May 21, 2025
The AI Revolution is Here: Understanding the Power of AI Agents
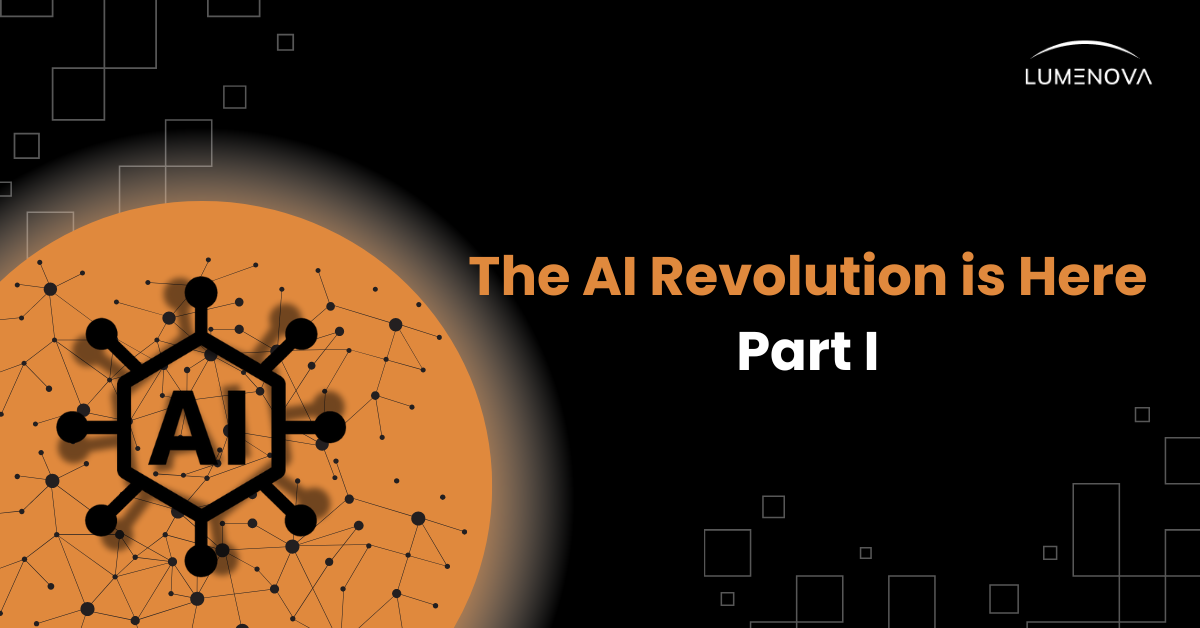
Contents
The next era of AI is on the brink of large-scale realization: the AI agent revolution. To make sense of this incoming era, however, we should reflect on the last couple of years to build a chronology of modern-day AI advancements that extends to the present day.
In late 2022, the world was introduced to ChatGPT. Some months later, Anthropic launched Claude, and Google released Gemini. Roughly one year after that, X brought Grok into the picture, cementing the first stage of the global Generative AI (GenAI) uprising.
During this period, the AI frontier was represented by general-purpose (GPAI) models — models with limited multi-modal capabilities that could perform various hard and soft tasks, from data analysis and code generation to creative writing and knowledge synthesis. Looking back on early GPAI, it’s almost funny to think how far these models have come, but even then, potential impacts bordered on groundbreaking:
- In June of 2023, McKinsey reported, in collaboration with the World Economic Forum, that GenAI could automate up to 70% of employees’ most time-intensive tasks. They also estimated it could contribute between $2.6 and $4.4 trillion to the global economy.
- In August of 2023, KPMG revealed that more than 80% of business leaders suspected GenAI investments would increase by over 50% by early to mid-2024. They also highlighted key adoption barriers, including infrastructural unpreparedness, use case identification, insufficient skilled talent, and regulatory uncertainty.
- In early 2024, The Economist showcased that almost 45% of businesses indicated strong investment interest in AI for advanced supply-chain automation. They further pointed out that among the 67% of the C-suite, approximately one quarter of AI investments would prioritize GenAI technology.
