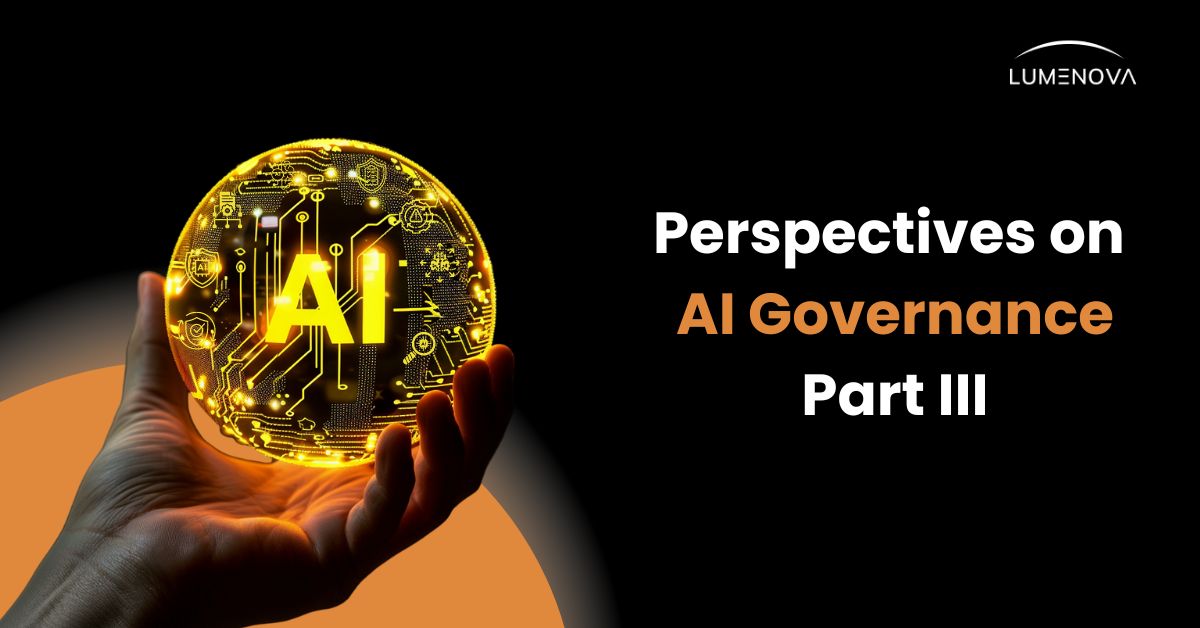
“An eye for an eye” is a phrase many are familiar with, tracing its roots back to one of humanity’s earliest written laws—the Code of Hammurabi. This phrase serves as an astute reminder and example of the longstanding intimate relationship between ethics and law, and the importance of foundational ethical principles such as fairness, justice, and reciprocity throughout time.
However, as we know, humanity’s ethical compass tends to change as civilization evolves, and as Gandhi himself put it, “An eye for an eye leaves the whole world blind.” In simple terms, laws are designed for a specific time and place, becoming outdated once the ethos of that time and place no longer corresponds with the ethos of today.
But, interestingly enough, even when ethos changes, ethics remain. Core principles in Virtue Ethics—a foundational convergent branch of both ancient Western and Eastern philosophy—continue to remain relevant today. Confucian principles such as benevolence and humaneness are integral to maintaining healthy social relationships and collectively beneficial norms whereas Aristotelian principles like temperance, prudence, and honesty play a major role in achieving and maintaining individual flourishing and happiness.
Simply put, ethics will always outlive laws—ethical principles are flexible to the point where they can be adjusted to suit our current ethos, but not so flexible that their entire foundation can be reconstructed. The concept of justice, predicated on fairness and reciprocity, is a great example of this. Throughout the 1600s, indentured servitude was common practice in the US, representing a “just” agreement between early settlers and immigrants, whose voyage to the US was subsidized by settlers in exchange for labor. Once it became clear in the early 20th century that this agreement directly exploited immigrants, failing to adhere to more modern understandings of fairness and reciprocity, indentured servitude was abolished.
