January 23, 2024
Demystifying Responsible AI Governance: 4 Myths to Let Go Of in 2024
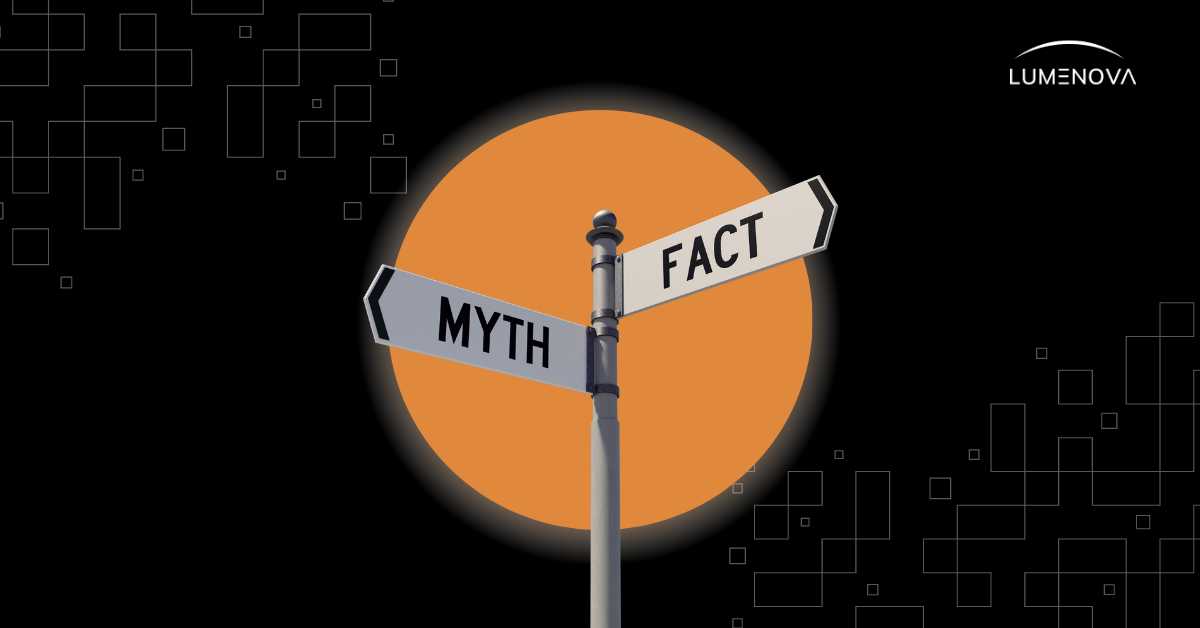
As the world’s reliance on AI continues to grow, one question persists: are these systems to be trusted?
Consumers, corporate clients, and employees expect businesses to adhere to ethical standards in the adoption and development of AI. And while a vast majority of business leaders acknowledge the importance of clear and transparent guidelines for the responsible use of AI, as shown in a recent survey, only a minority indicate a comprehensive understanding of what Responsible AI truly is.
Beyond this ambiguity regarding Responsible AI implementation across the organization, we noticed there’s also an increasing number of myths and misconceptions revolving around the topic of Responsible AI. This makes it even more difficult for business leaders to make decisions based on adequate information and facts.
For this reason, today’s article aims to dispel four prevalent myths surrounding Responsible AI, offering clarity on its implications for businesses.
Before we start, let’s briefly review what Responsible AI is:
💡 Responsible AI refers to the development, deployment, and use of AI in ways that empower individuals and businesses, exerting a fair and positive impact on customers and society at large. This approach enables companies to cultivate trust and confidently scale AI initiatives.
Myth 1: Responsible AI Practices Will Only Slow Us Down
There’s a fear that rigorous AI governance practices could stifle innovation, creating barriers and bureaucratic hurdles for developers and organizations. Advocates for a more flexible environment argue that a more lenient approach would allow AI to advance faster. This, we think, stems from the outdated viewpoint that GRC processes often slow down technological advancements and cause a delay in achieving business value.
But while risk management used to be a slow, laborious, and often disconnected process involving spreadsheets, checklists, and lots of emails, the reality of today’s enterprise landscape is far removed from this image.
At Lumenova AI, we firmly believe that responsible AI governance, when implemented correctly, does not need to be a hindrance to innovation.