- In April of this year, the UN Trade and Development (UNCTAD) commission reported that by 2033, the global AI market could reach $4.8 trillion, which, if true, would dwarf 2023’s AI market ($189 billion) by a factor of 25.
Enter September 2024, marked by the release of the first models designed for advanced reasoning, namely OpenAI’s o1. The reasoners — today they include a growing family of models like o1-pro, o3, o4, Grok 3, DeepThink-R1, and Claude 3.7 Sonnet — established a new class of AI, defined by its ability to leverage chain-of-thought to “think” in real-time, solving increasingly complex problems, particularly across STEM-based fields. These models would set the stage for AI agents, though there are a few breakthroughs and challenges worth discussing first:
- A comprehensive 2024 study on OpenAI’s o1 revealed major advancements in core capabilities areas like analytical and logical reasoning, cross-domain competency, and dynamic problem-solving. Key findings included o1’s ability to adapt to novel scenarios, solve multi-step/layered problems, operationalize multi-domain PhD-level knowledge, and synthesize multi-disciplinary information.
- In December of 2024, OpenAI’s o3 (the high compute version) passed the ARC-AGI-1 benchmark with a score of 88%, which was eight percentage points higher than the average human score and a startling 56% higher than o1’s score (which was tested a few months earlier). Prior to o3, no frontier AI models had even come close to passing this gold-standard benchmark, and o3’s performance signified one of the most substantial, quantifiable leaps in machine intelligence in the modern AI era.
- On the second version of the benchmark — ARC-AGI-2 — which has a passing threshold of 60% and requires much more abstraction, OpenAI’s o4-mini-medium scored 41%, which researchers deemed promising. However, the team also noted some important challenges, namely declining accuracy rates across later responses and high-compute inefficiencies.
- Via our AI experiments, we’ve demonstrated that reasoners and GPAI models aren’t as “intelligent” as they’re made out to be. They still struggle across tasks or problems involving social, cultural, and moral intelligence, strategic ideation under uncertainty, multi-phase, evolutionary problem-solving, meta-analysis self-reflection, and self-verification, associative reasoning, ambiguity, contextual nuance, long-term planning, and causal understanding (in the absence of structure).
In any case, we’re here now. AI agents have arrived, there don’t seem to be any legitimate regulatory hurdles that will delay their deployment (in the US, at least), and funding continues to increase steadily — by the end of 2024, private, US-based AI investments exceeded $100 billion, and during just this year, EU-based VCs have allocated more than half a billion specifically to AI agent startups. Chinese AI investments are also accelerating rapidly, and in January 2025, the nation launched a state-subsidized AI fund, eclipsing over $8 billion.
- Note: Although AI regulation in the US remains immature and deeply fragmented, large-scale agentic AI deployment still hinges on infrastructure preparedness, which will represent one if not the most crucial barrier to scalable deployment. This also partly explains the Trump administration’s public-private partnership, the heavily funded Stargate Project, which aggressively aims to bolster AI infrastructure nationwide and maintain US AI dominance.
All this hype and investment signals confidence, and state-of-the-art offerings like OpenAI’s Operator, Anthropic’s AI Agent, and Google’s Vertex AI Agent Builder indicate that AI agents are being explicitly framed and marketed as the next AI frontier. But we’re not here to hype. We’re here to help you understand. So, what are AI agents, how do they differ from the AI we “know” already, and where could they offer pragmatic value?
An Introduction to AI Agents: What Are They?
Understanding AI agents independently is insufficient. These systems are only one part of the AI family, and to see what makes them unique, we need to understand their relatives, which include:
- GPAI: Per our original definition, models that possess a diverse task repertoire, performing both soft and hard tasks across a range of functions and application domains (e.g., ChatGPT, Claude, Grok).
- Multi-Modal AI: Models that can process and generate information across multiple modalities, including audio, visual, code, and text-based data (e.g., ChatGPT, Claude, Grok).
- Reasoners: Models that can reason in real-time, “thinking” through problems strategically while reflecting on and refining their problem-solving approach (e.g., o4, DeepThink-R1, Claude 3.7 Sonnet).
