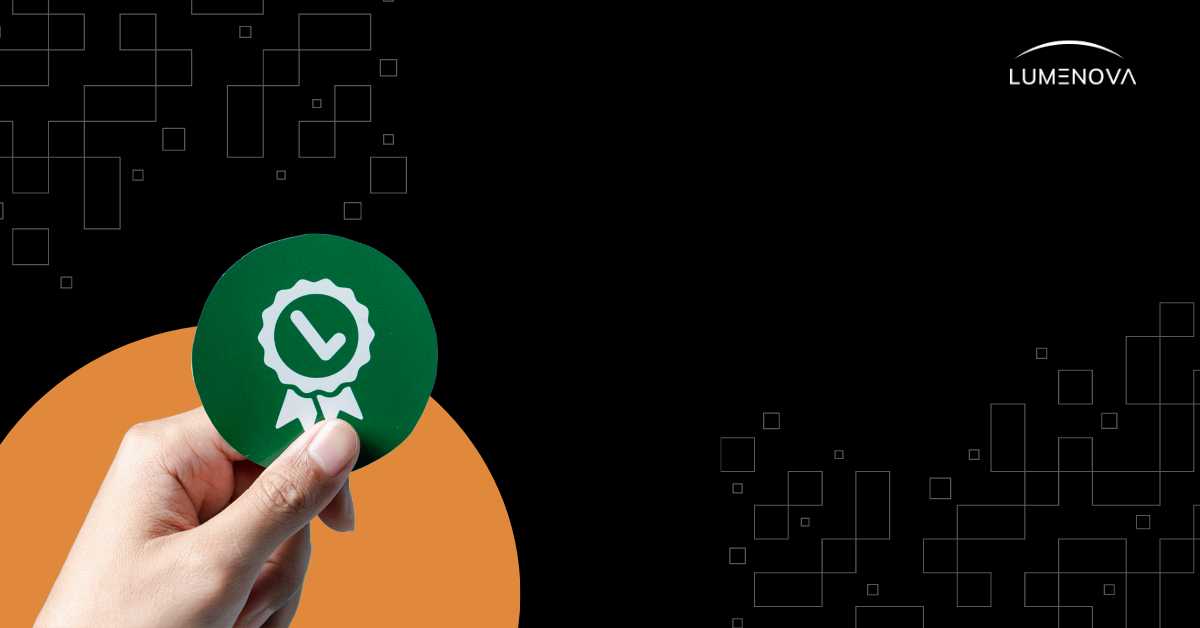
Contents
The importance of trustworthy and responsible AI (RAI) development and deployment is paramount to ensuring a safe and beneficial AI-driven future, particularly as organizations around the world, many of which are far from being AI natives, increasingly adopt AI systems. AI can do a lot of good, especially in a business setting. But, failing to account for the risks it might inspire in a customer-centric context—even when AI systems are leveraged internally—can significantly damage a company’s reputation, competitive edge, trustworthiness, and compliance with relevant business and regulatory requirements.
Determining how to navigate the complexities of the AI risk management landscape is a challenging feat, even for companies that possess a deep understanding of AI and the impacts it may generate. This is because AI is a multifaceted and rapidly evolving technology that’s useful across several business domains, from HR to marketing, while progressing at a speed that often precludes human comprehension—an established RAI integration strategy that was sufficient one month ago may no longer be sufficient now. Moreover, the multifaceted nature of AI doesn’t only concern the wide array of tasks it might be used for, but also the diversity of risks it inspires, ranging from the technical and organizational to the social and systemic.
The evident difficulties ingrained in AI risk management practices suggest that some kind of code of conduct, which lays the foundation for a shared AI risk management language, must be developed. Fortunately, we are now witnessing early attempts at AI risk management standardization, with ISO 42001 being the first global AI risk management standard. Unlike NIST’s AI Risk Management Framework, which serves as a flexible guide to AI risk management best practices, ISO 42001 actually sets concrete benchmarks (i.e., standards) for companies to follow—this is not to say that NIST’s framework isn’t a great resource. Currently, ISO 42001 is still voluntary, but its status as the first global AI risk management standard, coupled with its increasing popularity, suggests that it may soon become compulsory.
