Trusted AI
with One Platform
Automate, simplify, and streamline your end-to-end AI Risk Management process.
All your AI Risk Management needs.
One platform.
With Lumenova you can:
- Manage AI risks, build trust, and accelerate the adoption of AI across your entire organization.
- Gain real-time insights into the reasoning behind outcomes, improve and monitor AI performance.
- Leverage Trustworthy AI to promote enterprise-wide transparency and accountability.
- Design user-friendly procedures and policies that increase company- wide awareness of Al risk exposure and address compliance shortcomings.
Lead with trust in AI.
Risk & Compliance Frameworks
Purpose-built to manage the risk and compliance of AI models, Lumenova provides a library of pre-built, customizable AI frameworks based on major regulatory and industry standards.
- Detect and track AI risk with a 360 visibility on your AI models
- Ensure up-to-date compliance with regulations and industry standards
- Stay on top of AI regulations and take a proactive approach to managing AI risk and compliance
Technical Evaluation Engine
Our module enables you to perform the technical evaluation of your AI models based on 5 key risk verticals: Fairness, Explainability & Interpretability, Validity & Reliability, Security & Resilience, Data Integrity.
- Understand how your AI works to optimize, improve, and future proof your model
- Extensive technical tests integrated directly into the risk management process
- Choose from hundreds pre-developed test templates with configurable parameters
Monitoring & Improvement Platform
AI requires dynamic safeguards, but traditional risk management tools were not designed for systems that evolve beyond their initial development.
Lumenova enables you to break these barriers and continuously monitor and improve your AI models.
- Evaluate model performance over time and take a proactive stance to risk mitigation
- Keep an eye open for data, model, or framework drift and adjust when needed
- Avoid reputational damage or financial losses
Lumenova evaluates your AI model
based on 5 key risk verticals
Fairness
- Analyze your model's predictions to make sure they are not biased
- Measure and compare a multitude of fairness metrics across the intersection of sensitive attributes
- Evaluate fairness in a wide range of model types
Explainability & Interpretability
- Uncover how each individual input influences the model's decision-making process
- Gain insights into what your AI has actually learned during training
- Determine how consistent your model is in the way it uses features across different predictions
Validity & Reliability
- Measure predictive performance with a multitude of metrics, including Accuracy, Precision, and Recall
- Ensure your model's performance is consistent across the whole feature space without concealed weak spots
- Analyze the extent to which your model is affected by data distribution drift
Security & Resilience
- Identify potential model issues and weak spots
- Discover adversarial vulnerabilities which make the model unreasonably sensitive to changes in the input
- Check if your AI relies too heavily on only a few dominant features when making predictions
Data Integrity
- Uncover data quality issues, such as class unbalance, outliers, unusual distributions, missing data, data drift