- Image/Video Generators: Models that can generate sophisticated, and oftentimes hyperrealistic images or videos in a wealth of different styles or formats (e.g., Stable Diffusion, Midjourney, Sora).
- AI Assistants/Chatbots: Models that function as helpful language-based chatbots, frequently (although not always) fine-tuned for specific tasks like customer service or internal communication.
- Voice AI: Models that support voice interaction, responding to and engaging with users in their spoken language (e.g., ChatGPT “Voice” mode).
- Decision-Making AI: Systems explicitly designed and used for decision-making assistance, augmentation, or automation, typically within critical domains like healthcare, finance, and employment.
- Recommender/Personalization AI: Algorithms that learn from user behavior to provide personalized recommendations (e.g., content curation, product recommendation) for content, products, services, and even learning interventions (e.g., AI “tutors”).
- Edge AI: Systems integrated in edge devices (e.g., smartphones, autonomous vehicles, IoT sensors, smart home assistants, etc.) that usually process and store data locally but can support cloud connectivity (e.g., Amazon’s Alexa, Google’s Nest thermostat).
- Biometric AI: Systems that ingest and process highly sensitive data (e.g., personal identifiers, financial information, health history, etc.), often used for user/consumer profiling, behavioral analysis, or hyper-personalization (e.g., psychometric profiling, surveillance).
- Diagnostic AI: Systems purpose-built for diagnostic procedures (e.g., cancer or pre-diabetes screening, supply-chain resilience, manufacturing efficiency, etc.), popularized in healthcare use contexts, but also leveraged in supply-chain automation and large-scale manufacturing.
- Safety AI: Systems that function as safety components of critical physical and digital infrastructures (e.g., emergency response, traffic control, disaster prediction).
- Risk Assessment AI: Systems, models, or platforms used to assess risks in other AI and machine learning technologies, for instance, Lumenova’s Responsible AI (RAI) platform.
- Governance AI: Systems that are integrated in and used for processes involving judicial, democratic, migration, asylum, and border control (e.g., predicting the rate of recidivism for convicted felons).
To be clear, these different AI “types” aren’t mutually exclusive (e.g., multi-modal GPAI or reasoners), and the same applies to AI agents (e.g., an AI agent powered by a reasoner that functions as an AI assistant). However, “AI agent” is a blanket term that describes an AI subclass, which can be further divided into four non-mutually exclusive categories:
-
Autonomous Agents: While fully autonomous agents aren’t yet operational, such agents are characterized by their capacity for independent action and decision-making complexity. These include:
a) Theory-of-Mind (ToM) Agents: Agents capable of inferring, comprehending, predicting, or responding to the emotional states, intentions, and beliefs of other humans.
b) Reactive Agents: Agents that don’t possess long-term memory or knowledge of previous interactions, responding exclusively to current inputs.
c) Limited Memory Agents: Agents that inform their current actions and decisions by relying on short-term historical data and extended knowledge across previous interactions.
-
Task-Based Agents: Agents purpose-built to execute specific tasks or functions, subsequently classified by reference to the tasks and functions they perform. These include:
a) Collaborative Agents: Agents that work together with other agents or systems to accomplish or perform complex goals and behaviors.
b) Assistants: Agents that function as semi-autonomous personal assistants, performing tasks like meeting scheduling and workflow optimization, tailoring their responses and behaviors to individual users.
c) Physical/Robotic Agents: Agents integrated with physical systems to enable dynamic interactions like object manipulation and spatial navigation, within real-world environments (e.g., Boston Dynamics’ Spot, Tesla’s Optimus).
d) Autonomous Software Agents: Agents that operate with limited human intervention and oversight to independently orchestrate and complete multi-step software tasks and workflows.
e) Conversational Agents: Agents that utilize conversational interfaces to communicate with users in natural language and complete semi-autonomous tasks on their behalf, according to their instructions. These differ from traditional chatbots because they can perform certain actions (e.g., a web search).