Throughout this post, we’ll break down the core components of the ISO 42001 standard, providing an overview of what companies who are considering implementation should know. We’ll begin with a discussion of the standard’s scope by reference to the organizational goals it suggests, followed by the series of specific requirements it proposes.
To keep up with the latest developments in the AI risk management landscape, follow Lumenova AI’s blog, where you can also explore content related to the most recent AI policy, generative AI, and RAI developments.
ISO 42001 Scope
The overall purpose of ISO 42001 is to illustrate concrete benchmarks for organizations to follow during AI development and integration, to ensure that these procedures align with RAI objectives, business objectives, and compliance requirements. More specifically, ISO 42001 proposes several noteworthy AI risk management goals for businesses to strive toward, categorized by the context of the organization, the needs of interested parties (usually users/consumers/key stakeholders), leadership responsibilities, and risk management. In terms of organizational context, these goals are:
- Understand the overall scope, purpose, and key actors involved in or relevant to AI development and integration within your organization.
- Understand the risks and hurdles your organization faces in terms of AI development and integration.
- Understand your organization’s AI compliance requirements.
- Understand the intended purpose of the AI system(s) your organization plans to implement, especially as it concerns RAI use/development, organizational objectives, and ongoing AI developments.
Considering the needs and expectations of interested parties in relation to AI integration and development is critical to developing a holistic understanding of AI risks, both internally and externally. In this respect, organizations should entertain the following questions:
- Who will be affected by the AI system we integrate, both internally and externally?
- What requirements and/or rights will those who are affected by or use the AI system(s) we integrate be subject to?
- How will our AI risk management strategy effectively address the above kinds of requirements?
Leadership, which concerns mainly the c-suite, is vital to RAI development and integration—if the c-suite possesses a limited understanding of AI risk management best practices, especially with regard to compliance requirements and business objectives, it will permeate every level of an organization. In this respect, ISO 42001 proposes that business leaders should:
- Ensure that RAI development, integration procedures, and objectives are closely aligned with organizational procedures and objectives and that AI systems are utilized in line with their intended purpose.
- Promote an organization-wide understanding of why compliance matters, and ensure that any AI system in use or under development is compliant with business and regulatory requirements.
- Assign roles and responsibilities to key personnel for AI system compliance and performance monitoring.
- Internally, provide enough resources for AI integration, which include support personnel, AI training, awareness, communication, and documentation procedures that foster an organizational culture that values the continual improvement, adaption, monitoring, competence, and transparency of AI systems and the key personnel working with them.
Leadership is also responsible for establishing AI policies to be implemented throughout the organization. AI policies should align with organizational objectives, enable AI benchmarking against AI objectives, include and identify compliance requirements, promote sustained revision and improvement of AI systems, and be documented, communicated, and easily accessible. Simply put, AI objectives should:
- Adhere to compliance and/or business requirements.
- Be measurable, monitored, communicated, updated, and documented.
On the other hand, the goal of AI risk management is to certify that an AI system is actually being leveraged in accordance with its intended purpose by addressing relevant risks and demonstrating a strong interest in continual improvement. However, to measure AI risks, organizations will need to establish risk criteria, mitigation and assessment procedures, and methods by which to understand possible AI-related impacts.
- Risk criteria should target four main areas: risk prioritization, assessment, mitigation, and impacts.
- AI risks and opportunities should be measured according to potential AI use cases, organizational context, and a system’s intended use, and any actions taken to address AI risks and opportunities should be documented—intended use concerns how a system is actually used whereas intended purpose concerns how a system should be used.
- For an AI risk management strategy to prove effective, it should:
- Identify which risk management strategies and options are appropriate.