- Assess whether data is impartial to sensitive attributes or if it contains biases that can translate into model unfairness
- Uncover mislabelled training and test data samples that can impact model performance
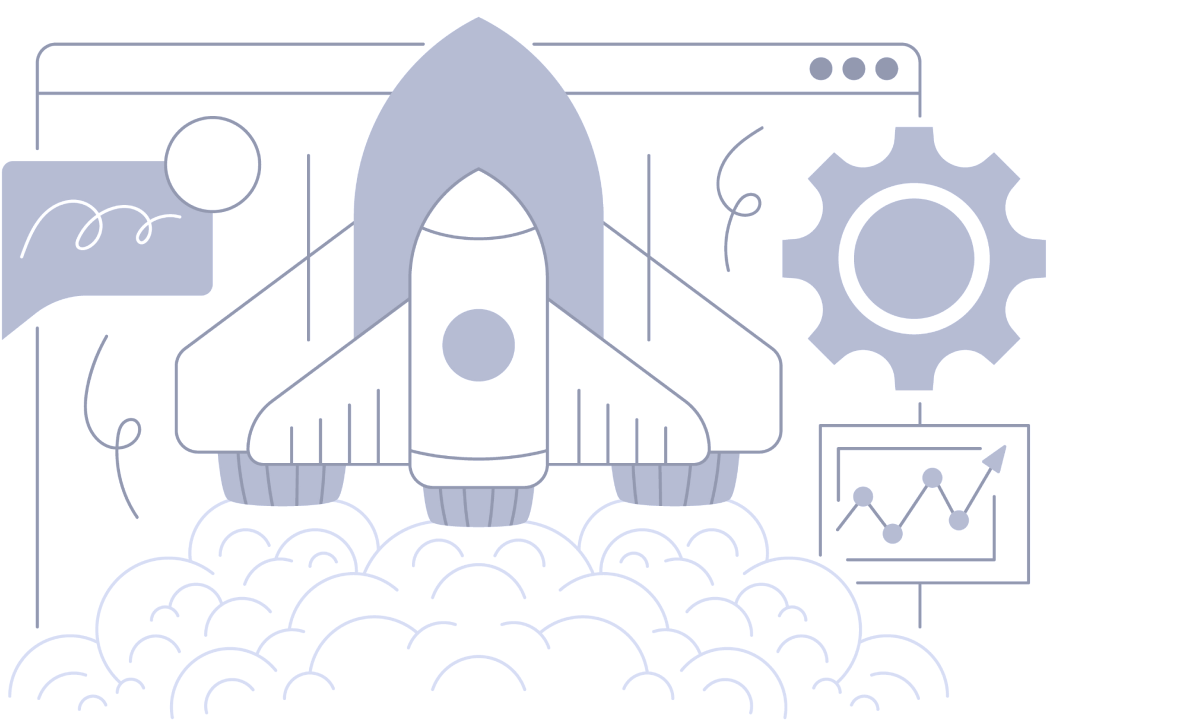
Fairness
Explainability & Interpretability
- Analyze your model's predictions to make sure they are not biased
- Uncover how each individual input influences the model's decision-making process
- Measure and compare a multitude of fairness metrics across the intersection of sensitive attributes
- Gain insights into what your AI has actually learned during training
- Evaluate fairness in a wide range of model types
- Determine how consistent your model is in the way it uses features across different predictions
Validity & Reliability
Security & Resilience
- Measure predictive performance with a multitude of metrics, including Accuracy, Precision, and Recall
- Identify potential model issues and weak spots
- Ensure your model's performance is consistent across the whole feature space without concealed weak spots
- Discover adversarial vulnerabilities which make the model unreasonably sensitive to changes in the input
- Analyze the extent to which your model is affected by data distribution drift
- Check if your AI relies too heavily on only a few dominant features when making predictions
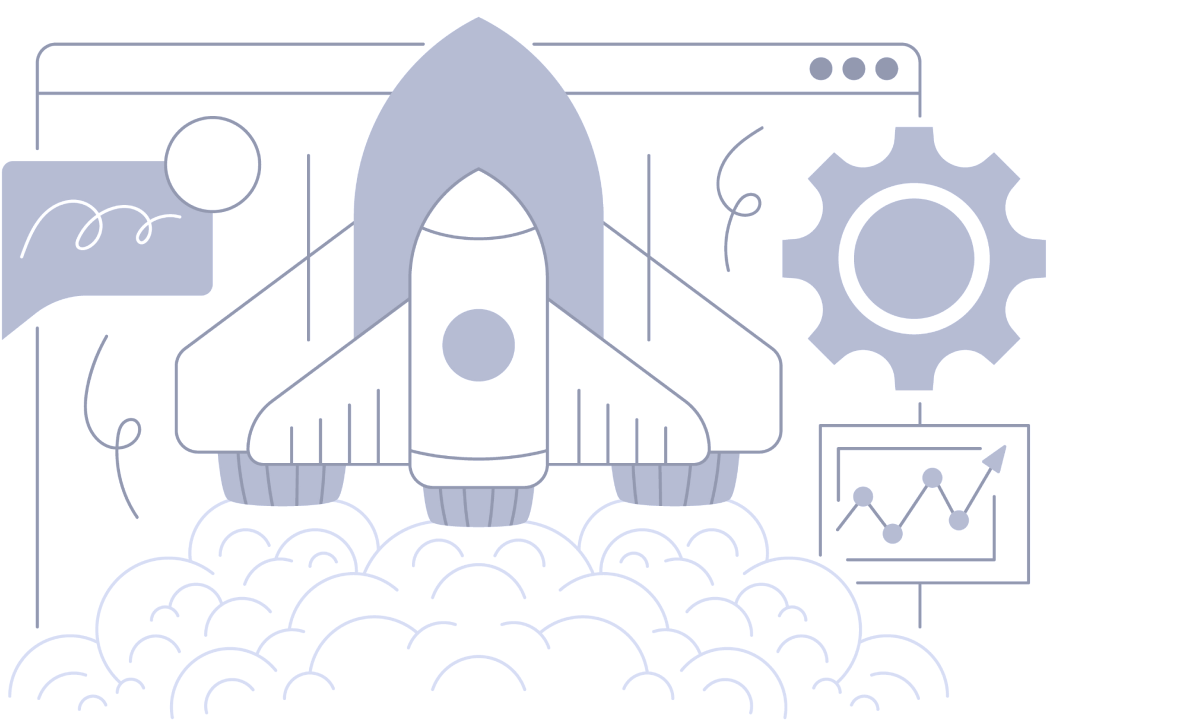
Data Integrity
- Uncover data quality issues, such as class unbalance, outliers, unusual distributions, missing data, data drift
- Assess whether data is impartial to sensitive attributes or if it contains biases that can translate into model unfairness
- Uncover mislabelled training and test data samples that can impact model performance
Gain visibility, agility, and efficiency with Lumenova
Laborious AI risk management tools
that slow IT down
Complex technical metrics
- difficult to align to business objectives
Risk management tools with slow-moving
safeguards that don't account
for AI
Concerns regarding AI security
and resilience
Easy-to-deploy and lightweight
platform that won't put strain on IT
Business-friendly insights
- easy to measure against business metrics
Purpose-built AI risk platform that
monitors AI and flags
risks
Full visibility into potential model
weak spots and adversarial vulnerabilities
Laborious AI risk management tools
that slow IT down
Complex technical metrics
- difficult to align to business objectives
Risk management tools with slow-moving
safeguards that don't account
for AI
Concerns regarding AI security
and resilience
that slow IT down
Complex technical metrics
- difficult to align to business objectives
safeguards that don't account
for AI
Concerns regarding AI security
and resilience
Easy-to-deploy and lightweight
platform that won't put strain on IT
Business-friendly insights
- easy to measure against business metrics
Purpose-built AI risk platform that
monitors AI and flags
risks
Full visibility into potential model
weak spots and adversarial vulnerabilities
platform that won't put strain on IT
Business-friendly insights
- easy to measure against business metrics
monitors AI and flags
risks
Full visibility into potential model
weak spots and adversarial vulnerabilities