-
Intelligence Agents: Agents defined and differentiated by their superior cognitive capabilities, like multi-step problem solving, dynamic adaptation, or long-term planning. These include:
a) Narrow or “Weak” AI (Procedural AI): Agents designed to perform one or several procedural tasks with average human-level proficiency.
b) General or “Strong” AI (AGI): Agents capable of performing any conceivable cognitive task with average human-level proficiency.
c) Superintelligence (ASI): Agents that can perform any conceivable cognitive task with super-human proficiency, and make novel, likely incomprehensible scientific and philosophical discoveries.
d) Note: While we may be inching our way toward AGI, both AGI and ASI remain theoretical.
-
Domain-Specific Agents: Agents designed for certain application domains like healthcare, supply chain logistics, loan assessment, and manufacturing. Examples include:
a) Healthcare AI: An agent tasked with compiling patient diagnostics into a comprehensive report that includes treatment intervention suggestions for doctors.
b) Financial AI: An agent tasked with managing and optimizing an entire investment portfolio.
c) Education AI: An agent that works as a personalized tutor, crafting individualized lesson plans tailored to students’ interests and learning abilities.
d) Supply Chain AI: An agent that forecasts supply chain risks and predicts shortages, making semi-autonomous decisions based on pertinent constraints and optimization goals.
e) Infrastructure AI: An agent that autonomously triggers alerts for emergency or crisis events, sending dispatch notifications and event details to relevant response personnel.
If you’re starting to see some overlap between AI agents and their relatives, you’re on the right track, especially for building a holistic understanding of the AI frontier. Nonetheless, for those who are more technically inclined, we can also characterize AI agents by their fundamental properties and functional types. We’ll start with types:
- Single-Agent Systems: A single agent that acts independently within a single operational environment, like an underwater drone used to chart unexplored deep-sea areas.
- Multi-Agent Systems (MAS): A system composed of multiple intelligent agents, each with a specialized skillset, that dynamically collaborate and learn alongside each other to achieve complex goals.
- Note: In MAS, multi-agent cooperation isn’t a guarantee; it’s a desirable outcome. If you’re interested in learning more about this topic, click here.
- Hierarchical Agents: A multi-agent system in which agents operate independently as various hierarchical layers, where agents in higher layers assume strategic responsibilities while agents in lower layers perform tactical or operational tasks.
- Goal-Based Agents: Agents that determine which actions to pursue based on the optimization of a specific goal, for example, an agent that optimizes a shipping route based on weather predictions, ocean currents, and potential obstacles.
- Model-Based Agents: Agents that can build and maintain an internal model of their operational environment, to understand environmental dynamics and infer which actions are best. Returning to the deep-sea drone, imagine that it doesn’t “randomly” explore, but instead, actively chooses which areas are worth exploring based on relevant environmental factors (e.g., deep-sea currents) and the goals assigned to it.
- Simple Reflex Agents: The most rudimentary in the AI agent family, these agents follow specific, conditional rule-action structures and respond only to current information (e.g., a smart thermostat that turns on the AC when internal temperature rises above a certain threshold).
- Learning Agents: Agents that learn from their environment and users, via direct or indirect feedback, and improve their performance accordingly (e.g., a content curation algorithm that regularly updates content suggestions in response to subtle shifts in user preferences).
- Background Agents: Agents that don’t directly interact with users or their operational environment, performing passive functions (e.g., monitoring, data collection) “behind the scenes.”
- Surface Agents: The opposite of background agents — agents that directly interact with users or their environment (e.g., a simple chatbot).
Note: As AI agents advance, it’s possible that certain AI technologies once deemed “agentic” could lose their AI agent status due to agentic AI thresholds or criteria becoming steadily more difficult to fulfill as we update our understanding of AI agents. Consider a practical example: passing the Turing Test used to be a hallmark of AGI, however, virtually any present-day frontier AI model would easily pass this test, and yet we still don’t classify these models as AGI, because the Turing Test was a poor measure.
While there’s still debate around the core properties a system would need to possess to be deemed agentic, modern AI agents, when stripped to their essence, should exhibit the following capabilities:
- Goal-Directedness: The ability to take specific actions in the pursuit of specific goals.