So, what does all this have to do with AI governance? First off, it’s important to note our discussion of AI governance throughout this series has thus far taken an organization-centric perspective. In this respect, organizations have their own value structures, and though such value structures frequently overlap with collective morals and social norms, there are often fine nuances that emerge because, after all, an organization has specific needs and objectives that it must reach, many of which do not generalize. Ethics, in this context, is the tool through which organizations can ensure that their value propositions, objectives, needs, and governance protocols align with innovation trends, safety and risk requirements, emerging regulations, and collective expectations.
Moreover, for an AI governance framework to be effective, it must be fine-tuned for a specific scope, purpose, and context, retain enough flexibility and adaptability to accommodate change, and correspond with best practices and standards across responsible AI (RAI), risk management, and safety. Simply put, an AI governance strategy must be pragmatically oriented, and fortunately, ethics is nothing if not pragmatic—ethics grapples with what we should and shouldn’t do in the real world, given what we know about the consequences of our actions and the nature of our environment. Ethics isn’t about creating an ideal world, it’s about making our current one better.
The relationship between AI ethics and AI governance is not only intimate but crucial, especially when it comes to managing AI risks and enacting benefits. For instance, risks are defined by reference to the degree of harm they might cause whereas benefits are understood in terms of their positive impacts on human wellbeing. In the absence of ethics, our understanding of AI risks and benefits would be completely detached from the human experience, causing a slew of cascading problems across domains such as RAI development, risk management, and most importantly, governance.
Consequently, this post will argue in favor of the idea that AI governance frameworks, strategies, and approaches should be rooted in, inspired, and driven by AI ethics. First, we’ll break down several core AI ethics principles that should sit at the foundation of any AI governance framework. Then, we’ll discuss why it is that an “ethics-first” governance framework is likely to prove more effective, robust, and resilient than an “ethics-later” governance framework. Finally, we’ll conclude by exploring a series of additional AI ethics principles that we expect could emerge in the near future.
AI Ethics: Core Principles
AI ethics is a specific branch of ethics that deals with the ethics of the design, development, deployment, and evolution of AI systems. Moreover, while some argue that there are differences between the terms RAI, trustworthy AI, and ethical AI, we will use them interchangeably to avoid confusion.
Many of the principles we discuss in this section are pulled from the OECD AI Principles, Intel AI Ethics Framework, UNESCO AI Ethics Principles, and IBM AI Ethics Principles since these resources represent some of the most widely endorsed and accepted AI Ethics propositions. However, we do note that some of the principles we describe in this section are developed by those of us here at Lumenova AI.
Each principle we describe below is actionable and therefore paired with several mechanisms through which it can be implemented and upheld (readers of our first post in this series will note some overlap in this section, however, the angle we take here is ethics-centric as opposed to governance-centric). The principles are:
- Trustworthiness: AI systems should perform reliably and consistently, be developed sustainably, respect human rights and democratic values, and foster inclusive growth alongside widespread benefits. The role of an AI system and its intended purpose, especially in high-impact contexts, should also be clearly defined and communicated, and any humans involved in AI system operation should be held to a high accountability and expertise standard. Organizations that leverage or develop AI should also freely cooperate with policymakers, AI safety researchers, RAI practitioners, and consumers/users where relevant.
- Mechanisms: Human oversight and involvement, authentication of AI-generated content, and continuous maintenance of AI inventories.
- Human-Centric Design and Development: AI systems should be designed and developed with humans at their center, prioritizing human values, needs, and objectives. AI systems should possess a friendly user interface and be accessible to users of all backgrounds, and in cases where complex systems are leveraged, plain-language user guides should be provided with clear parameters and ethical guidelines that map onto organizational value structures. In cases where AI is leveraged or tested under real-world conditions, those subject to its impacts should be made aware of their rights and how to exercise them, and organizations using AI should ensure that all actors and stakeholders involved in AI initiatives are sufficiently skilled and knowledgeable.
