May 22, 2025
AI Governance Frameworks Explained: Comparing NIST RMF, EU AI Act, and Internal Approaches
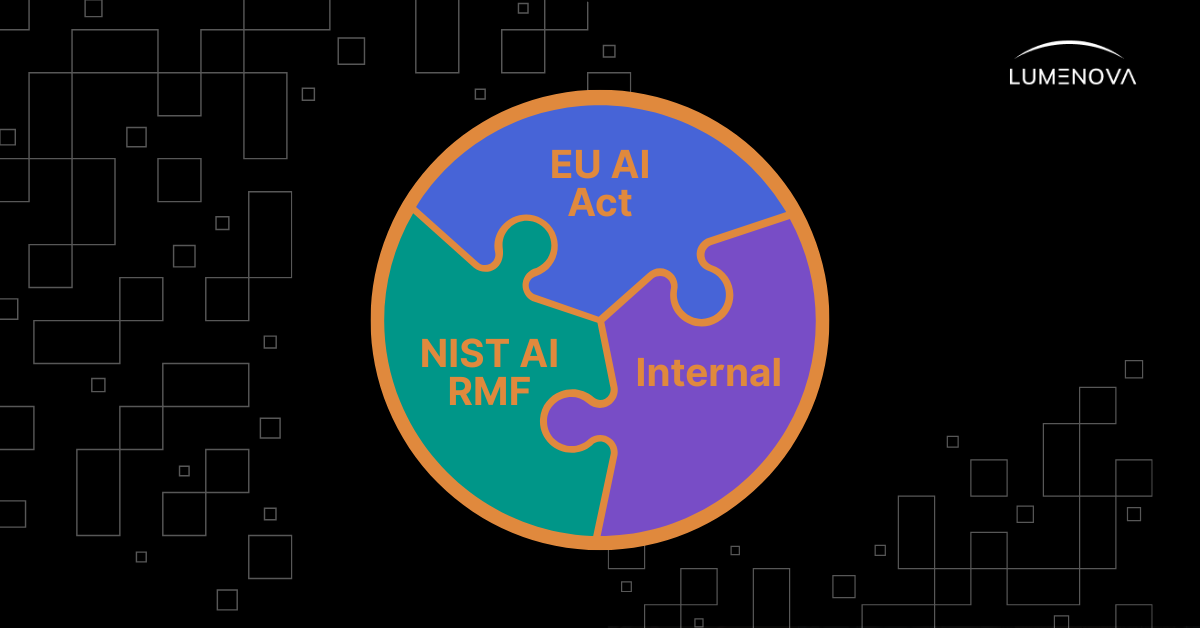
Contents
Following 2024’s surge in AI adoption, organizations are now focusing on governance and extracting value from Generative AI (GenAI). Nonetheless, while 78% use some AI, only 21% have fundamentally redesigned workflows due to GenAI (McKinsey). This disparity underscores the critical need for AI governance to mitigate risks like bias, loss of privacy, security threats, trust erosion, and legal fines. For companies that have AI in the house but just parked somewhere and not governed yet, this article explores key AI governance frameworks comparatively: the NIST AI Risk Management Framework, the EU AI Act, and internal company initiatives.
Key External AI Governance Frameworks
The NIST AI Risk Management Framework (NIST AI RMF)
The NIST AI RMF is a voluntary framework developed by the U.S. National Institute of Standards and Technology in 2023. Through its structured and measurable guidelines for AI risk management, it aims to provide a flexible approach for managing AI risks across the entire AI lifecycle. Core principles are trustworthiness, safety, security, resilience, explainability, interpretability, privacy, fairness, accountability, and social responsibility, and the framework proposes four core functions:
- Govern: Establishing a culture of risk management, defining roles and responsibilities, and implementing policies.
- Map: Identifying and understanding AI risks within specific contexts and throughout the AI lifecycle.
- Measure: Assessing, analyzing, and tracking identified risks.
- Manage: Prioritizing risks and taking action to mitigate them.
The NIST AI RMF is relevant for a variety of stakeholders including: organizations that develop, deploy and/or use AI systems, specific roles within those organizations (AI system designers, developers, data scientists, data engineers, risk managers, compliance officers, legal counsel, business leaders) and the broader societal ecosystem in which the AI systems operate.
Designed as a voluntary and flexible resource for anyone involved in or affected by AI systems across various sectors and use cases, the NIST AI RMF establishes a trustworthy AI foundation, the advantages of which are significant, especially for improving risk management, fostering responsible innovation, and maintaining an upstanding reputation.
SEE ALSO: Lumenova AI Joins NIST AI’s Safety Institute Consortium
The EU AI Act
We’re now leaving the realm of voluntary frameworks and entering that of enforceable regulation. The EU AI Act is a landmark, risk-centric regulation that the European Union signed into law in 2024. One of its key objectives is to provide guidelines for preserving the safety and fundamental rights of individuals while promoting EU-based AI innovation.
The act leverages a tiered risk classification framework, defining the following AI risk categories:
- Prohibited AI Systems: AI systems that pose unacceptable risks to human rights, health, and safety (e.g., systems used for social scoring or real-time remote biometric identification.
- High-Risk AI Systems: AI systems that can significantly impact human rights, health, and safety (e.g., systems used in critical infrastructure, employment, education, and law enforcement).
- Limited Risk AI Systems: AI systems that must adhere to specific transparency obligations, such as human-AI interaction disclosures (e.g., chatbots, facial recognition systems).
- Minimal/No Risk AI Systems: AI systems that do not inspire any tangible risks or impacts on human rights, health, and safety. Systems that fall into this category and are unregulated, though adherence to voluntary codes of conduct is encouraged.