- Independent Action: The ability to act independently or semi-independently with limited human oversight, guidance, and/or intervention.
- Complex Planning: The ability to develop and orchestrate multi-step/layered planning structures, usually with a long-term focus.
- Learning: The ability to learn from direct and indirect environmental and user feedback, as well as interactions with other systems or agents.
- Dynamic Adaptation: The ability to meaningfully engage with and adapt to novel problem-solving scenarios or environments not captured in training data.
- Explainability: The ability to transparently and coherently explain, typically via natural language, the reasoning motivating certain decisions, actions, or outputs.
- Self-Assessment: The ability to periodically self-assess and validate performance, particularly for accuracy and truthfulness, without human input.
- Uncertainty: The ability to gauge and evaluate decisions or actions that could inspire uncertain downstream impacts, and communicate confidence levels to human overseers responsible for high-impact decision/action approval.
- Cooperation: The ability to cooperate with other humans or agents to achieve complex goals or behaviors.
- Real-World Impacts: The ability to make decisions or take actions that drive real-world consequences.
We’ve now provided a comprehensive overview of AI agents, and at this point, we encourage readers to take a break and reflect on all the information we’ve presented. In doing so, we encourage you to ask yourself the following questions:
- What does the term “AI agent” mean to you, and does it change how you think about your professional growth and future?
- If you were asked to brainstorm three real-world AI agent use cases, what would they be and why?
- Do you believe the AI agent “hype” is warranted, and if not, what are your concerns or reservations?
- If you were asked to determine whether three frontier models — GPT-4o, Claude 3.7 Sonnet, and o4 — are agentic, what conclusion would you draw and why?
- Without reading up on it, what might some of the key benefits and risks of AI agents be?
- If you could envision AI agents ten years from now, what would they look like and what role might they play in an average user’s daily life?
AI Agents: Pragmatic Value or Hyped Up Nonsense?
We’ve got one question left to answer: where are AI agents delivering pragmatic value? Let’s start with some stats:
- According to a Grand View Research report, the AI agent market could reach over $7 billion by the end of this year, and by 2030, AI agents could contribute more than $50 billion in global revenue.
- In a separate international survey by Cloudera, 96% of respondents indicated interest in expanding their AI agent procurements over the next year, while over 50% strive for enterprise-scale integration. Moreover, there’s a fairly even split (66% vs. 60%) between organizations’ deployment preferences, with the majority leveraging internal digital infrastructures and the minority using embedded capabilities in existing applications.
- The same report further reveals key industries employing agentic AI, namely finance, insurance, manufacturing, telecommunications, and healthcare. Popular applications include fraud detection, security monitoring, diagnostics, customer support, and process and supply chain automation (among others).
- Across key industries, AI agent automation and productivity potential are high:
- Manufacturing: AI-powered predictive maintenance tools have diminished manufacturing downtimes by 40%, and in 2024, over 70% of manufacturers adopted AI.
- Healthcare: AI agents are dramatically reducing healthcare inefficiencies, automating almost 90% of clinical documentation tasks. By the end of this year, it’s expected that at least 9 out of 10 hospitals globally will pursue agentic AI solutions and integration.
- Finance: By 2035, AI agents could facilitate a nearly 40% increase in financial institutions’ profitability, and such institutions are forecast to account for 20% of global AI spending between now and 2028.
- Marketing: Almost 90% of marketing professionals use agentic AI regularly, with over half leveraging other AI tools, in addition to AI agents. Content generation represents a leading use case.
- Retail: AI agents are emerging as a powerful asset for driving personalized e-commerce services, with roughly 70% of retailers indicating agent-driven revenue gains.
- According to Zendesk, an AI Agent-as-a-Service (AaaS) platform, employee attitudes toward AI are deeply positive, with a stark majority (83%) highlighting AI’s decision-making potential as a core benefit. Similarly, 8 out of 10 employees claim AI agents have enhanced their work significantly.