- Mechanisms: Value alignment, RAI toolkits, user feedback loops, and inclusive design methodologies.
- Stewardship: Given how capable and widespread AI systems are becoming, especially general purpose AI systems, it’s easy to fall into the trap of failing to pinpoint who is accountable for AI impacts when they occur. Organizations must carefully consider the scope and intensity of their accountability frameworks, thinking critically about their organizational needs and objectives, and aligning their frameworks with existing regulations, RAI best practices, and designated AI use cases. Transparent protocols for dealing with serious incidents and reporting them should also be established and implemented.
- Mechanisms: Clear roles and responsibilities for key personnel, access controls, and incident response procedures.
- Transparency and Explainability: The design, function, intended purpose, outputs, use cases, risks, and impacts of an AI system should be communicated to and easily understood by all relevant stakeholders, from policymakers and upper management to end users and the general workforce. Internally, an organization should also disseminate, to key personnel, critical information on AI initiatives, integration efforts, goals, potential bottlenecks, pain points, and silos, as well as compliance and business requirements. Especially close attention should be paid to high-risk and high-impact AI systems, particularly during deployment and integration processes.
- Mechanisms: Publicly accessible communication and feedback channels, AI lifecycle documentation and reporting, and AI system registration.
- Objectivity: AI systems should perform in a non-discriminatory manner, and training data should possess a high degree of integrity, such that it accurately represents real-world contexts—data lineage should also be scrutinized appropriately. State-of-the-art techniques to identify and mitigate bias in AI models and datasets should be implemented and administered on a regular basis, and the results of these assessments should be openly reported and documented. AI systems should also be leveraged in line with their intended use cases, as specified by AI providers and developers.
- Mechanisms: Bias audits and impact assessments.
- Validity and Reliability: The outputs that AI systems produce should be both valid and reliable over time. If AI systems undergo substantial modifications or changes, such processes should be documented, and systems should be subjected to additional performance, risk, and impact assessments. AI outputs should be fact-based and truthful, and in cases where systems perform unreliably, recall or withdrawal procedures should be established and implemented to ensure that further use does not result in adverse impacts. AI system capabilities and limitations should also be communicated to those involved in AI operations.
- Mechanisms: Expert-in-the-loop, continuous testing, remediation, and improvement, risk assessments, and input validation.
- Robustness and Resilience: AI systems should perform reliably across novel and changing environments, be resistant to adversarial threats and cyber attacks, maintain operational integrity if AI infrastructures fail, and quickly recover to a functional state when failures occur. To ensure robustness and resilience, AI systems should undergo regular updates and continuous monitoring, be subjected to a wide variety of diverse environments and testing procedures, and be designed and developed with redundancy in mind to ensure that potential vulnerabilities do not result in catastrophic failures. Under certain conditions, organizations should also consider the array of potential dual-use cases stemming from the AI systems they implement or develop.
- Mechanisms: Adversarial training and testing, failure mode discovery and fail-safe implementation, and performance evaluation across novel and/or changing environments.
- Privacy and Security: AI systems, their training data, and the data they process should be managed securely and responsibly. AI systems should never be used in a way that infringes upon people’s basic rights to privacy, for instance in a workplace monitoring or biometric categorization context, and any sensitive data that is leveraged to train an AI system or is processed by it should be obtained with clear consent from data subjects. Sensitive data should also be anonymized and encrypted accordingly and security methods that address potential risks like model inversion attacks, which reverse-engineer model outputs to infer sensitive data characteristics, should be established and implemented. Organizations should also take care to protect their proprietary data and AI assets to ensure that malicious actors cannot exploit them for their own purposes.
- Mechanisms: Data governance, cybersecurity protocols, predictive threat intelligence, and identification and protection of AI assets.