- Foster an understanding of how to implement such strategies and options alongside necessary controls.
- Determine whether additional controls are required and how to implement them.
- Outline why certain controls were chosen and not others.
- Lay the groundwork for a concrete risk management strategy that targets alignment between AI and organizational objectives.
- Be documented, communicated, and accessible organization-wide.
- AI impact assessments should:
- Consider AI impacts on individuals, groups, and society at large.
- Consider the technical and societal context in which the system is deployed.
- Ensure that impact assessment results are clearly documented.
All of the organizational goals discussed in this section will inform the kinds of AI objectives an organization selects. However, to ensure they can realistically achieve their predetermined AI objectives, organizations must, at the very least:
- Understand what process is required to achieve AI objectives.
- Understand what resources will be required for this process to prove effective.
- Understand the timeline of this process.
- Understand how the success or failure of this process will be measured.
ISO 42001 Requirements
ISO 42001 proposes several requirements for organizations to follow when determining the scope, objectives, procedures, and mechanisms of their AI risk management strategy. To make these requirements more palatable for readers, we’ve categorized them in terms of generality followed by policy and risk-specific requirements. We note, however, that most of these requirements take the form of actions or controls (as referred to in Annex A)—things that organizations can do—and we suggest that readers keep this perspective in mind as we move forward.
General requirements
At a broad level, ISO 42001 identifies several important requirements for organizations to consider when developing and implementing their AI risk management strategy, which are described below:
- Document AI risk management strategies, so that they are easily available for use where relevant, and adequately protected in terms of confidentiality and integrity.
- Document and explain the reasoning behind the why and how underpinning the development of a particular AI system.
- Document and establish concrete procedures and mechanisms by which to verify and validate AI system performance, ensure that system design and development are aligned with organizational objectives and requirements, and establish a system deployment, monitoring, and operation plan.
- Identify and document the array of resources required for the successful management of an AI system throughout its lifecycle, to ensure a more holistic understanding of potential AI risks and impacts. Resources to consider include system components, data, relevant tools, as well as any necessary system, computing, and human resources. Organizations should pay especially close attention to computing resources and the role they play in enhancing or diminishing continual improvement initiatives.
- Document data resources across all relevant organizational domains, to confirm data quality, integrity, validity, and accuracy.
- Establish an internal and external mechanism by which individuals can report concerns related to the use or development of AI systems.
- Provide a mechanism by which users can request information on an AI system. This information should illustrate systems' intended purpose, human oversight mechanisms, system accuracy and performance data, how-to guides or any other relevant education materials regarding how to appropriately interact with the system, and any changes made to the system affecting its operation, among several other important characteristics.
- When determining how to monitor, measure, analyze, and evaluate AI system performance, an organization should:
- Understand which characteristics of system performance should be monitored and measured.
- Develop methods by which to ensure that system monitoring, measuring, analysis, and evaluation procedures produce consistent, accurate, and reliable results.
- Understand when monitoring and measuring system performance is necessary, and when the results from these procedures should be analyzed and evaluated.
- Document all evaluations of AI system performance.
- Administer regular internal audits targeting AI system compliance with business requirements and ISO provisions. Internal audit programs should:
- Have clearly articulated audit objectives that consider concrete criteria and the scope for each audit.
- Be impartial and objective, and results should be documented and communicated to key management personnel.
- Display tangible efforts aimed at the continual improvement of active AI systems and the policies surrounding them—AI system performance is not static.
- When AI systems are noncompliant with ISO, organizations should:
- Address the area of noncompliance and implement measures to resolve it in consideration of the impacts generated.
- Determine whether the initial cause of noncompliance can, from a pragmatic standpoint, be eliminated to prevent further issues.
- Evaluate whether the measures implemented to address the area of noncompliance have proved sufficient. If not, changes should be made as necessary.
- Document the measures taken to address noncompliance and their subsequent results.