- Major consulting firms, notably KPMG and Accenture, have already begun integrating AI agents into their operations, focusing on automation potential. Meanwhile, PWC predicts that AI agents will increase workplace productivity by over 50%, while Deloitte anticipates that a quarter of GenAI-enabled enterprises will deploy some form of AI agent this year, with adoption rates expected to reach 50% by 2027.
AI agent stats are expanding rapidly, but suffice to say, the ones we’ve illustrated here paint a solid picture of the landscape. Nonetheless, we encourage readers to interpret these trends with a grain of salt, particularly since most AI statistics come from organizations with a vested interest in streamlining AI adoption and procurement efforts (usually for profitability’s sake). So, returning to our initial question and reframing it slightly: do AI agents offer more value than “traditional” GenAI tools and technologies? Or, in other words, are AI agents imperative to unlocking the next stage of AI potential?
To answer this question, we’ve fabricated four hypothetical, real-world AI use cases across four distinct industries. We present two versions of each use case, the first of which relies on traditional GenAI, whereas the second integrates AI agents.
Use Case 1: Healthcare
Description: Automation of Clinical Documentation Processes.
Version 1: A hospital deploys a GenAI platform that seamlessly integrates with their clinical documentation systems, where doctors record patient-provider interactions. The platform works by analyzing and aggregating interaction data to generate coherent, easy-to-parse clinical summaries.
Positive Impacts:
- Data entry times decrease by almost 45%.
- The rate of human error decreases across manual entry tasks.
- Accelerates workflows significantly, providing some doctors with an extra 90 “free” minutes daily.
Negative Impacts:
- AI doesn’t reliably interpret nuanced medical judgements, context, or jargon.
- Human oversight remains crucial due to difficulties with evaluating accuracy autonomously.
- “Messy” patient records elevate ethical and compliance risks.
Version 2: A hospital deploys an AI agent, tasked not only with summarizing clinical interaction data but also with cross-referencing interaction data with previous medical records to provide tailored recommendations for potential treatment interventions.
Positive Impacts:
- Enhances patient safety by identifying medical incongruencies and discrepancies.
- Updates patient records, provides follow-ups, and generates alerts autonomously.
- Reduces data entry times and human error rates by roughly 80%.
Negative Impacts:
- System complexity makes for costly deployment, maintenance, and updates.
- Agent-driven decision-making requires continuous monitoring and strong, dynamic governance.
- Broader data access significantly increases privacy risks and introduces HIPAA-related compliance concerns.
Use Case 2: Education
Description: Adaptive Learning and Student Support.
Version 1: A high school is piloting a new GenAI application designed to provide personalized learning recommendations to teachers based on students’ performance across previous study materials and assessments.
Positive Impacts:
- Student engagement increases by 25%.
- Learning difficulties in different students are identified more efficiently by teachers.
- Some learning experiences can be personalized once performance changes are revealed.
Negative Impacts:
- Reinforces the notion that performance is all that matters in education, reducing students to their assignments and test scores.
- Personalized learning recommendations are static and can only be updated when new performance data is available.
- Teachers’ workload increases: they must figure out how to interpret performance data and use it to curate personalized learning plans.
Version 2: A high school assigns an individual AI agent to each of its students. This agent is designed to continuously monitor students’ learning progression, style, and performance, crafting a dynamic, student-specific learning profile. As AI agents update learning profiles, they also modify learning modules, provide interactive tutoring, and notify educators when direct intervention is necessary.
Positive Impacts:
- Student engagement rates increase by 45%.
- Via automated identification and intervention recommendations, teacher workload is reduced by 30%.
- Predicts potential learning difficulties, enabling proactive intervention.
Negative Impacts:
- Can’t capture outliers (e.g., students with specific learning disabilities), allowing them to fall behind.
- Parents raise serious privacy concerns regarding their children’s data, namely how it’s accessed, processed, protected, and stored.
- Teachers require extensive, hands-on training (and retraining when systems are updated) to foster effective use.
Use Case 3: Transportation
Description: Optimizing Routes and Fleet Management.