There are practical ways to align Responsible AI with the speed of innovation. One approach is through the automation and simplification of AI governance processes. Another is the seamless integration of compliance activities into the overall AI governance program, facilitated by built-in frameworks and templates – like the ones embedded in the Lumenova AI platform.
Another avenue we endorse, and one that finds its place within the Lumenova AI platform as well, involves the automation of AI testing. This not only ensures robust testing, but also contributes to heightened process efficiency and productivity across the board.
This approach not only works towards preserving the reputation of a company, but also ensures regulatory compliance as AI evolves within the organization.
Myth 2: Responsible AI Is the AI Specialist’s Job
Contrary to the misconception that Responsible AI is solely the concern of AI specialists, the reality is that coding and programming alone won’t be enough for ensuring Responsible AI practices.
Business leaders and teams from diverse functions, not limited to the tech sector, must actively contribute to establishing robust AI governance frameworks and processes within their respective organizations.
Crafting effective AI governance is about giving the right stakeholders across the organization the tools and insights they need, and the right stakeholders are not limited to tech teams. They are:
- The enterprise’s risk, compliance, legal, and privacy teams, overseeing protocols within the organization.
- The technical specialists, including data scientists and ML engineers, who have access to model evaluation outputs that guide and enhance their work.
- And finally, the executive leaders who require in-depth visibility into emerging issues linked to strategic priorities or regulatory obligations.
All of these professionals should work together in order to transition Responsible AI from a theoretical concept to a tangible and measurable endeavor, one that can be firmly placed within individual ownership and tracked against a well defined set of Responsible AI principles.
Myth 3: Testing Models Just Before Deployment Is Enough
Many organizations typically follow the traditional MLOps lifecycle, initiating an additional step of Responsible AI activities, such as risk and compliance reviews, just before deploying a model to production.
This approach, however, proves to be tardy in the process, as substantial time and dollars have already been invested by this stage.
The more effective solution would be the integration of Responsible AI activities throughout the entire model lifecycle. This way, by transitioning from a reactive stance to a proactive one, AI risks can be identified and mitigated earlier in the process. This usually proves to be significantly less expensive than discovering them when the model is on the verge of deployment.
Myth 4: Addressing Responsible AI Challenges Solely Through a Tool-Based Approach Is Enough
While leveraging tools can enhance an organization’s implementation of a Responsible AI strategy, it’s essential to recognize that tools alone are not bulletproof.
The effective execution of MLOps and AI governance demands dedicated effort, discipline, and time from individuals across the organization who are now tasked with the challenge of finding the delicate balance between achieving operational efficiency and safeguarding against the increasing spectrum of AI risks.
From a broader perspective, while platforms such as Lumenova AI contribute to the transparency and trustworthiness of AI models, it’s essential to recognize that tools alone do not encompass the entirety of Responsible AI. Tools serve as aids for the effective execution of Responsible AI processes and principles defined within an organization.
In simpler terms, it boils down to humans to investigate why AI models reach certain decisions, to audit these systems and ask all the necessary questions to ensure they align well with organizational standards.
Let’s follow the example of fairness. As we discussed in one of our previous blog posts, fairness isn’t a one-size-fits-all concept – it can be defined in a myriad of ways, and sometimes these definitions don’t match each other. Individual fairness differs from group fairness, and what is fair may also be different based on use case.
While various tools can be employed to assess AI fairness, the ultimate responsibility lies with organizations, as they must firstly identify the definition that aligns with their values and suits a particular use case.
Final Words
Dispelling these Responsible AI myths will demand commitment and action from leaders across various departments within your organization.
It’s also crucial for businesses to acknowledge that Responsible AI is just one facet of the broader sustainability considerations surrounding AI initiatives. This involves ensuring the ongoing dependability of AI-driven processes in both their implementation and execution.
If the prospect of investing in Responsible AI seems daunting, consider the alternative: uncovering that your current AI models harbor biases against specific groups could lead to significant public backlash for your organization. Consequences may include legal actions from affected parties, regulatory fines and audits, and severe damage to your public reputation.
The good news is, embracing Responsible AI isn’t merely a moral imperative—it’s an achievable objective. In a world where AI is evolving at lightning speed, we’re excited about a future where ethics are an integral part of AI innovation.
Lumenova AI: AI Governance, Simplified
Take AI governance from challenge to competitive advantage with the only platform that integrates Responsible AI practices directly into your technical workflows.
Our platform follows a structured yet flexible workflow to help enterprises govern the entire AI lifecycle. Find out how it works by requesting a product demo today.