Policy requirements
According to ISO 42001, internal AI policies should be documented, regularly reviewed, aligned with organizational objectives, and target the use or development of AI systems. In this respect, AI policies should be predicated on several characteristics, which are described below:
- Business strategy, values, culture, risk threshold, and risk environment.
- The risk profile of an AI system(s), its potential impacts, as well as business and legal requirements.
- Core RAI principles that determine how AI activities are carried out internally, as well as potential exceptions to these principles and procedures for addressing possible deviations. RAI principles to be aware of include:
- Fairness, accountability, transparency, explainability, reliability, safety, robustness and redundancy, privacy and security, and accessibility.
- Targeted sub-domains such as AI resources, assets, impact assessments, and development procedures.
- RAI strategies, which include mechanisms for human oversight and review, system performance monitoring, AI impacts reporting, and evaluation of whether the level of system autonomy appropriately corresponds with systems’ intended purpose and use context.
For AI risk management policies to prove effective, relevant roles and responsibilities must also be established across the following business domains and functions:
- Risk management, impact assessments, system development, and system performance procedures.
- Asset and resource management, as well as supplier relationships.
- Security, safety, privacy, and human oversight.
- Compliance with business and legal requirements.
- Data management throughout the data lifecycle.
Moreover, it’s essential that the information of key personnel involved in AI risk management procedures is documented to illustrate relevant competence levels, and any tools leveraged to manage an AI system must also be documented and evaluated for efficacy accordingly. But, it doesn’t stop there—organizations need to possess and demonstrate a solid technical understanding of the AI systems they’re deploying or implementing.
- Technical documentation on AI systems should include:
- A description of intended purpose and usage instructions.
- Any relevant technical assumptions related to system deployment and operation, system limitations, and system capabilities in relation to system operation.
- Technical documentation on the stages of the AI life cycle should include:
- Information on system architecture and design, as well as any relevant choices influencing these characteristics.
- Training data and data quality assurance measures/assumptions.
- Risk management activities, impact assessments, and any changes made to a system during operation.
- Any relevant or necessary verification and validation documentation.
All in all, organizations should define and establish measures by which to execute and uphold RAI objectives during AI system development and integration—these measures will likely take the form of internal policies, and reflect pre-established RAI objectives. RAI objectives should be designed to address the various lifecycle stages of the AI development and integration process, and the possible risks and benefits that arise throughout. In this respect, organizations should strive to reach the following AI objectives:
- Ensure the presence of mechanisms by which to hold key actors accountable for their use of AI systems.
- Build AI expertise by creating interdisciplinary teams of AI specialists.
- Validate and verify the data used for AI training to ensure consistent data availability and quality.
- Determine the scope of AI impacts on the environment.
- Establish preventive or corrective measures to ensure that AI systems don’t inadvertently perpetuate discrimination or unfair outcomes.
- Develop standardized procedures by which to update or repair an AI system as necessary.
- Maintain data confidentiality and security to protect sensitive data and/or vulnerable data subjects.
- Prevent and mitigate AI-driven threats to human health, safety, property, or the environment, and ensure that AI systems are operated securely.
- Promote and uphold transparency and explainability to ensure that AI systems design, intended purpose, function, and use are easily interpretable and understandable.
Risk requirements
Under ISO 42001, AI risk requirements primarily concern risk and impact assessments as well as risk and data management procedures. However, before diving into this set of requirements, we first outline what, according to ISO, are the most prevalent AI risks for organizations to feature on the risk management radar:
- Inconsistent AI system performance across novel situations or within changing environments—a lack of robustness and resilience.
- Lack of transparency and explainability.
- Excessive automation that compromises core RAI principles, such as safety or fairness.
- Data quality, especially during AI training phases.
- Potential hardware vulnerabilities or inadequacies.
- Failing to account for all possible risks that arise throughout the stages of the AI lifecycle.