- Respect for Human Dignity and Autonomy: AI systems should never be leveraged to manipulate, coerce, or undermine humans, and AI outputs should never compromise an individual’s fundamental human rights and autonomy—the ability to govern oneself. Organizations should dedicate significant time and resources to growing AI awareness and skills acquisition at scale, and ensure that their AI initiatives correspond with the concept of human augmentation as opposed to human replacement. In cases where AI-driven automation will inevitably replace human labor, organizations should establish mechanisms through which affected persons can upskill and reskill as necessary, to preserve human value and dignity.
- Mechanisms: AI skills development, awareness, and upskilling/reskilling initiatives.
- Proactive Future-Oriented Thinking: The rate at which AI innovates and proliferates necessitates that organizations adopt and endorse a proactive future-oriented mindset from the C-suite to the general workforce. Change management should be at the center of any organization’s AI integration strategy, and constant attention should be paid to AI’s transformative and disruptive impacts, not only throughout your specific industry or domain but also throughout others. Organizations should also design their AI governance frameworks with built-in flexibility and adaptability in mind, otherwise, they risk being unable to accommodate AI-driven changes, which could result in numerous adverse impacts from compliance penalties to severe reputational damage and loss of consumer trust.
- Mechanisms: Innovation, market, and use-case predictive trend analysis, AI policy analysis, and continuous investment in AI research and development.
- Anthropomorphic Prevention: Humans are quick to anthropomorphize—imbue non-human objects with human characteristics—which is notably concerning with AI systems that display human-like qualities, particularly those with natural language capabilities. Preventing the anthropomorphization of AI systems is crucial to preserving sound human judgment and reducing the possibility of excessive reliance on AI. Moreover, where conversational AI is leveraged for recommendation purposes, anthropomorphic tendencies could facilitate unfounded trust in AI, resulting in poor real-world decision-making.
- Mechanisms: Notifying and reminding users that they’re interacting with an AI system before, during, and after AI use.
- Goal Orientation and Prioritization: AI initiatives, strategies, goals, and objectives should be clearly defined and prioritized for key teams and personnel within an organization. Whether you’re developing or integrating AI, the efforts you take will require substantial time and resources, and if they are conducted in the absence of a concrete structure with tangible guidelines and a viable direction, the results will be discordant, difficult to predict, and potentially harmful. We refer to goal orientation and prioritization as an ethical principle because it ensures that any AI initiatives are purposely aligned with broader ethical values while also ensuring that AI resources and efforts are allocated to the most critical and impactful areas.
- Mechanisms: Benchmarking, KPIs, and corporate social responsibility frameworks.
- Cooperation and interoperability: To be clear, we’re not saying that AI providers or developers should open-source all of their AI models since this would, in itself, introduce a variety of risks that are difficult to mitigate and understand. Nor are we saying that organizations should freely share all of the details of their AI initiatives and assets. Rather, organizations leveraging or developing AI should cooperate with one another, as well as with other relevant government agencies, industry specialists, and AI experts to ensure that their AI efforts are aligned with the latest standards, guidelines, and best practices. Doing so will also help facilitate governance interoperability while reducing the risk of liability linked to irresponsible or disorganized AI use. At a higher level, and perhaps more importantly, cooperation and interoperability both contribute to a healthy and trustworthy AI innovation ecosystem, increasing the probability that AI systems are intentionally designed to be trustworthy and human-centric.
- Mechanisms: Industry partnerships, multi-stakeholder engagement and feedback, corporate communication channels, regulatory sandbox testing and experimentation.
Why “Ethics-First” AI Governance is the Best Approach
Regardless of whether your organization adopts an “ethics-first” vs. “ethics-later” AI governance approach, AI ethics will undoubtedly make its way into your AI governance model—AI regulations, risk management standards and guidelines, safety requirements, and RAI best practices all cover at least some characteristics and properties of AI ethics.