AI systems classified as high-risk by the EU AI Act must comply with strict provisions. These include requirements for implementing risk management systems, robust data governance and management practices, and detailed technical documentation. Providers must also ensure comprehensive record-keeping (logging), adherence to transparency and explainability standards, and appropriate human oversight. Furthermore, systems in this category must meet high accuracy, robustness, and cybersecurity thresholds, undergo conformity assessments, and maintain quality management systems and post-market monitoring practices.
The EU AI Act has an extraterritorial scope, covering not only EU-based providers and users, but also non-EU providers whose systems are used within the EU. Those who violate the act’s provisions will be subject to substantial fines. However, fines issued depend on the severity of the infringement, and are capped at €35 million or 7% of a company’s annual turnover, whichever amount is higher – for prohibited AI practices.
Comparing the Frameworks: NIST vs. EU AI Act
Comparing the NIST AI Risk Management Framework and the EU AI Act reveals both shared goals and meaningful differences. On common ground, both frameworks aim to promote trustworthy and responsible AI (RAI), placing a strong emphasis on risk assessment and mitigation. They also mutually recognize the importance of core RAI principles like transparency, explainability, and fairness in AI systems.
However, their key differences are substantial. The NIST AI RMF is a high-level, voluntary, guidance-based framework without legal penalties and a broad but non-binding scope. By contrast, the EU AI Act is a binding regulation with a rules-based, prescriptive approach, particularly for high-risk AI, and is enforced through fines for non-compliance. While NIST broadly focuses on risk management processes, the EU AI Act mandates specific technical and process requirements, especially for systems categorized as high-risk and those operating within or impacting the EU. The applicability and primary relevance of the NIST AI RMF and the EU AI Act vary depending on an organization’s industry, location, and specific use of AI.
As a voluntary and highly flexible framework, the NIST AI RMF offers valuable guidance for managing AI risks and fostering trustworthiness across virtually all industries, particularly for organizations prioritizing RAI best practices and internal risk management standards, regardless of their geographic focus.
The EU AI Act has a significant impact on high-risk sectors, including:
- Healthcare: AI in medical devices, diagnostics, and patient risk assessment.
- Finance: Credit scoring, insurance risk assessment, and fraud detection.
- Transportation: Safety components in vehicles and traffic management systems.
- Employment & Worker Management: Recruitment, selection, and performance evaluation.
- Law Enforcement & Justice: Risk assessment, evidence evaluation, and judicial decision support systems.
- Critical Infrastructure & Utilities: Managing energy, water, transport, and digital networks where failure could cause serious harm.
Therefore, organizations must consider their specific AI use cases, their industry’s regulatory landscape, and their geographic markets to determine which framework(s) are most critical for their AI governance strategy and ensure necessary compliance or best practices are adopted.
Internal AI Governance Approaches
The concept of internal AI governance encompasses the policies, procedures, structures, and responsibilities that an individual organization implements to govern its development, deployment, and use of AI. Internal approaches may include grassroots RAI principles and risk management practices that can fill the gaps between framework-based AI governance and the reality of organizationally-specific use cases that reveal unforeseen AI risks, emerging at various stages throughout the AI lifecycle.
Stemming from the well-known practice of data governance, internal AI governance is an essential layer of proactive risk management. Organizations need their internal framework, even when external frameworks exist, to tailor approaches to specific business needs and AI use cases, embed ethical principles and values, ensure compliance with regulations, manage unique operational risks, and foster an RAI culture.
Effective internal AI governance involves several key components that organizations should consider:
- Establishing an AI ethics committee or review board.
- Developing internal AI use policies and guidelines for employees.
- Implementing robust data governance practices for AI development and deployment.
- Defining clear roles and responsibilities for AI oversight.
- Providing relevant training and education.
- Implementing internal audit and monitoring mechanisms.
- Integrating AI governance into existing risk management and compliance processes.
Internal AI governance efforts should align with the specific requirements and standards set by frameworks like the NIST AI RMF and EU AI Act. Essentially, internal governance translates external guidelines into practical, actionable policies and processes tailored to the organization’s unique context and AI initiatives.
Building a Comprehensive AI Governance Strategy: Integration is Key
To build truly effective AI governance, organizations must recognize that the most impactful approach involves seamlessly integrating external frameworks with tailored internal practices, in order to create a cohesive, proactive strategy.
Building this robust AI governance strategy follows several key steps. It begins by assessing the organization’s current AI landscape and identifying specific use cases; here, established governance frameworks can help validate or inform these scenarios. Next, determine which external regulations and frameworks apply, and conduct a comprehensive AI risk assessment. With this foundation, define your organization’s core AI principles and values, then develop and implement internal policies, procedures, and guidelines, establishing the necessary governance structures and roles for oversight. Providing training to build internal expertise and implementing ongoing monitoring, auditing, and review processes are crucial for continuous improvement, ultimately fostering an RAI culture throughout the organization.
Main Takeaways
In summary, navigating the AI landscape effectively requires understanding distinct governance approaches: the flexible, voluntary guidance of the NIST AI RMF, the legally binding requirements of the EU AI Act, and the essential role of tailored internal organizational frameworks.
Ultimately, proactive AI governance is paramount, extending beyond mere compliance to become fundamental for building trust, mitigating risks, and enabling responsible innovation.
At Lumenova AI, we encourage organizations to prioritize strengthening their AI governance to harness AI’s potential confidently. Moreover, we use our robust AI governance platform to help you design and implement the best compliance strategy for your organization. Request a demo today, and let’s discuss how we can help meet your unique needs and AI goals for maximizing business success in the age of AI.