- The degree of technology preparedness possessed by an organization at large.
An AI impact assessment should address core AI impacts, only be performed when necessary, possess a uniform structure, and be evaluated in accordance with key RAI principles. There are many possible AI-related impacts organizations could encounter, but at the very least, they should be aware of:
- Whether a system produces adverse legal effects or diminishes the opportunities available in an individual’s life.
- Whether a system threatens human well-being or fundamental rights, and which groups or individuals are most likely to be affected.
- Whether a system poses a societal risk or a risk to individuals or groups due to its intended purpose. Societal risks include those related to environmental sustainability, governance and economic processes, human health and safety, as well as social norms and culture.
On top of being aware of these AI impacts, organizations should also investigate the nature of potential impacts—whether they are positive or negative and at what scale they apply—the level of complexity of an AI system, and whether there exist pre-identified risks that can be easily addressed as well as the steps taken to mitigate them, the role of any key personnel involved in AI system management procedures, and who is responsible for administering the impact assessment as well as how it will be used. Nonetheless, understanding when an impact assessment is necessary depends on whether:
- Changes to the intended purpose of or context in which an AI system is leveraged occur.
- An AI system displays a particularly high level of complexity and/or autonomy.
- The data on which a system is trained is considered sensitive.
Though organizations may need to tweak the structure of their impact assessments to suit their particular organizational context or needs, impact assessments should still possess a uniform structure rooted in the following procedural components:
- Identification of potential impact sources, events, and outcomes.
- Analysis of the saliency and probability of potential impacts.
- Evaluation of the measures taken to address potential impacts.
- Management of established impacts via previously approved measures.
- Documentation, reporting, and communication of impact assessment results to relevant stakeholders.
Like impact assessments, risk assessments should also be performed when changes to the intended purpose or context in which an AI system operates occur. In this respect, risk assessments should:
- Align with AI compliance requirements and business objectives.
- Possess a uniform structure, such that assessment results are consistent and comparable over time.
- Pinpoint risks and opportunities that hinder or enhance an organization’s ability to achieve its pre-established AI objectives.
- Envision the potential impacts of AI risks and determine their probability and saliency.
- Measure identified AI risks against risk criteria and prioritize risks accordingly.
Organizations should record certain events relating to AI system performance, such as whether system performance is producing undesirable impacts, and determine at which stages of the AI lifecycle such recording will take place. Moreover, when a risk assessment reveals possible risks, they must be managed accordingly. If the risk management plan is insufficient, alternative approaches must be considered and incorporated to re-validate it, and all results must be documented.
As for data management, organizations should develop, establish, and execute a data management strategy targeting data privacy, security, accuracy, integrity, representativeness, as well as transparency and explainability, centering on the role data plays in AI system use and/or operation. Furthermore, this strategy should also consider how data is acquired, namely as it concerns the nature, quantity, and lineage of data required for AI training, the sources of said data and their characteristics, how prospective data was previously used, whether meta-data is being utilized, and what data-specific rights, such as IP, are relevant. For more granular guidance on data management procedures, we suggest that readers review ISO 42001 directly.
Conclusion
ISO 42001 sets the stage for a standardized approach to AI risk management, which could prove highly useful to many kinds of organizations, especially when coupled with AI risk management guides like the NIST AI Risk Management Framework. Nonetheless, when considering how best to structure and execute their AI risk management strategies, organizations should approach this process with care and flexibility—rapid advancement in the AI ecosystem and regulatory landscape will continue to reshape the way we think about AI risks and opportunities.
To maintain a relevant understanding of what constitutes best practices in the AI risk management sphere, we invite readers to follow Lumenova AI’s blog, where they can find additional in-depth information on the latest risk management standards and frameworks, AI policy developments, and progress in the fields of generative and RAI.
For readers who wish to take concrete steps toward RAI integration, development, or risk management, we suggest that you familiarize yourselves with Lumenova AI’s platform by booking a product demo today.