For instance, the EU AI Act explicitly supports human-centric and trustworthy AI development, the NIST AI Risk Management Framework and ISO 42001 both directly emphasize principles like accountability, transparency, and explainability, the UK AI Safety Institute, as a core part of its mission, aims to preserve and protect human autonomy, dignity, and democracy, whereas virtually all gold-standard RAI toolkits, whether they’re offered by government agencies or private companies, operationalize many of the AI ethics principles we’ve just discussed.
Broadly speaking, this serves as a good reason to pursue an ethics-first AI governance model—doing so will enable a higher degree of preparedness for and alignment with AI regulations, risk management standards, safety requirements, and RAI best practices. Moreover, and as we’ve alluded to in our previous posts on this topic, AI governance is a time-consuming and resource-intensive process that can quickly become complex and convoluted. While the ethics-first approach won’t eliminate all the difficult aspects of developing and implementing an AI governance strategy, it will help streamline the process by laying a foundational structure that already adheres to existing standards.
Extrapolating from this point, the ethics-first approach will also help organizations avoid falling into the trap of “compliance is all that matters.” We call this a trap because regulations will always linger behind AI advancements, and if organizations focus solely on compliance requirements, they’re likely to miss the big picture and fail to establish mechanisms that allow them to manage novel and emerging AI risks that regulations have yet to account for. In other words, the ethics-first approach forces organizations to widen their perspective and view their governance models through a more comprehensive lens.
On the other hand, the ethics-first approach implicitly supports adaptability and flexibility, which is a critical component of any successful AI governance model—AI governance models must be able to accommodate and adapt to rapid AI-driven changes. While our interpretation of AI ethics principles will change over time, the principles themselves will remain relevant. Let’s consider a concrete example: stewardship.
- Example: As our world becomes increasingly data-driven and AI models continue to proliferate and integrate into all things complex and mundane, the burden of stewardship may no longer fall primarily on organizations leveraging these technologies. Regular people will also be held to a certain accountability standard seeing as on a day-by-day basis it’s becoming progressively easier for less sophisticated actors with malicious intentions to execute significant AI-driven threats. This is an extreme example, but it should also be noted that a regular person can use AI negligently—just as we hold a Tesla driver accountable for getting into a crash while in semi-autonomous driving mode, we should also hold AI users accountable for failing to use AI systems in line with their intended purpose and use cases or without knowledge of their limitations. For instance, if an academic researcher were to use ChatGPT to conduct an automated literature review, and include these sources in a research publication only to find that half of them were hallucinations, the researcher, not OpenAI, would be responsible for failing to fact-check AI-generated outputs.
By adopting an ethics-first governance model, organizations can design AI policies that maintain relevance but remain amenable to AI-driven changes. As we see in the example above, the principle of stewardship doesn’t lose any importance, but rather, undergoes a shift in its scope. Simply put, the ethics-first approach will substantially reduce the probability the AI governance models need to be holistically re-designed from the ground up in light of AI-driven changes. On a related note, this will also help organizations determine the right bureaucratic balance for their AI governance model since the degree of bureaucracy supported by an AI governance model should roughly correspond with the degree of change management an organization expects it will have to deal with.
Moreover, as we discussed in our first post of this series, AI governance strategies must prioritize AI risks, oftentimes according to the real-world impacts they inspire. Since ethics grapples with real-world moral decision-making, and most if not all AI impacts are ethically laden in some way, AI ethics serve as a potent and effective supplementary tool for risk prioritization. Understanding the saliency and probability of AI risks and their impacts is crucial, but we also need to consider their magnitude—how severe the consequences of a risk or corresponding impact would be if it were to materialize—and this is where ethics can help us clarify the scale and intensity of any potential harm.
On a more subtle note, AI governance frameworks should encourage experimentation with AI systems—AI experimentation constitutes a foundational facet of learning how to use AI effectively, spotting potential high-value use cases, and identifying and mitigating AI risks appropriately. Obviously, this kind of experimentation should ideally be conducted in accordance with strict ethics and safety parameters that ensure a safe and secure experimentation environment. Nonetheless, even an immature AI governance framework, if it is truly ethics-first, will enable at least some degree of responsible AI experimentation, which could help inform and streamline the development of a more robust and resilient governance framework.
