May 15, 2025
Can Your AI Be Hacked? What to Know About AI and Data Security
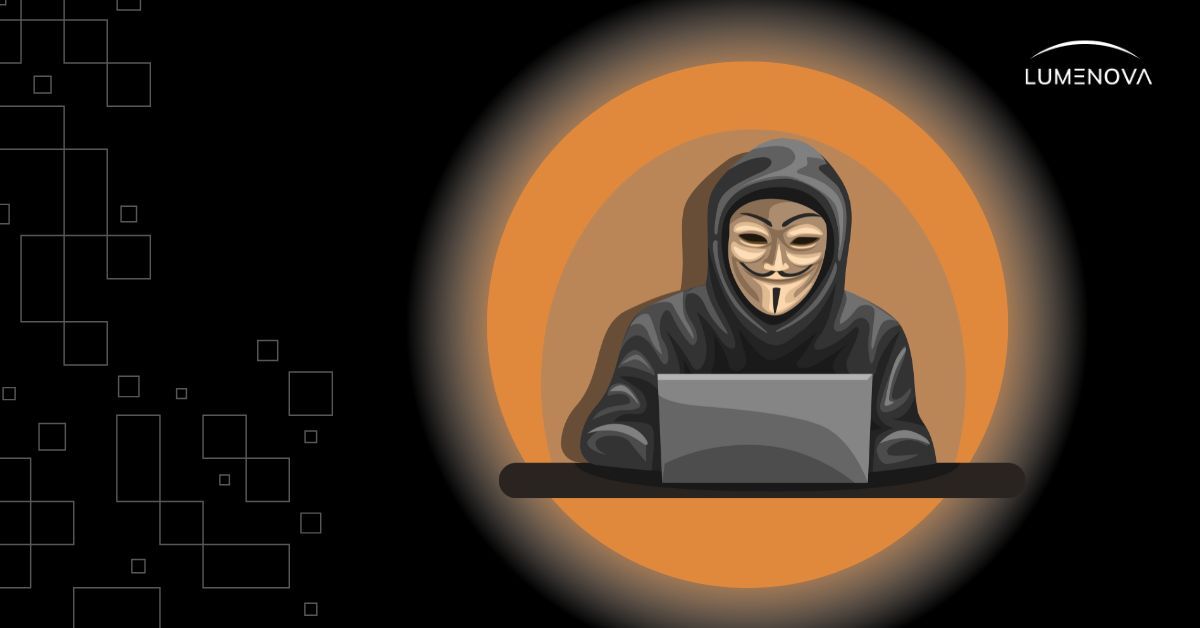
Contents
AI and data security must go hand-in-hand to protect your organization and manage your AI risk.
Artificial intelligence (AI) systems are advancing in sophistication by the day. Traditional machine learning models are evolving, generative AI is becoming widely popular in business, and AI agents are likely the next wave of innovation. As AI systems become more complex and dynamic, capable of taking in and learning from vast datasets and adapting in real time, they also become vulnerable to novel cyber threats. Attackers are finding new ways to infiltrate AI systems and corrupt training data, hijack model behavior in production, and otherwise exploit AI use.
In this post, we’ll break down how these attacks work, what your organization stands to lose, and—most importantly—what actionable steps your teams can take to protect your AI assets.
SEE ALSO: 3 Questions to Ask Before Purchasing an AI Data Governance Solution
How AI Can Be Hacked: 3 Key Attack Vectors
Adaptability is an attractive feature of artificial intelligence, and developers have been hard at work to create systems that learn from data in real-time. While this opens the door for AI to become more useful to businesses, it also has led to the emergence of a new category of cyber threat. Next, we’ve outlined three of the most critical attack vectors to understand and mitigate.
1. Adversarial Attacks
Hackers have begun to exploit the way AI systems continuously learn by injecting malicious or misleading information into the data they use to adapt. Known as adversarial attacks, the goal of these types of threats is typically to trick an AI model into making inaccurate predictions. In financial services, someone might modify transaction data just enough to bypass a bank’s fraud detection model.
Most dangerously, these small manipulations to AI systems are often not detected by human reviewers. An AI risk management platform that prioritizes security, like Lumenova AI does, can help your organization to catch these threats much more effectively than human review alone.
2. Data Poisoning
As the name suggests, data poisoning involves injecting corrupted data into an AI’s training pipeline. Unlike adversarial attacks which strike after an AI system is live by adding malicious data to the information it adapts with, data poisoning targets an AI model while it’s in its training phase. The goal of this type of attack, most commonly, is to corrupt the model’s learning process and cause long-term degradation of the model. In some cases, though, hackers will aim to create a backdoor access point into the model for themselves.
SEE ALSO: Types of Adversarial Attacks and How To Overcome Them
3. Model Inversion
Even without access to the original training data, attackers can exploit AI models through techniques like model inversion. The technique does not involve breaking into servers, but instead aims to exploit the AI model by asking carefully crafted questions that allow a threat actor to piece information together about its internal logic and/or training data. For financial institutions and insurers handling personally identifiable information (PII), this presents a significant data privacy risk and could result in non-compliance with regulations like GDPR or the EU AI Act.
To imagine an example of model inversion, picture a facial recognition system trained on employee ID photos. If that model is exposed, even indirectly, an attacker could repeatedly query it and gradually reconstruct approximate images of the people it was trained on. In highly sensitive fields like finance, healthcare, or biometrics, this is a privacy nightmare.
AI and Data Security Defense Strategies
Hackers are inventive, and have created ways to exploit AI systems at every stage of the AI lifecycle. To mitigate these threats, it’s essential to protect your system thoroughly with the following tactics (at minimum):
- Frequent model audits to assess for AI bias, model drift, and adversarial robustness.
- AI explainability tools to ensure decisions are transparent and defensible under scrutiny.
- Secure MLOps practices such as version control, access restrictions, and encrypted pipelines to keep vulnerabilities to a minimum.
- Real-time AI monitoring to detect input anomalies, data drift, and abnormal model behavior before damage occurs.
- Detailed documentation of model development, deployment, and testing processes can assist with identifying vulnerabilities quickly and efficiently.
- AI governance platforms like Lumenova AI make these defense strategies easy to implement and even enhance the performance of AI systems.
Protect Your Organization from AI Security Threats
AI systems are revolutionizing how companies operate, but they are also introducing new vulnerabilities that hackers can exploit. As we’ve seen, AI can be hacked in ways that go beyond traditional cybersecurity threats and can easily go undetected by human reviewers. These vulnerabilities put not only your data at risk, but also your compliance posture, customer trust, and competitive advantage.
To protect your organization, you need a responsible AI platform in place. Our free AI risk advisor can help you to assess your AI risk management strategy right now - or, if you’re ready to talk to someone about your AI governance needs, reach out tobook a consultation today.