through continuous transaction monitoring. The integration of GenAI’s large language models into the financial sector marks a significant advancement, offering a strategic path forward while addressing the challenges of adoption. As this technology continues to evolve, it is poised to drive transformative growth within the industry.
The Case for Centralized Governance
In recent years, the concept of AI governance has gained increasing attention, especially with the rise of GenAI. As AI continues to evolve, the need for structured oversight and regulation becomes more critical. Much like traditional governance models in finance, AI governance seeks to ensure compliance, mitigate risks, and promote transparency. However, with the rapid advancements in GenAI, it is clear that the current governance framework requires an update. Historically, centralized governance has been the standard in finance, particularly for managing regulatory compliance and risk.
A similar approach may be necessary for AI, but it must adapt to the complexities and unique challenges AI presents. Regulatory bodies like the SEC and the Federal Reserve provide a clear framework within which financial institutions must operate. This centralized approach ensures uniformity, consistency, and a level playing field, all of which are crucial in maintaining the stability of the financial system.
In the context of GenAI, a centralized governance model could offer several advantages. For one, it would provide a standardized set of rules and guidelines for the development and deployment of AI technologies across the industry. This could help mitigate risks associated with bias, data privacy, and security, as well as ensure that AI applications comply with existing financial regulations. Moreover, centralized oversight could facilitate the monitoring of AI systems, making it easier to identify and address potential issues before they escalate into systemic risks.
Another argument in favor of centralization is the ability to enforce accountability. In a highly regulated industry like finance, ensuring that AI systems are transparent and explainable is critical. Centralized governance could mandate that financial institutions implement rigorous auditing and reporting mechanisms, which would help regulators and stakeholders understand how AI decisions are made and ensure that they align with ethical and legal standards.
The Limitations of Centralized Governance
However, while centralization offers certain benefits, it is not without its drawbacks. One of the most significant limitations is the potential for rigidity. Financial markets are dynamic, with new risks and opportunities emerging constantly. A highly centralized governance model may struggle to keep pace with these changes, leading to outdated regulations that stifle innovation and limit the ability of financial institutions to respond to evolving market conditions.
For example, centralized regulations that are too prescriptive may hinder the development of novel GenAI applications that could offer significant value to the industry. If financial institutions are required to adhere to a rigid set of rules, they may be less likely to experiment with new AI technologies or to adopt innovative approaches that could enhance their competitiveness.
Moreover, centralized governance can create bottlenecks in decision-making processes. In a rapidly changing environment, financial institutions need to be able to make swift decisions to capitalize on emerging opportunities. Over-centralization could slow down these processes, leading to missed opportunities and reduced agility.
The Case for Decentralized Governance
On the other hand, decentralized governance offers a more flexible approach that could better accommodate the fast-paced nature of the financial industry. By allowing individual institutions greater autonomy in how they develop and deploy GenAI technologies, a decentralized model could foster innovation and enable financial organizations to quickly adapt to new challenges and opportunities.
Decentralized governance also encourages a more collaborative approach to risk management. Instead of relying solely on centralized regulators, financial institutions could work together to develop industry-wide standards and best practices for GenAI. This could lead to more effective and responsive governance, as institutions share their insights and experiences with each other, collectively addressing emerging risks and refining their AI strategies.
Furthermore, a decentralized approach could enhance resilience. In a centralized system, a failure at the top can have widespread consequences. However, in a decentralized model, individual institutions can act as independent nodes, reducing the risk of a single point of failure. This distributed approach could make the financial system more robust and better equipped to handle disruptions.
Striking the Right Balance
While both centralized and decentralized governance models have their merits, a more balanced approach that combines elements of both may be the most effective way to govern GenAI in finance. Such a hybrid model could leverage the strengths of centralization—such as uniformity, accountability, and regulatory oversight—while also incorporating the flexibility, innovation, and resilience offered by decentralization.
One way to achieve this balance is through a tiered regulatory framework. Under this model, centralized regulators could establish broad principles and guidelines for the development and use of GenAI in finance. These could include requirements for transparency, accountability, and ethical AI practices, as well as standards for data privacy and security. However, within this framework, individual institutions could have the freedom to tailor their AI strategies to their specific needs and risk profiles.
For example, regulators could mandate that all AI systems used in finance undergo regular audits to ensure compliance with ethical standards and legal requirements. However, financial institutions could be given the autonomy to determine how they implement these audits, allowing them to develop innovative solutions that align with their unique operational environments.
In addition, a balanced approach could involve the creation of industry-wide consortia or working groups, where financial institutions collaborate to develop shared standards and best practices for GenAI. These groups could operate under the guidance of centralized regulators but would have the flexibility to adapt their recommendations based on the latest developments in AI technology and the evolving needs of the industry.
The Role of Technology in Governance
Technology itself can play an important role in facilitating this balanced approach to governance. For instance, AI governance and automation ensure that artificial intelligence operates ethically and efficiently. When it comes to implementing AI governance frameworks, organizations can set clear guidelines for the development, deployment, and use of AI systems. These frameworks help to maintain transparency, fairness, and accountability in AI processes, mitigating risks such as bias or misuse. Automation plays a critical role here, allowing governance policies to be enforced consistently across all applications. Automated monitoring systems can track AI model performance, ensuring adherence to ethical standards and regulatory compliance without constant human intervention.
Additionally, automated compliance tools can streamline governance by automatically enforcing rules and policies. For example, automated auditing systems can regularly check AI outputs for biases or deviations from regulatory guidelines, reducing the need for manual reviews. This not only minimizes the risk of errors but also significantly boosts efficiency, allowing AI systems to operate smoothly while maintaining a high level of accountability. Combining AI governance with automation ensures ethical, compliant, and transparent AI deployment at scale.
Moreover, advanced data analytics tools can monitor AI performance and detect anomalies in real-time, providing early warnings of potential issues such as bias or security breaches. Machine learning algorithms can analyze patterns and trends in AI behavior, offering insights that help regulators and institutions adjust their strategies proactively. By leveraging these technologies, financial institutions can create a more responsive and adaptive governance framework, capable of evolving with the rapid pace of technological change.
In addition, cloud computing offers scalable infrastructure that can support the development and deployment of AI models across multiple institutions. This shared infrastructure can reduce costs and promote collaborative innovation while ensuring that each institution can tailor its AI applications to its specific needs. As these technologies continue to advance, they will play an increasingly important role in shaping the governance landscape for AI in finance, balancing the need for oversight with the desire for innovation and agility.
Conclusion: The Path Forward
Governing GenAI in finance requires a nuanced approach that balances the need for centralized oversight with the benefits of decentralized innovation. A hybrid model that incorporates elements of both centralization and decentralization offers a promising path forward, enabling financial institutions to harness the full potential of GenAI while managing the associated risks.
By establishing broad regulatory principles while allowing for flexibility in implementation, and by fostering collaboration between institutions through industry-wide initiatives, the financial sector can create a governance framework that is both robust and adaptable. However, high centralization will almost certainly lead to rigidity, which could create a host of problems both for managing emerging/novel GenAI risks and compliance requirements. Consequently, over-centralization will also prevent financial organizations from quickly and efficiently capitalizing on emerging high-value AI use cases.
In the end, the goal is to create a governance structure that supports the responsible and sustainable use of GenAI in finance, enabling the industry to thrive in an increasingly complex and dynamic environment.
Here, at Lumenova AI, we offer a risk management platform that can help ensure that your AI systems operate within the legal frameworks and if you want us to support you navigate the complexities of AI deployment, you can request a demo, or contact our AI experts for more details.