Finally, and in reference to another point we touched on in the first post of this series, AI governance strategies need to possess a targeted scope. Most organizations have mission statements and value propositions they aim to uphold, and an AI governance framework should easily map onto these concepts, which can be tricky since they tend to be abstract and difficult to operationalize. However, if organizations identify which principles in AI ethics are relevant to their mission statements and value propositions, they can hone in on the scope of their AI governance strategy more effectively and efficiently. Consequently, organizations become less prone to a check-the-box AI governance mindset while also reducing the risk that they adopt a generic one-size-fits-all governance model.
AI Ethics: What Principles Might We See Emerge in the Near Future?
In this penultimate section, we’ll take a look at a series of ethical principles that we expect will become relevant in light of the current evolution and trajectory of AI. It’s important to note that the principles we identify below are not drawn from any existing AI ethics frameworks but rather from philosophical frameworks and theories including virtue ethics, utilitarianism, deontology, social contract theory, bioethics, and the ethics of animal minds. Each one of the principles stated below has been modified for AI-specificity and attempts to build upon the core ethical principles we’ve already described:
- Temperance: we should practice self-control and moderation. AI offers us with many exciting opportunities to improve our wellbeing. But, we have to think critically about which areas of our lives we want to work on ourselves vs. which areas of our lives we’d like to improve by using AI. Work, whether it’s personal or professional, imbues us with a sense of purpose and direction. If we delegate too much to AI, we not only risk losing control of our own lives but also relinquishing our sense of purpose in favor of superficial conveniences. In the context of AI, temperance is the ability to understand when to use and when not to use AI, which is equally important for organizations and individuals alike.
- Courage: we should confront our challenges and fears appropriately. Don’t be intimidated by AI. This technology is here to stay, and the sooner you learn to work with it, the sooner you’ll be able to discover your niche, namely how you can continue to provide value in an increasingly AI-driven economy. As for organizations, the same applies, but also don’t hesitate to stand up for your values—if an organization finds that one of its partners is leveraging AI in a way that undermines fundamental rights, it should promptly terminate the partnership and publicly disclose the reasons for which this occurred. While this may result in some short-term damages, but it will ultimately reflect positively on the organization in question, demonstrating their trustworthiness and unwavering commitment to RAI.
- Enrichment: the environments we exist in should allow us to freely pursue our natural behaviors, provided they don’t result in significant harm to others. In the context of AI, this is more than just a respect for fundamental rights and human autonomy. AI systems should be actively designed, developed, deployed, and integrated such that natural human behaviors are rarely corrupted, prevented, or manipulated (except in targeted cases like violent crime, personalized learning, or addiction treatment). Human agency—the ability to exercise control over one’s actions and environment, which goes beyond the ability for self-governance—is vital to exercising individual decision-making power, action-oriented behavior, and the feeling of influence or control over personal life outcomes. Simply put, people need to be allowed to make mistakes, learn from them, and better themselves—AI shouldn’t solve all our problems for us.
- Universalizability: we should apply ethics principles indiscriminately and universally. With something as widespread, powerful, and continually innovative as AI a universal language of AI ethics must be established. This language should be globally motivated and easily transcend cultural boundaries to ensure that AI efforts benefit humanity as a whole, not just some country, government, or company.
- Individualistic Moral Fidelity: we should stay true to our moral compass, and in cases where we find that it’s misaligned, we should refine it accordingly. Morality is not unique to humans, and while certain moral codes or nuances might be, almost all humans are born with an intuitive understanding of morality. This doesn’t mean that we’ll always act morally, but rather, that the vast majority of us experience instinctual and immediate moral reactions—that often take the form of universal emotions like guilt, shame, excitement, fear, or surprise—in response to certain actions or events in our lives. When we’re using AI or experimenting with it, we should listen to our moral instincts and intuitions, especially during periods of uncertainty where we can’t easily predict or understand potential risks and impacts. Conversely, we should also acknowledge when AI-driven adverse impacts like discrimination are actually a direct reflection of much deeper moral problems that operate at the level of our institutions and societies—AI puts a mirror on our humanity.