Version 1: A ground-shipping company wants to streamline and enhance its delivery process, attempting to expedite shipments to intended recipients. Via a custom-built GenAI tool that integrates with drivers’ smartphones and GPS, potential routes are analyzed according to historical traffic and route efficiency data, after which an optimized route is recommended.
Positive Impacts:
- Delivery punctuality gains are almost 20% higher.
- Fuel use and operational costs drop by more than 18%.
- Diminishes planning overhead for fleet management.
Negative Impacts:
- Inability to capture real-time events relevant to route optimization.
- Route optimization schedules are refreshed daily, but not updated throughout the day.
- Some drivers don’t perceive the gains afforded by the tool.
Version 2: An innovation-focused ground-shipping company deploys a team of identical AI agents, each tasked with managing their own shipping fleet. The AI agents analyze real-time traffic data, dynamically optimizing driver routes, coordinating different fleet members, and interacting with external traffic management systems to anticipate and manage delays.
Positive Impacts:
- System benefits are obvious to most drivers: delivery punctuality increases by 44% while fuel costs drop by 32%.
- Drivers and fleet managers can track real-time route optimization and fleet coordination activities.
- Due to semi-autonomous fleet management, fleet managers’ workload is reduced by 35%.
Negative Impacts:
- Some drivers don’t “trust” the system and prefer relying on traditional navigation systems like GPS.
- Most drivers heavily depend on the system, raising overreliance concerns should an AI failure occur.
- Integration complexity is very high: deploying the system is labor-intensive and costly.
Use Case 4: Finance
Description: Risk Assessment and Fraud Detection.
Version 1: A bank leverages GenAI to review historical transaction data and assess whether certain transaction patterns are indicative of fraud. When a fraudulent risk is identified, the system sends an automated alert to human reviewers.
Positive Impacts:
- Accuracy rates are high, with 80% of known fraudulent risks getting identified.
- Compliance teams see a significant reduction in manual workload.
- Time-to-action for fraudulent transactions is accelerated.
Negative Impacts:
- False positives are a clear problem: around 15% to 18% of flagged transactions aren’t fraudulent.
- The system exhibits limited adaptability, requiring extensive retraining to capture new risks.
- The system can’t provide explainable insights into its decision-making processes.
Version 2: A bank deploys a collaborative team of AI agents, each provided with a set of unique responsibilities, namely real-time transaction monitoring, autonomous intervention, and coordination with external financial systems. The agents all optimize for one goal: identifying fraudulent risks before they materialize. Across processes, agents aggregate the insights they obtain and learn from previous experiences.
Positive Impacts:
- Extremely high accuracy rate. Over 90% of fraudulent risks are correctly identified.
- Adaptivity enables the reduction of false positives to 7%.
- Can autonomously freeze potentially fraudulent transactions in real-time.
- Provides real-time insights into decision-making logic to enable transparency.
Negative Impacts:
- False Positives: Customer dissatisfaction rates increase due to agents freezing some legitimate transactions.
- If agents are compromised, system collapse may be imminent, driving a catastrophic AI failure.
- Intersystem dynamics dramatically complexify human oversight and monitoring practices.
Bottom Line: AI agents aren’t hyped up nonsense. However, they might not be for everyone, and most importantly, they represent one part of the AI frontier, not all of it. Think of it this way: if GenAI or even traditional machine learning can solve your problem at a lower cost than AI agents, then there’s no need to overcomplexify. Your AI ROI isn’t defined by innovation potential. It’s defined by practical constraints and the ability to build real-world solutions to the challenges you face today.
If you enjoy our content, please consider following Lumenova’s blog, where we explore a wealth of topics from AI governance and risk management to AI innovation, literacy, and strategy. For readers interested in longer-form, complex discussions, consider checking out our “Deep Dive” series and AI experiments, where we run weekly capabilities tests with frontier AI models.
On the other hand, if you’ve already initiated your AI governance journey, regardless of your maturity levels, we invite you to check out Lumenova’s RAI platform and book a product demo today. If this is something you’re interested in, you may also want to consider taking a look at our AI Policy Analyzer and Risk Advisor.