April 23, 2024
Beware the Pitfalls: Why Skimping on AI-Specific Risk Management Spells Trouble
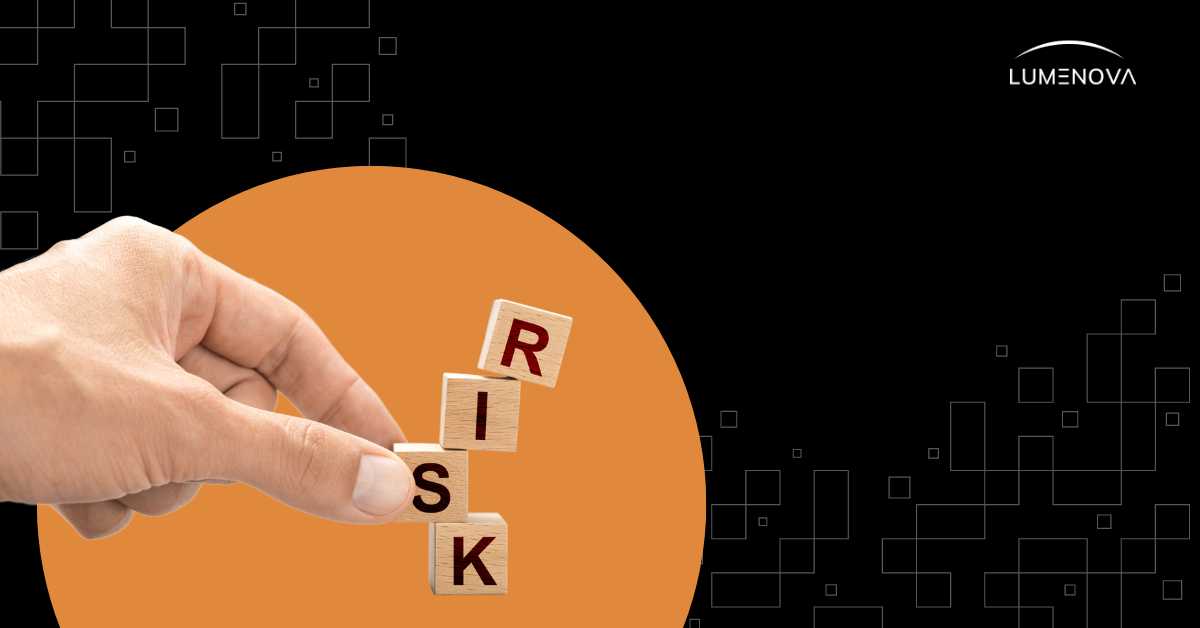
AI has slipped into the forefront of public consciousness, dominating conversations and headlines with its exponential growth. Amidst this surge in adoption, however, the truth is that many businesses still struggle to fully comprehend the entire spectrum of AI risk, which in turn makes the risk landscape even more sophisticated.
One of the major misconceptions related to AI risk management is that AI can be treated the same way as software. This flawed comparison leads organizations into a false sense of security, believing that their existing software risk management strategies will suffice for AI.
Yet, the truth is far from it, as AI stands apart from conventional software, rendering traditional risk management approaches inadequate in the face of its unique challenges.
Why Traditional AI Risk Management Does Not Work for AI
Looking into why traditional AI risk management falls short highlights its struggle to deal with the distinctive nature of AI. Unlike regular software, AI is always changing and adapting. This means the usual methods we use to manage software don’t quite fit when it comes to managing AI.
Beyond the obvious contrasts, there’s a unique social aspect to AI, since it’s the ongoing collaboration between machines and humans that truly amplifies AI’s capabilities. AI deployment and fine tuning involves a higher degree of human involvement compared to traditional software. In this case, we’re talking less about it being merely a new technology, and more about it powering intelligent processes.
That alone means that the paradigm in how organizations manage software capabilities will not fit into how they should manage AI capabilities.
The Landscape Of AI Risk is Multifaceted
There’s a wide array of AI risks that we need to keep in mind, and the conversation around identifying and managing them is constantly evolving.
Some key concerns include privacy breaches, biases in algorithms due to poor data quality, the rise of deepfakes, and issues related to copyright infringement, among others. This list isn’t exhaustive, but it gives us a glimpse into the diverse challenges we need to tackle with ongoing vigilance and proactive solutions.
Despite the media focusing more on ethical dilemmas, biases, or existential concerns like AI replacing jobs, the spectrum of AI risks extends beyond these topics. Often overlooked in the media discourse are risks that could significantly impact organizations, such as financial risks, risks to customer experience, and operational challenges. Effective AI risk management should incorporate both societal and organizational perspectives to ensure its responsible and beneficial integration.
Cybersecurity Considerations
Cybersecurity also poses different challenges for AI models compared to traditional software. Malicious actors actively trying to subvert AI tools led to the emergence of adversarial machine learning, which aims to systematically probe worst-case failure scenarios of ML models.
The vulnerabilities impacting AI can take various forms:
- Classical vulnerabilities, such as flaws in code or APIs
- New types of vulnerabilities, such as those specific to the supply chain - for example: tainted databases due to data injection.
Unlike code vulnerabilities that can be isolated and fixed, vulnerabilities in datasets are not as easily patched. This underscores the critical importance of AI risk management for organizations.
What the Future Looks Like
To protect against risk and malicious attacks, it’s essential for risk practitioners within enterprises to take swift action. However, particularly in the area where AI is deeply ingrained in processes and makes decisions autonomously, it’s vital to acknowledge that AI often operates at a scale and speed that’s impossible to manage manually.
This reality underscores the need for a proactive approach and risk practitioners should consider using intelligent tooling across their AI portfolio in order to create visibility and transparency.
In addition to this, in order to effectively align with today’s AI landscape and carry out their duties proficiently, risk teams may consider the following steps:
- Embrace AI: Understanding how AI influences daily operations is crucial for effective risk management. Experimenting with AI tools and techniques provides invaluable insights into its actual, day-to-day impact.
- Explore novel AI risk management approaches: Recognizing that AI differs from traditional software means exploring new strategies that can be tailored to its unique characteristics. Traditional AI risk frameworks fall short in addressing AI risk.
- Focus on business outcomes: While AI monitoring often centers on technical metrics, it’s essential to delve deeper into the business implications of AI usage. Shifting the focus from technical metrics to tangible business outcomes should help define an effective AI risk management strategy.
- Engage diverse stakeholders: Recognizing the multifaceted nature of AI risk requires involving a wide range of stakeholders beyond just technical teams. Incorporating input from legal, compliance, ethics, and diverse business units will ensure a more comprehensive understanding of potential AI risks, enhancing the effectiveness of risk management strategies.
- Stay informed on external developments: Continuous monitoring of technological advancements in AI is essential, and so is staying abreast of evolving methods for responsible AI implementation and ongoing developments in AI regulation. This way, risk practitioners can ensure their strategies remain adaptive and compliant with evolving standards and expectations.
How Lumenova AI Can Help
At Lumenova AI, we firmly advocate for the immense potential of AI, all the while understanding the importance of practicing responsible AI. Since the beginning, our mission has been to help enterprises like yours in automating, simplifying, and streamlining the entire AI risk management process.
With Lumenova AI you can:
- Launch governance initiatives
- Establish policies and frameworks
- Assess model performance
- Pinpoint potential risks and vulnerabilities
- Consistently monitor and report on discoveries
Request a demo today to see it in action.