- Legitimacy of Authority: rules are legitimized when they are substantiated by the consent of the governed. This principle is specific to the notion of organizational AI governance. If organizations don’t develop their AI governance strategies with the support and engagement of interested parties, AI governance models will fail to grasp all the nuances associated with AI initiatives. Importantly, the word “consent” here is not used in its classical sense, more closely corresponding with the notion of endorsement. An endorsement from those governed strongly suggests that the governance model in place adequately and comprehensively represents all the needs and objectives of interested parties.
- Anti-Hedonism: the experience of pleasure without pain is worthless. AI could dramatically reduce human suffering around the world, but it should never be intentionally designed to eliminate it entirely. A world where hedonism is the only source of value would be devoid of meaning—we cherish our relationships with others because we know that our lives are finite, we rise to face adversity because we’ve overcome failure, and we fight to create a better world so that we don’t make the same mistakes as our predecessors. Life is rich, complex, confusing, exciting, boring, weird, and sad, among many other things, and this—the contrast between all the various components of life—is ultimately what makes it worth living.
Conclusion
We’ve rounded out our Perspectives on AI Governance series with a more lofty and philosophical discussion than those in our previous posts. In this spirit, we’d like to conclude by challenging readers to think about their philosophies and ethics of AI by entertaining the following questions:
- In the age of AI, what values are most important to you and why, and what societal or cultural values do you think have become outdated or require reconsideration? Can you identify any experiences in your daily life where your values have been directly threatened, challenged, or influenced by AI or someone using AI?
- When you think about AI governance, both independently and in relation to AI ethics, what worries or excites you about it? Do you think that AI governance is the answer to safe, secure, and trustworthy AI, or do you think that something else is needed? If so, what might it be?
- If you could envision an ideal AI-driven world or society, what would it look like? Who (or what) would govern it and how? How would people find meaning and purpose? How would this future society be different from the one we live in today?
- Of all the principles we’ve discussed in this post, are there any that stand out as more important, interesting, or perplexing than others, and why? Do you find yourself vehemently disagreeing with any of the principles suggested or points made, and what’s the nature of this disagreement?
- If you were to develop your own AI governance framework and had all the time, resources, and expertise you require, would you adopt an ethics-first or an ethics-later approach, and why? Based on your current understanding of AI governance, where do you think it’s likely to fail vs. succeed?
- AI ethics, governance, risk management, and safety are all integral components of RAI. What do you think the RAI landscape is missing? Can you think of any RAI initiatives that, though well-meaning, are poorly constructed, implemented, or administered?
- If there was one thing you could do to guarantee an AI-driven future that benefits all of humanity, what would it be? Alternatively, if you had the option to stop all AI development and research now, would you take it?
There are no right answers to these questions, only ones that are better and worse. Regardless, if you choose to take these questions seriously, you’ll find that they reveal profound insights into your knowledge and understanding of AI governance and ethics, while also prompting you to venture beyond your assumptions and creatively consider what kind of world you would like to be a part of. Not all of us are philosophers at heart, but that doesn’t mean we can’t philosophize. And, funnily enough, philosophy is arguably more important today than it ever has been.
For readers interested in exploring additional topics related to AI governance, safety, risk management, generative AI, and RAI, we recommend that you follow Lumenova AI’s blog.
For those who are actively developing or implementing their AI governance and risk management frameworks, we invite you to check out Lumenova AI’s platform and book a product demo today.
Perspectives on AI Governance Series
Perspectives on AI Governance: AI Governance Frameworks
Perspectives on AI Governance: Do’s and Don’ts
The Intersection Between AI Ethics and AI Governance